df = pd.read_csv( /train.csv , sep= )
时间: 2023-12-09 17:34:58 浏览: 109
很抱歉,引用中提供的代码片段中没有给出`/train.csv`的具体路径,无法确定`pd.read_csv()`函数中的文件路径参数。但是,根据代码片段中的其他信息,我们可以了解到以下内容:
1. `pd.read_csv()`函数用于读取CSV文件并将其转换为DataFrame格式。
2. `sep`参数用于指定CSV文件中的分隔符。
3. 引用中的代码片段使用`pd.read_csv()`函数从`adult.data`文件中读取数据,并将其保存为`adults.csv`文件。
4. 引用中的代码片段使用`pd.read_csv()`函数从`train`文件中读取数据,并将其转换为DataFrame格式。
因此,如果您想使用`pd.read_csv()`函数读取CSV文件并将其转换为DataFrame格式,您需要提供CSV文件的路径和文件名,并使用`sep`参数指定分隔符。例如,如果您的CSV文件名为`train.csv`,并且该文件位于当前工作目录中,则可以使用以下代码:
```python
import pandas as pd
df = pd.read_csv('train.csv', sep=',')
```
请注意,上述代码假定CSV文件中使用逗号作为分隔符。如果您的CSV文件使用其他分隔符,请相应地更改`sep`参数的值。
相关问题
根据以下训练好的模型,预测待预测样本(test_price.csv)中车身类型(bodyType字段)为“微型车”的price,将预测的price数据保存在submit.csv文件。import pandas as pd import numpy as np from sklearn.model_selection import train_test_split from sklearn.metrics import r2_score from sklearn.ensemble import RandomForestRegressor from sklearn.metrics import mean_absolute_error train_data = pd.read_csv('train_price.csv',sep=" ") test_data = pd.read_csv('test_price.csv',sep=" ") microcar_data = train_data[train_data['bodyType'] == 1.0] microcar1_data = test_data[test_data['bodyType'] == 1.0] # # 1、“微型车”待预测样本的df.head()和df.shape # print(microcar1_data.head()) # microcar1_data.shape # 2、模型训练,及模型评价 features = ['v_1','v_2','v_3','v_4'] # 自由选择特征列 target = 'price' X_train, X_test, y_train, y_test = train_test_split(microcar_data[features], microcar_data[target], test_size=0.2, random_state=int('0713')) model = RandomForestRegressor() model.fit(X_train, y_train) y_pred = model.predict(X_test)
根据以上代码,你需要对待预测样本中车身类型为“微型车”的price进行预测,并将预测结果保存在submit.csv文件中。你可以使用以下代码来进行预测和保存结果:
# 3、预测待预测样本中车身类型为“微型车”的price
microcar1_data['price'] = model.predict(microcar1_data[features])
# 4、保存预测结果
microcar1_data[['SaleID', 'price']].to_csv('submit.csv', index=False)
需要注意的是,以上代码只针对车身类型为“微型车”的待预测样本进行预测和保存结果。如果待预测样本中还有其他车身类型的样本需要进行预测,则需要对相应的样本进行处理并进行预测。
import numpy as np import pandas as pd import matplotlib.pyplot as plt df=pd.read_csv('C:\\Users\ASUS\Desktop\AI\实训\汽车销量数据new.csv',sep=',',header=0) plt.rcParams['font.sans-serif'] = ['SimHei'] plt.figure(figsize=(10,4)) ax1=plt.subplot(121) ax1.scatter(df['price'],df['quantity'],c='b') df=(df-df.min())/(df.max()-df.min()) df.to_csv('quantity.txt',sep='\t',index=False) train_data=df.sample(frac=0.8,replace=False) test_data=df.drop(train_data.index) x_train=train_data['price'].values.reshape(-1, 1) y_train=train_data['quantity'].values x_test=test_data['price'].values.reshape(-1, 1) y_test=test_data['quantity'].values from sklearn.linear_model import LinearRegression import joblib #model=SGDRegressor(max_iter=500,learning_rate='constant',eta0=0.01) model = LinearRegression() #训练模型 model.fit(x_train,y_train) #输出训练结果 pre_score=model.score(x_train,y_train) print('训练集准确性得分=',pre_score) print('coef=',model.coef_,'intercept=',model.intercept_) #保存训练后的模型 joblib.dump(model,'LinearRegression.model') ax2=plt.subplot(122) ax2.scatter(x_train,y_train,label='测试集') ax2.plot(x_train,model.predict(x_train),color='blue') ax2.set_xlabel('工龄') ax2.set_ylabel('工资') plt.legend(loc='upper left') model=joblib.load('LinearRegression.model') y_pred=model.predict(x_test)#得到预测值 print('测试集准确性得分=%.5f'%model.score(x_test,y_test)) #计算测试集的损失(用均方差) MSE=np.mean((y_test - y_pred)**2) print('损失MSE={:.5f}'.format(MSE)) plt.rcParams['font.sans-serif'] = ['SimHei'] plt.figure(figsize=(10,4)) ax1=plt.subplot(121) plt.scatter(x_test,y_test,label='测试集') plt.plot(x_test,y_pred,'r',label='预测回归线') ax1.set_xlabel('工龄') ax1.set_ylabel('工资') plt.legend(loc='upper left') ax2=plt.subplot(122) x=range(0,len(y_test)) plt.plot(x,y_test,'g',label='真实值') plt.plot(x,y_pred,'r',label='预测值') ax2.set_xlabel('样本序号') ax2.set_ylabel('工资') plt.legend(loc='upper right') plt.show()怎么预测价格为15万时的销量
要预测价格为15万时的销量,可以使用训练好的线性回归模型进行预测。首先需要将15万的价格转换为模型可接受的输入格式,即将其转换为一个形状为(1,1)的二维数组:
```python
price = np.array([[15]])
```
然后使用训练好的模型进行预测:
```python
quantity = model.predict(price)
print('价格为15万时的销量预测值为:', quantity)
```
该代码将输出一个预测值,即价格为15万时的销量预测值。
阅读全文
相关推荐





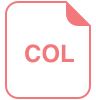








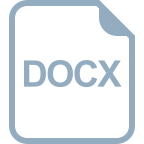