C4.5决策树随机森林代码实现
时间: 2024-09-29 14:01:00 浏览: 21
C4.5决策树是一种常用的机器学习算法,而随机森林(Random Forest)则是基于多个决策树集成的预测模型,它通过构建大量决策树并取其平均结果来提高模型的稳定性和准确性。以下是Python中使用sklearn库实现C4.5决策树和随机森林的一个简单示例:
首先,安装所需的库:
```bash
pip install scikit-learn pandas numpy
```
然后,你可以按照以下步骤创建和训练随机森林模型:
```python
# 导入必要的库
from sklearn import datasets
from sklearn.model_selection import train_test_split
from sklearn.tree import DecisionTreeClassifier
from sklearn.ensemble import RandomForestClassifier
import pandas as pd
# 加载数据集,例如鸢尾花数据集
iris = datasets.load_iris()
X = iris.data
y = iris.target
# 划分数据集为训练集和测试集
X_train, X_test, y_train, y_test = train_test_split(X, y, test_size=0.2, random_state=42)
# 创建单棵C4.5决策树
c45_tree = DecisionTreeClassifier(criterion='gini', max_depth=None)
c45_tree.fit(X_train, y_train)
# 使用sklearn的RandomForestClassifier创建随机森林
random_forest = RandomForestClassifier(n_estimators=100, criterion='gini') # n_estimators是树的数量
random_forest.fit(X_train, y_train)
# 预测测试集
c45_tree_predictions = c45_tree.predict(X_test)
random_forest_predictions = random_forest.predict(X_test)
# 打印性能指标,如准确率
print("C4.5 Decision Tree accuracy:", accuracy_score(y_test, c45_tree_predictions))
print("Random Forest accuracy:", accuracy_score(y_test, random_forest_predictions))
相关推荐
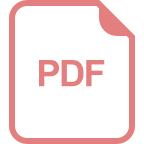
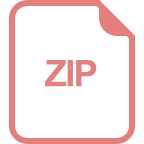
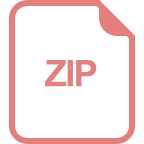














