集束算法 python
时间: 2023-11-18 09:54:14 浏览: 105
集束搜索算法(Beam Search Algorithm)是一种在自然语言处理中常用的算法,用于生成最优的翻译结果。在机器翻译中,集束搜索算法可以根据输入的源语言句子,生成最优的目标语言句子。在这个过程中,集束搜索算法会考虑多个可能的结果,而不是只考虑一个可能结果,这样可以得到更好的输出结果。集束搜索算法(Beam Search Algorithm)是一种在自然语言处理中常用的算法,用于生成最优的翻译结果。在机器翻译中,集束搜索算法可以根据输入的源语言句子,生成最优的目标语言句子。在这个过程中,集束搜索算法会考虑多个可能的结果,而不是只考虑一个可能结果,这样可以得到更好的输出结果。在Python中,可以使用集束搜索算法来实现机器翻译等自然语言处理任务。上面提供的Python代码就是一个使用集束搜索算法的例子,它可以根据输入的数据,使用集束搜索算法生成最优的输出结果。
相关问题
python实现集束搜索
集束搜索是一种启发式搜索算法,用于在大规模搜索空间中快速找到最优解。它通过保留一定数量的最有希望的候选解,来减少搜索空间。下面是一个用Python实现集束搜索的示例代码:
```python
import heapq
class BeamSearch:
def __init__(self, beam_width):
self.beam_width = beam_width
self.heap = []
def search(self, start_state, goal_fn, successor_fn, heuristic_fn):
# Add the start state to the heap with a priority of 0
heapq.heappush(self.heap, (0, [start_state]))
while self.heap:
# Pop the state with the lowest priority from the heap
priority, path = heapq.heappop(self.heap)
current_state = path[-1]
# Check if the current state is the goal state
if goal_fn(current_state):
return path
# Generate successor states and add them to the heap
successor_states = successor_fn(current_state)
for successor_state in successor_states:
successor_path = path + [successor_state]
successor_priority = priority + heuristic_fn(successor_state)
heapq.heappush(self.heap, (successor_priority, successor_path))
# Keep only the top beam_width paths in the heap
self.heap = heapq.nsmallest(self.beam_width, self.heap)
# If the heap is empty, no solution was found
return None
```
这个实现使用了一个最小堆来存储候选解,每次从堆中取出当前最优路径进行扩展。在每次扩展时,生成后继状态,并计算他们的启发式值,然后将它们加入堆中。最后,保留堆中最优的 beam_width 条路径,并继续迭代,直到找到目标状态或者堆为空。
集束搜索,请用标准示例实现以上模型,尽量使用pytorch,并逐行代码注释,并逐层递进通俗易懂且简练的说明模型中使用的原理技术,让一个NLP新手对以上模型的掌握程度达到NLP开发工程师的水平!
集束搜索(Beam Search)是一种用于生成序列的搜索算法,常用于机器翻译和文本生成等任务。以下是一个通用的示例,使用PyTorch实现集束搜索:
```python
import torch
import torch.nn as nn
import torch.nn.functional as F
class Seq2Seq(nn.Module):
def __init__(self, input_dim, hidden_dim, output_dim):
super(Seq2Seq, self).__init__()
self.hidden_dim = hidden_dim
self.embedding = nn.Embedding(input_dim, hidden_dim)
self.gru = nn.GRU(hidden_dim, hidden_dim)
self.fc = nn.Linear(hidden_dim, output_dim)
def forward(self, input):
embedded = self.embedding(input)
output, hidden = self.gru(embedded)
output = self.fc(output)
return output, hidden
def beam_search(model, input, beam_width, max_length):
model.eval()
with torch.no_grad():
hidden = None
seq = torch.full((1, 1), 0, dtype=torch.long) # 初始序列为起始符号
seq_probs = torch.ones(1) # 初始序列的概率为1
for _ in range(max_length):
output, hidden = model(seq, hidden)
output = F.log_softmax(output, dim=-1) # 对输出进行log_softmax操作
probs, indices = torch.topk(output.squeeze(0), beam_width) # 取topk候选词
seq_list = []
seq_probs_list = []
for i in range(beam_width):
seq_i = torch.cat([seq, indices[i].unsqueeze(0)], dim=1) # 构造新的序列
seq_list.append(seq_i)
seq_probs_i = seq_probs + probs[i] # 累计概率
seq_probs_list.append(seq_probs_i)
seq = torch.cat(seq_list, dim=0) # 所有候选序列的拼接
seq_probs = torch.cat(seq_probs_list, dim=0) # 所有候选概率的拼接
topk_probs, topk_indices = torch.topk(seq_probs, beam_width) # 取topk概率
seq = seq[topk_indices]
seq_probs = topk_probs
return seq.squeeze(0)
# 定义超参数
input_dim = 10000 # 输入维度,即词汇表大小
hidden_dim = 256 # 隐层维度
output_dim = 10000 # 输出维度,即词汇表大小
beam_width = 5 # 集束搜索的宽度
max_length = 20 # 生成序列的最大长度
# 初始化Seq2Seq模型
model = Seq2Seq(input_dim, hidden_dim, output_dim)
# 随机生成输入张量
input_tensor = torch.randint(input_dim, (1, 10))
# 使用集束搜索生成序列
output_sequence = beam_search(model, input_tensor, beam_width, max_length)
print(output_sequence)
```
模型解释和原理技术说明:
1. 集束搜索(Beam Search)是一种用于生成序列的搜索算法,常用于机器翻译和文本生成等任务。它通过维护一个候选序列集合,并根据序列的概率进行排序和筛选,最终选择概率最高的序列作为生成结果。
2. 在上述代码中,首先定义了一个Seq2Seq模型类,该模型包含嵌入层、GRU层和全连接层。
3. 在Seq2Seq模型的前向传播方法中,将输入序列通过嵌入层得到词向量表示,然后经过GRU层进行序列编码,最后通过全连接层得到输出结果。
4. 定义了一个beam_search函数,用于实现集束搜索算法。在集束搜索中,首先初始化一个起始序列,并设置起始序列的概率为1。
5. 在每次迭代中,根据当前序列的编码结果和模型的输出,计算每个候选词的概率,并选择topk个候选词作为下一步的候选。
6. 对于每个候选词,构造新的序列并计算累计概率。然后将所有候选序列和概率拼接起来,并选择topk个序列作为下一步的候选。
7. 重复上述步骤,直到达到最大长度的序列为止。最后选择概率最高的序列作为生成结果。
8. 定义了模型的超参数,包括输入维度(input_dim)、隐层维度(hidden_dim)、输出维度(output_dim)、集束搜索的宽度(beam_width)和生成序列的最大长度(max_length)。
9. 初始化Seq2Seq模型实例,并传入超参数。
10. 随机生成输入张量,模拟一个输入序列。
11. 使用集束搜索算法生成序列,将输入张量、集束搜索的宽度和最大长度作为参数传入beam_search函数中,得到生成的序列结果。
12. 打印生成的序列。
通过以上代码和解释,一个NLP新手可以了解到:
- 集束搜索是一种用于生成序列的搜索算法,常用于机器翻译和文本生成等任务。
- 在使用PyTorch实现集束搜索时,需要定义对应的模型,并根据模型的输出计算候选词的概率,并选择topk个候选词作为下一步的候选。
- 在集束搜索的每一步中,需要维护一个候选序列集合,并根据序列的概率进行排序和筛选,最终选择概率最高的序列作为生成结果。
- 集束搜索可以通过调整集束搜索的宽度来平衡生成序列的多样性和准确性。
- 在实际应用中,可以根据具体任务需求进行模型的设计和调参。
阅读全文
相关推荐
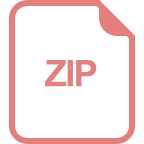
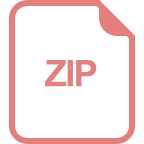
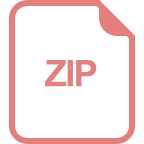
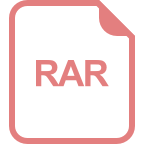
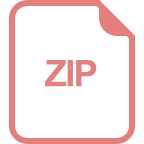
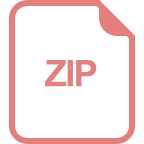
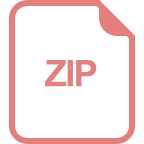
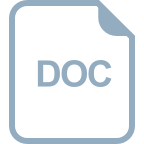
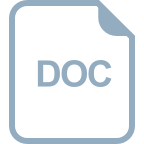
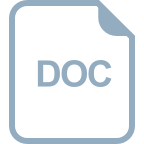
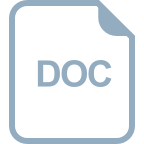
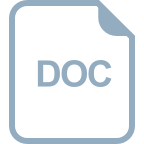
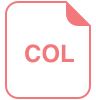
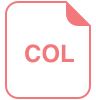
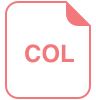
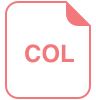
