粒子群算法求解最优解的python代码
时间: 2024-06-17 15:01:11 浏览: 195
粒子群算法(Particle Swarm Optimization, PSO)是一种模拟鸟群或鱼群寻找食物行为的优化算法,用于寻找函数的全局最优解。在Python中,有许多库可以帮助你实现PSO,如`psoptim`、`deap`等。下面是一个简单的粒子群优化求解一维函数最小值的示例代码:
```python
# 导入必要的库
import numpy as np
from psoptim import PSO
# 定义一个简单的函数(例如求f(x) = x^2)
def objective_function(x):
return x**2
# 创建粒子群参数
n_particles = 50 # 粒子数量
n_dimensions = 1 # 搜索维度
max_iterations = 100 # 迭代次数
velocity_bound = 2 # 速度范围
position_bound = 10 # 位置范围
# 初始化粒子群
pso = PSO(n_particles, n_dimensions, objective_function,
velocity_bound=velocity_bound, position_bound=position_bound)
# 运行粒子群优化
solution, best_fitness = pso.run(max_iterations)
# 输出最优解和最佳适应度
print(f"最优解: {solution}, 最佳适应度: {best_fitness}")
```
相关问题
粒子群算法求解车间调度问题python代码
粒子群算法(Particle Swarm Optimization,PSO)是一种具有全局寻优能力的优化方法,可以应用于车间调度问题。下面是用Python实现车间调度问题的粒子群算法。
首先,定义函数以计算每个粒子的适应度,即车间调度的总加工时间:
```
def fitness_func(schedule, jobs):
times = [0] * len(jobs)
for i in range(len(schedule)):
job = jobs[schedule[i]]
if i == 0:
times[i] = job[0] + job[1]
else:
times[i] = max(times[i-1], job[0]) + job[1]
return max(times)
```
然后,实现粒子群算法:
```
# 初始化粒子
def init_particles(num_p, num_j):
particles = []
for i in range(num_p):
particle = []
for j in range(num_j):
particle.append(random.randint(0, num_j-1))
particles.append(particle)
return particles
# 计算每个粒子的适应度
def update_fitness(particles, jobs):
fitness = []
for particle in particles:
fitness.append(fitness_func(particle, jobs))
return fitness
# 更新每个粒子的速度和位置
def update_particles(particles, best, w, c1, c2):
for i in range(len(particles)):
for j in range(len(particles[i])):
r1 = random.uniform(0, 1)
r2 = random.uniform(0, 1)
particles[i][j] = int(particles[i][j] + w * (best[i][j] - particles[i][j]) + c1 * r1 * (global_best[j] - particles[i][j]) + c2 * r2 * (best_global[j] - particles[i][j]))
if particles[i][j] < 0:
particles[i][j] = 0
elif particles[i][j] > len(jobs)-1:
particles[i][j] = len(jobs)-1
# 计算全局最优解和每个粒子的最优解
def update_best(particles, best):
for i in range(len(particles)):
if fitness[i] < best[i][len(jobs)]:
best[i] = particles[i] + [fitness[i]]
if fitness[i] < best_global[len(jobs)]:
best_global = particles[i] + [fitness[i]]
```
最后,运行粒子群算法的主函数:
```
if __name__ == '__main__':
jobs = [(4, 5), (1, 3), (2, 4), (4, 2), (1, 5), (4, 2), (3, 5), (2, 1), (5, 2), (4, 4)]
num_particles = 50
num_generations = 100
w = 0.9
c1 = 2
c2 = 2
particles = init_particles(num_particles, len(jobs))
fitness = update_fitness(particles, jobs)
best, best_global = [], particles[0] + [fitness[0]]
for i in range(len(particles)):
best.append(particles[i] + [fitness[i]])
for i in range(num_generations):
update_particles(particles, best, w, c1, c2)
fitness = update_fitness(particles, jobs)
update_best(particles, best)
print('Generation: {} Best Fitness: {}'.format(i, best_global[len(jobs)]))
print('Best Schedule: {}'.format(best_global[:-1]))
```
在以上代码中,我们使用随机生成的作业数据完成车间调度问题的求解。输出结果包括每一代的最佳适应度和最终的最佳排程方案。随着迭代次数的增加,算法得到的最佳适应度越来越接近全局最优解,最终得到的排程方案也更加合理。
粒子群算法求解01背包问题python代码
下面是使用粒子群算法求解01背包问题的Python代码:
```python
import random
# 01背包问题
class KnapsackProblem:
def __init__(self, n, c, w, v):
self.n = n # 物品数量
self.c = c # 背包容量
self.w = w # 物品重量
self.v = v # 物品价值
# 计算个体的适应度
def fitness(self, x):
weight = sum([x[i] * self.w[i] for i in range(self.n)]) # 计算重量
if weight > self.c: # 如果超过了背包容量,则适应度为0
return 0
else: # 否则适应度为物品的总价值
return sum([x[i] * self.v[i] for i in range(self.n)])
# 粒子群算法
class PSO:
def __init__(self, problem, pop_size, max_iter, c1, c2, w):
self.problem = problem # 问题实例
self.pop_size = pop_size # 粒子群大小
self.max_iter = max_iter # 最大迭代次数
self.c1 = c1 # 学习因子1
self.c2 = c2 # 学习因子2
self.w = w # 惯性因子
self.gbest = None # 全局最优解
self.particles = [] # 所有粒子
self.init_particles() # 初始化所有粒子
# 初始化一个粒子
def init_particle(self):
x = [random.randint(0, 1) for i in range(self.problem.n)] # 随机生成一个个体
p = Particle(x) # 创建一个粒子对象
p.fitness = self.problem.fitness(p.x) # 计算个体的适应度
p.pbest = p.x[:] # 初始化个体最优解
p.pbest_fitness = p.fitness # 初始化个体最优解的适应度
return p
# 初始化所有粒子
def init_particles(self):
self.particles = [self.init_particle() for i in range(self.pop_size)]
self.gbest = max(self.particles, key=lambda p: p.fitness) # 初始化全局最优解
# 更新粒子的速度和位置
def update_particle(self, p):
r1, r2 = random.random(), random.random() # 生成两个随机数
for i in range(self.problem.n):
p.v[i] = self.w * p.v[i] + self.c1 * r1 * (p.pbest[i] - p.x[i]) + self.c2 * r2 * (self.gbest.x[i] - p.x[i])
if p.v[i] > 1: # 速度限制在[-1, 1]范围内
p.v[i] = 1
elif p.v[i] < -1:
p.v[i] = -1
p.x[i] = 1 if random.random() < sigmoid(p.v[i]) else 0 # 更新位置
p.fitness = self.problem.fitness(p.x) # 计算适应度
if p.fitness > p.pbest_fitness: # 更新个体最优解
p.pbest = p.x[:]
p.pbest_fitness = p.fitness
# 迭代粒子群
def iterate(self):
for i in range(self.max_iter):
for p in self.particles:
self.update_particle(p)
if p.fitness > self.gbest.fitness: # 更新全局最优解
self.gbest = p
# 输出结果
def output(self):
print("最优解:", self.gbest.x)
print("最优解的适应度:", self.gbest.fitness)
# 粒子类
class Particle:
def __init__(self, x):
self.x = x # 粒子的位置(即个体)
self.v = [random.uniform(-1, 1) for i in range(len(x))] # 粒子的速度
self.fitness = 0 # 适应度(用于评价个体好坏)
self.pbest = x[:] # 个体最优解
self.pbest_fitness = 0 # 个体最优解的适应度
# sigmoid函数
def sigmoid(x):
return 1 / (1 + math.exp(-x))
# 测试
if __name__ == '__main__':
n = 10 # 物品数量
c = 50 # 背包容量
w = [random.randint(1, 10) for i in range(n)] # 物品重量
v = [random.randint(1, 10) for i in range(n)] # 物品价值
problem = KnapsackProblem(n, c, w, v)
pso = PSO(problem, pop_size=50, max_iter=100, c1=2, c2=2, w=0.8)
pso.iterate()
pso.output()
```
代码中使用了sigmoid函数来把速度转换为位置,这样可以避免速度过大或过小导致的问题。代码还使用了粒子群算法的经典公式来更新粒子的速度和位置。最后,我们可以通过运行代码来测试它的效果。
阅读全文
相关推荐
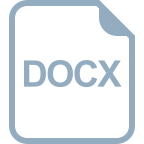
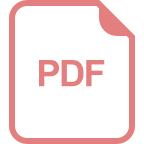
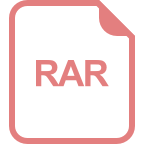


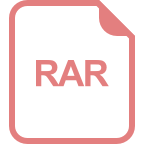
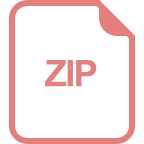
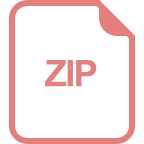
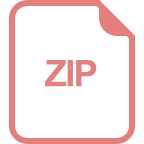
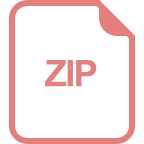
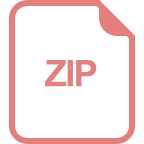
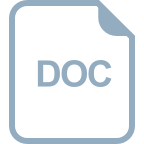



