/home/mating/untitled1/CMakeLists.txt:15: error: No SOURCES given to target: buff
时间: 2024-09-22 15:08:18 浏览: 46
在你的`/home/mating/untitled1/CMakeLists.txt`文件的第15行出现了错误:“No SOURCES given to target: buff”。这表示CMake没有找到目标“buff”的源代码文件列表。`buff`可能是你想编译的一个程序、库或者是其它类型的构建单元。
解决此问题你需要确保在`add_library`或`add_executable`这样的指令中,你为`buff`这个target提供了至少一个源文件。例如,如果是`add_library`,应该类似这样:
```cmake
add_library(buff src/buff.cpp)
```
如果是`add_executable`用于创建可执行程序:
```cmake
add_executable(buff main.cpp src/buff.cpp)
```
这里假设`src/buff.cpp`是你想要作为`buff` target的源文件之一。
如果你有多个源文件,你可以将它们放在数组中:
```cmake
add_executable(buff main.cpp src/file1.cpp src/file2.cpp src/buff.cpp)
```
相关问题
function offspring = crossover(mating_pool, crossover_rate,sparse_degree) population_size = size(mating_pool, 1);%获取行数即种群大小 offspring = zeros(population_size, size(mating_pool, 2));%初始化一个和配对的父母一样大小的矩阵,用来存放交叉后的新的后代 for i = 1:2:population_size-1 parent1 = mating_pool(i, :); parent2 = mating_pool(i+1, :); if rand() < crossover_rate % 根据交叉概率决定是否对当前的一对父母进行交叉操作 indices1 = find(parent1 == 1); indices2 = find(parent2 == 1);%找出每个父本中基因为 1 的位置,将这些位置存储在 indices1 和 indices2 中 num_to_swap = min([sparse_degree, length(indices1), length(indices2)]);% 确定要交换的基因数量,这个数量由稀疏度、父本1中基因为 1 的位置数量以及父本2中基因为 1 的位置数量中的最小值确定 if num_to_swap > 0 swap_indices1 = indices1(randperm(length(indices1), num_to_swap));%从每个父母中选择一些随机的位置用于交换 swap_indices2 = indices2(randperm(length(indices2), num_to_swap)); temp1 = parent1; temp2 = parent2; temp1(swap_indices1) = parent2(swap_indices2); temp2(swap_indices2) = parent1(swap_indices1); % Force sparsity temp1 = enforce_sparsity(temp1, sparse_degree); temp2 = enforce_sparsity(temp2, sparse_degree); offspring(i, :) = temp1;%经过交叉后的新的后代存入后代矩阵中 offspring(i+1, :) = temp2; else offspring(i, :) = parent1;%如果不进行交叉操作,那么子代就直接是父母 offspring(i+1, :) = parent2; end else offspring(i, :) = parent1; offspring(i+1, :) = parent2; end end end上面这个算法是什么交叉方式
这是一种基因型部分匹配交叉(Partially-Mapped Crossover, PMX)的交叉方式。在该方式中,首先随机选择两个父本,然后根据交叉概率决定是否对它们进行交叉操作。如果进行交叉操作,首先找出每个父本中基因为1的位置,将这些位置存储在indices1和indices2中。接着,确定要交换的基因数量,这个数量由稀疏度、父本1中基因为1的位置数量以及父本2中基因为1的位置数量中的最小值确定。然后从每个父母中选择一些随机的位置用于交换,将这些位置上的基因进行交叉操作。最后,根据稀疏度对交叉后的个体进行修剪,生成新的后代。如果不进行交叉操作,那么子代就直接是父母。
解释以下代码:import numpy as np import pandas as pd from scipy.optimize import minimize from pygad import GA 读取数据 stations = pd.read_excel("附件 1:车站数据.xlsx") section_time = pd.read_excel("附件 2:区间运行时间.xlsx") OD_flow = pd.read_excel("附件 3:OD 客流数据.xlsx") section_flow = pd.read_excel("附件 4:断面客流数据.xlsx") other_data = pd.read_excel("附件 5:其他数据.xlsx") 参数设定 w1, w2, w3, w4 = 0.25, 0.25, 0.25, 0.25 目标函数 def fitness_function(solution, solution_idx): n1, n2 = solution D1, D2 = other_data["大交路运营里程"].values[0], other_data["小交路运营里 "].values[0] C_fixed = other_data["固定成本系数"].values[0] * (n1 + n2) C_variable = other_data["变动成本系数"].values[0] * (n1 * D1 + n2 * D2) T_wait = calculate_wait_time(n1, n2) T_onboard = calculate_onboard_time(n1, n2) cost = w1 C_fixed + w2 C_variable + w3 T_wait + w4 T_onboard return 1 / cost 计算等待时间 def calculate_wait_time(n1, n2): # 假设根据实际情况计算等待时间 T_wait = 0 return T_wait 计算在车时间 def calculate_onboard_time(n1, n2): # 假设根据实际情况计算在车时间 T_onboard = 0 return T_onboard 遗传算法求解 ga_instance = GA(num_generations=100, num_parents_mating=5, fitness_func=fitness_function, sol_per_pop=10, num_genes=2, gene_space=[(1, 20), (1, 20)], parent_selection_type="rank", keep_parents=2, crossover_type="single_point", mutation_type="random", mutation_percent_genes=10) ga_instance.run() solution, solution_fitness, _ = ga_instance.best_solution() n1, n2 = int(solution[0]), int(solution[1]) print(f"安排大交路列车开行的数量:{n1}") print(f"安排小交路列车开行的数量:{n2}")
这段代码是一个交通调度问题的求解代码,主要包括以下几个步骤:
1. 导入必要的库,包括numpy、pandas、scipy.optimize和pygad。
2. 读取数据,其中包括车站数据、区间运行时间、OD客流数据、断面客流数据和其他数据。
3. 设置参数,包括权重系数和交路运营里程等。
4. 定义目标函数fitness_function,其中通过计算固定成本、变动成本、等待时间和在车时间来计算总成本,然后通过倒数的方式将总成本转化为适应度值。
5. 定义两个子函数,用于计算等待时间和在车时间。
6. 使用遗传算法求解,其中设置了一些参数,如迭代次数、种群大小、基因个数、基因空间范围、选择方式、交叉方式、变异方式等。
7. 输出最优解,即安排大交路列车开行的数量和安排小交路列车开行的数量。
总的来说,这段代码是一个比较完整的交通调度问题的求解代码,包括了数据读取、参数设置、目标函数定义、遗传算法求解等多个步骤。
阅读全文
相关推荐
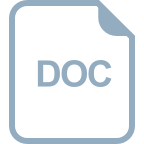
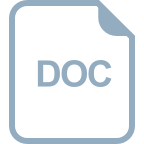
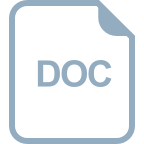
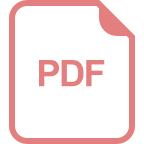
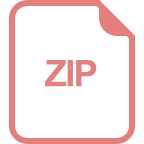
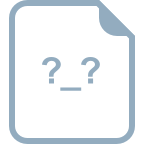
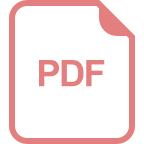
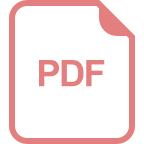
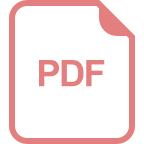
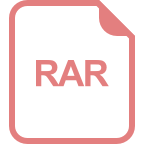
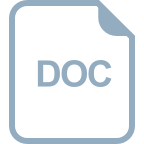
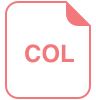




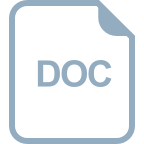