如何使用Python进行语义分析以识别文本中的当事人关系?
时间: 2024-10-22 09:18:44 浏览: 48
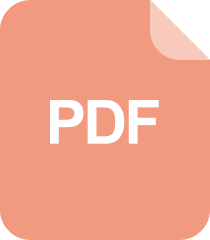
在会计研究中使用 Python 进行文本分析-研究论文
在Python中进行语义分析以识别文本中的当事人关系通常涉及到自然语言处理(NLP)技术和实体关系抽取(NER)。以下是一个简化的步骤:
1. **安装库**:首先需要安装一些关键的NLP库,如NLTK、spaCy、Stanford CoreNLP或Hugging Face的Transformers。
```python
!pip install nltk spacy stanfordnlp transformers
```
2. **数据预处理**:对文本进行清洗和分词,去除无关字符,并将文本转换成模型可以理解的格式。
```python
import nltk
from nltk.tokenize import word_tokenize
def preprocess_text(text):
text = nltk.sent_tokenize(text)
words = [word_tokenize(sent) for sent in text]
return words
```
3. **加载模型**:对于stanfordnlp或transformers,你需要下载预先训练好的模型。例如,使用BERT进行命名实体识别(NER):
```python
import stanza
nlp = stanza.Pipeline('zh', processors='tokenize,ner')
# 或者使用Hugging Face的BERT NER:
from transformers import pipeline
ner_pipeline = pipeline("ner", model="dbmdz/bert-large-cased-finetuned-conll03-english")
```
4. **提取实体及关系**:通过模型的`ents`属性获取每个句子中的实体及其类型,然后根据实体之间的关系规则找出当事人关系。这可能涉及词性标注、依存句法分析等。
```python
def extract_relations(words, nlp_model):
doc = nlp_model(words)
entities = [(ent.text, ent.type_) for sent in doc.sentences for ent in sent.ents]
# 简单示例,实际关系提取可能更复杂,依赖于领域知识或特定算法
relations = []
for i, entity_i in enumerate(entities):
for j, entity_j in enumerate(entities[i+1:], start=1):
if '人名' in (entity_i[1], entity_j[1]):
relations.append((entity_i[0], entity_j[0]))
return relations
```
5. **分析结果**:返回识别出的当事人关系列表。
注意,这个过程可能会非常复杂,因为语义分析涉及到深度学习模型和复杂的语言规则。实际应用中,可能需要结合领域知识和特定算法才能提高准确性和效率。
阅读全文