利用Matplotlib包进行数据可视化。调用Matplotlib包绘制数据框df的petal_length与petal_width的散点图,图片显示方式为静态绘图。设置图的名称为“花瓣散点图”,x轴的名称为“花瓣长度(厘米)”,y轴的名称为“花瓣宽度(厘米)
时间: 2024-02-05 08:11:55 浏览: 81
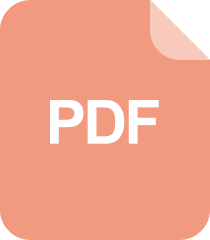
用Seaborn轻松的进行数据可视化
下面是代码实现:
```python
import matplotlib.pyplot as plt
# 绘制散点图
plt.scatter(df['petal_length'], df['petal_width'])
# 设置图的标题和坐标轴标签
plt.title('花瓣散点图')
plt.xlabel('花瓣长度(厘米)')
plt.ylabel('花瓣宽度(厘米)')
# 显示图像
plt.show()
```
其中,`df`是数据框的名称。如果没有导入数据框,可以使用以下代码:
```python
import seaborn as sns
iris = sns.load_dataset('iris')
df = iris[['petal_length', 'petal_width']]
```
这段代码会从seaborn库中加载鸢尾花数据集,并将数据框`df`定义为包含两列数据:`petal_length`和`petal_width`。
阅读全文
相关推荐
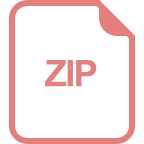
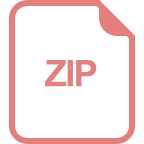















