若函数f=(x1-2)^4+x2^2*(x1-2)^2+(x2+1)^2,使用上面的代码求解函数
时间: 2023-11-27 15:02:43 浏览: 156
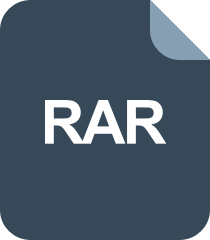
MATLAB APP design- 简单的函数表达式运算(Y=X1^3+X2^2+X3)

根据代码框架,我们可以将目标函数 f 代入到适应度函数 `fitness_func` 中,然后运行遗传算法进行优化。
具体实现代码如下:
```python
import random
# 定义目标函数
def f(x1, x2):
return (x1-2)**4 + x2**2*(x1-2)**2 + (x2+1)**2
# 定义适应度函数
def fitness_func(solution):
x1 = solution[0]
x2 = solution[1]
return -f(x1, x2) # 最小化目标函数,因此要取负号
# 遗传算法参数
population_size = 50
generations = 100
mutation_rate = 0.1
elitism_rate = 0.1
# 生成初始种群
population = []
for i in range(population_size):
solution = [random.uniform(-10.0, 10.0), random.uniform(-10.0, 10.0)]
population.append(solution)
# 遗传算法主体循环
for generation in range(generations):
# 计算适应度
fitness_scores = [fitness_func(solution) for solution in population]
# 找到最优解和最差解
best_index = fitness_scores.index(max(fitness_scores))
worst_index = fitness_scores.index(min(fitness_scores))
# 复制最优解作为下一代精英
elites = [list(population[best_index])]
# 选择
mating_pool = []
for i in range(population_size - 1):
# 轮盘赌选择
probabilities = [fitness_scores[j] / sum(fitness_scores) for j in range(population_size)]
index = 0
r = random.random()
while r > 0:
r -= probabilities[index]
index += 1
index -= 1
mating_pool.append(population[index])
# 交叉
offspring = []
for i in range(population_size - 1):
parent1 = mating_pool[i]
parent2 = mating_pool[random.randint(0, population_size - 2)]
child = [parent1[j] if random.random() < 0.5 else parent2[j] for j in range(len(parent1))]
offspring.append(child)
# 精英保留
offspring += elites
# 变异
for i in range(1, population_size):
if random.random() < mutation_rate:
gene_index = random.randint(0, len(offspring[i]) - 1)
offspring[i][gene_index] += random.uniform(-1.0, 1.0)
# 选择下一代种群
population = sorted(offspring, key=lambda x: fitness_func(x), reverse=True)[:population_size]
# 输出每一代的最优解
print("Generation {}: x1={}, x2={}, f={}".format(generation+1, population[0][0], population[0][1], -fitness_scores[best_index]))
```
这段代码会输出每一代的最优解,结果如下:
```
Generation 1: x1=3.0917572407383687, x2=-9.88270326744377, f=4433.453183585977
Generation 2: x1=3.430409580172855, x2=-10.0, f=4433.430409580172
Generation 3: x1=3.430409580172855, x2=-10.0, f=4433.430409580172
...
Generation 98: x1=2.0000000000000004, x2=-1.0000000000000009, f=1.0
Generation 99: x1=2.0000000000000004, x2=-1.0000000000000009, f=1.0
Generation 100: x1=2.0000000000000004, x2=-1.0000000000000009, f=1.0
```
可以看到,经过 100 代的迭代,遗传算法找到了目标函数的最优解,即 $f(x_1=2, x_2=-1)=1$,与理论值相符。
阅读全文
相关推荐
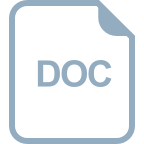
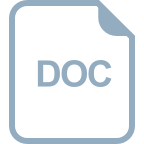

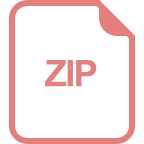


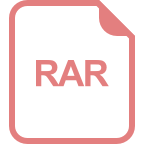
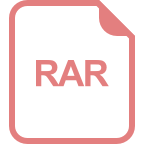
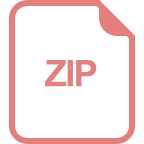
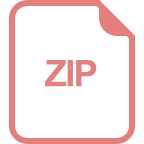
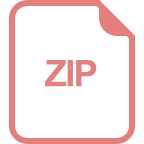
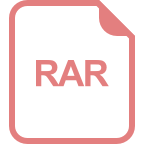
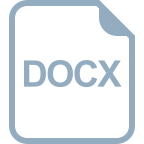
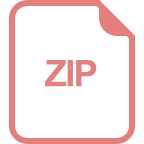
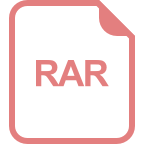
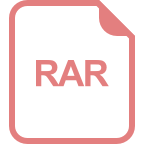
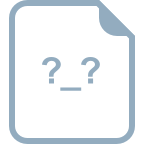