python bert模型实体抽取
时间: 2023-07-05 11:32:34 浏览: 47
以下是使用BERT模型进行实体抽取的Python代码示例:
```python
import torch
from transformers import BertTokenizer, BertForTokenClassification
# 加载BERT模型和tokenizer
tokenizer = BertTokenizer.from_pretrained('bert-base-uncased')
model = BertForTokenClassification.from_pretrained('bert-base-uncased', num_labels=3)
# 输入文本
text = "Tom works at Google in California."
# 对文本进行预处理并转换为模型输入
inputs = tokenizer(text, return_tensors='pt')
outputs = model(**inputs)
# 获取标签预测结果
predictions = outputs.logits.argmax(-1)[0].tolist()
print(predictions)
# 解码标签序列
decoded_predictions = [model.config.id2label[prediction] for prediction in predictions]
print(decoded_predictions)
# 提取实体
entities = []
current_entity = None
for token, prediction in zip(tokenizer.tokenize(text), decoded_predictions):
if prediction == "B-PER" or prediction == "B-ORG" or prediction == "B-LOC":
if current_entity is not None:
entities.append(current_entity)
current_entity = {"entity_type": prediction.split("-")[1], "text": token}
elif prediction == "I-PER" or prediction == "I-ORG" or prediction == "I-LOC":
current_entity["text"] += token
else:
if current_entity is not None:
entities.append(current_entity)
current_entity = None
print(entities)
```
在上述代码中,我们首先导入了`torch`和`transformers`库,然后加载了BERT模型和tokenizer。接着,我们定义了一个输入文本并使用tokenizer对其进行预处理。最后,我们将预处理后的输入传递给BERT模型,并使用`argmax()`方法获取了模型的预测结果。
在获取标签预测结果后,我们使用模型配置中的`id2label`映射将其解码为实际的标签序列。然后,我们遍历标记化的文本和解码的标签序列,提取实体并将其存储在一个列表中。
请注意,这里的BERT模型用于标记分类任务,因此我们将其`num_labels`设置为3,其中包括“O”(表示无标签)、“B-<entity_type>”(表示起始实体标签)和“I-<entity_type>”(表示中间实体标签)。如果您的任务需要提取不同类型的实体,请相应地设置`num_labels`和提取实体的逻辑。
相关推荐
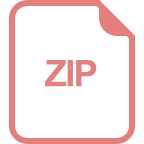
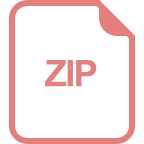
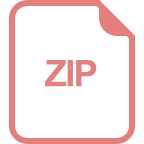














