accumulator的python怎么实现
时间: 2024-05-01 11:16:04 浏览: 18
在 Python 中实现累加器(accumulator)可以通过定义一个变量来存储累加的结果,然后在循环中不断更新它的值。以下是一个简单的累加器实现示例:
```python
# 初始化累加器
total = 0
# 循环累加
for i in range(1, 11):
total += i
# 输出结果
print("累加结果为:", total)
```
在上面的代码中,我们首先初始化了一个变量 `total` 作为累加器,并将其初始值设为 0。然后使用 for 循环对数字 1 到 10 进行遍历,并在每次循环中将当前数字加到累加器中。最后输出累加器的值,即得到了 1 到 10 的累加结果。
需要注意的是,累加器的实现方式可以根据具体的需求进行修改,例如可以在循环中根据条件进行累加,或者使用递归函数等方式实现。
相关问题
python实现hough变换
Hough变换是一种图像处理算法,用于在图像中检测直线、圆等几何形状。下面是Python实现Hough变换的代码:
首先,我们需要导入必要的库:
```python
import numpy as np
import cv2
import math
import matplotlib.pyplot as plt
```
接下来,我们读取一张图片,并将其转换为灰度图:
```python
img = cv2.imread('image.jpg')
gray = cv2.cvtColor(img,cv2.COLOR_BGR2GRAY)
```
然后,我们对灰度图进行边缘检测,这里使用Canny边缘检测算法:
```python
edges = cv2.Canny(gray,50,150,apertureSize = 3)
```
接下来,我们定义Hough变换的函数:
```python
def hough_transform(img, angle_step=1, lines_are_white=True, value_threshold=5):
"""
Hough transform for lines
Input:
img - 2D binary image with nonzeros representing edges
angle_step - Spacing between angles to use every n-th angle
between -90 and 90 degrees. Default step is 1.
lines_are_white - boolean indicating whether lines to be detected are white
value_threshold - Pixel values above or below the value_threshold are edges
Returns:
accumulator - 2D array of the hough transform accumulator
theta - array of angles used in computation, in radians.
rhos - array of rho values. Max size is 2 times the diagonal
distance of the input image.
"""
# Rho and Theta ranges
thetas = np.deg2rad(np.arange(-90.0, 90.0, angle_step))
width, height = img.shape
diag_len = int(round(math.sqrt(width * width + height * height)))
rhos = np.linspace(-diag_len, diag_len, diag_len * 2.0)
# Cache some resuable values
cos_t = np.cos(thetas)
sin_t = np.sin(thetas)
num_thetas = len(thetas)
# Hough accumulator array of theta vs rho
accumulator = np.zeros((2 * diag_len, num_thetas), dtype=np.uint8)
yc, xc = np.nonzero(img)
# Vote in the hough accumulator
for i in range(len(xc)):
x = xc[i]
y = yc[i]
for t_idx in range(num_thetas):
# Calculate rho. diag_len is added for a positive index
rho = int(round(x * cos_t[t_idx] + y * sin_t[t_idx]) + diag_len)
accumulator[rho, t_idx] += 1
return accumulator, thetas, rhos
```
最后,我们调用Hough变换函数,并将结果可视化:
```python
accumulator, thetas, rhos = hough_transform(edges)
# Show the accumulator array
plt.imshow(accumulator, cmap='jet', extent=[np.rad2deg(thetas[-1]), np.rad2deg(thetas[0]), rhos[-1], rhos[0]])
plt.title('Hough transform accumulator')
plt.xlabel('Angles (degrees)')
plt.ylabel('Distance (pixels)')
plt.show()
```
这样,我们就完成了Hough变换的实现。
用Python实现具体的hough变换
以下是一个Python实现的Hough变换的示例代码。这里的实现是用来检测图像中的直线。
```python
import numpy as np
import cv2
def hough_lines(img, rho, theta, threshold):
"""
返回一组检测到的直线
"""
# 初始化一个空的投票矩阵
height, width = img.shape
diag_len = int(np.ceil(np.sqrt(height**2 + width**2))) # 对角线长度
accumulator = np.zeros((2 * diag_len, 180), dtype=np.uint8)
# 取得图像中所有非零像素的坐标
coords = np.column_stack(np.where(img > 0))
# 对于每个非零像素,计算它们在Hough空间中的所有可能的直线
for x, y in coords:
for t in range(0, 180):
r = int((x * np.cos(np.deg2rad(t))) + (y * np.sin(np.deg2rad(t))))
accumulator[r + diag_len, t] += 1
# 返回所有投票数超过阈值的直线
lines = []
for r, theta in np.argwhere(accumulator >= threshold):
lines.append((r - diag_len, theta))
return lines
# 加载图像并将其转换为灰度
image = cv2.imread('image.jpg')
gray = cv2.cvtColor(image, cv2.COLOR_BGR2GRAY)
# 对灰度图像应用Canny边缘检测器
edges = cv2.Canny(gray, 50, 150, apertureSize=3)
# 运行Hough变换检测直线
lines = hough_lines(edges, 1, np.pi / 180, 200)
# 将检测到的直线绘制到原始图像上
for r, theta in lines:
a = np.cos(theta)
b = np.sin(theta)
x0 = a * r
y0 = b * r
x1 = int(x0 + 1000 * (-b))
y1 = int(y0 + 1000 * (a))
x2 = int(x0 - 1000 * (-b))
y2 = int(y0 - 1000 * (a))
cv2.line(image, (x1, y1), (x2, y2), (0, 0, 255), 2)
# 显示结果
cv2.imshow('Image', image)
cv2.waitKey(0)
cv2.destroyAllWindows()
```
这个示例代码加载了一个名为`image.jpg`的图像,并将其转换为灰度图像。然后,它使用Canny边缘检测器检测边缘,并通过调用`hough_lines`函数运行Hough变换来检测直线。最后,它将检测到的直线绘制到原始图像上,并显示结果。
相关推荐
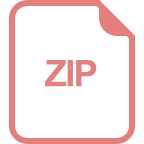
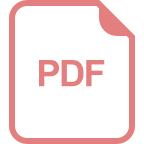












