class BufferedSequential(nn.Module): def __init__(self, layers, buffer_or_not): super(BufferedSequential, self).__init__() self.layers = layers self.buffer_or_not = buffer_or_not self.n_buffers = np.sum(buffer_or_not) def parameters(self): return [param for layer in self.layers for param in layer.parameters()] def forward(self, x): if not isinstance(x, Variable): if not isinstance(x, torch.Tensor): x = torch.Tensor(x) x = Variable(x) self.buffer = [] for layer, is_buffered in zip(self.layers, self.buffer_or_not): x = layer(x) if is_buffered: self.buffer.append(x) return x
时间: 2024-02-14 22:31:06 浏览: 27
这段代码定义了一个名为 `BufferedSequential` 的自定义神经网络模块。它接受两个参数:`layers` 和 `buffer_or_not`。`layers` 是一个包含多个层的列表,`buffer_or_not` 是一个布尔值的列表,用于指示每个层是否需要缓存输出。
在 `__init__` 方法中,它首先调用父类的 `__init__` 方法进行初始化,然后将传入的 `layers` 和 `buffer_or_not` 参数保存在对象的属性中。`n_buffers` 属性是通过统计 `buffer_or_not` 列表中为 True 的元素数量来计算的。
`parameters` 方法返回模块中所有层的参数。它通过遍历 `layers` 列表和每个层的参数,将所有参数收集到一个列表中并返回。
`forward` 方法定义了前向传播的逻辑。它接受输入 `x` 并将其转换为 `torch.Tensor` 类型的变量。然后,它创建了一个空的列表 `self.buffer` 用于存储缓存的输出。
接下来,它使用 `zip` 函数迭代 `layers` 和 `buffer_or_not` 列表,并对每个层进行前向传播。如果当前层需要缓存输出,则将输出添加到 `self.buffer` 列表中。
最后,它返回最后一个层的输出 `x`。
这段代码的作用是在神经网络模型中,允许选择性地缓存某些层的输出,以便后续的操作可以使用这些缓存的值。
相关问题
class LinearMaskedCoupling(nn.Module): """ Coupling Layers """ def __init__(self, input_size, hidden_size, n_hidden, mask, cond_label_size=None): super().__init__() # stored in state_dict, but not trained & not returned by nn.parameters(); similar purpose as nn.Parameter objects # this is because tensors won't be saved in state_dict and won't be pushed to the device self.register_buffer('mask', mask) # 0,1,0,1 # scale function # for conditional version, just concat label as the input into the network (conditional way of SRMD) s_net = [nn.Linear(input_size + (cond_label_size if cond_label_size is not None else 0), hidden_size)] for _ in range(n_hidden): s_net += [nn.Tanh(), nn.Linear(hidden_size, hidden_size)] s_net += [nn.Tanh(), nn.Linear(hidden_size, input_size)] self.s_net = nn.Sequential(*s_net) # translation function, the same structure self.t_net = copy.deepcopy(self.s_net) # replace Tanh with ReLU's per MAF paper for i in range(len(self.t_net)): if not isinstance(self.t_net[i], nn.Linear): self.t_net[i] = nn.ReLU() def forward(self, x, y=None): # apply mask mx = x * self.mask # run through model log_s = self.s_net(mx if y is None else torch.cat([y, mx], dim=1)) t = self.t_net(mx if y is None else torch.cat([y, mx], dim=1)) u = mx + (1 - self.mask) * (x - t) * torch.exp( -log_s) # cf RealNVP eq 8 where u corresponds to x (here we're modeling u) log_abs_det_jacobian = (- (1 - self.mask) * log_s).sum( 1) # log det du/dx; cf RealNVP 8 and 6; note, sum over input_size done at model log_prob return u, log_abs_det_jacobian 帮我解析代码
这段代码定义了一个名为LinearMaskedCoupling的类,该类继承自nn.Module。LinearMaskedCoupling是一种coupling层,用于在流式生成模型中实现可逆转换。下面对代码进行解析:
在__init__方法中,有以下几个参数:
- input_size:输入数据的维度大小
- hidden_size:隐藏层的维度大小
- n_hidden:隐藏层的数量
- mask:掩码,用于指定哪些输入特征是要保留的(值为1),哪些是要忽略的(值为0)
- cond_label_size:条件标签的维度大小,用于条件版本的生成(可选参数,默认为None)
在__init__方法中,首先调用父类nn.Module的构造函数super().__init__()。然后使用register_buffer方法注册了一个名为mask的缓冲区(buffer),用于存储掩码。这样做的目的是将mask保存在模型的state_dict中,但不作为需要训练的参数返回。
接下来定义了scale function和translation function。两者具有相同的结构,都是由一系列线性层和激活函数组成。其中,scale function用于计算尺度参数log_s,translation function用于计算平移参数t。
在forward方法中,首先根据掩码对输入x进行掩码操作,得到mx。然后根据是否有条件标签y,将mx与y进行拼接,作为输入传递给scale function和translation function,分别计算尺度参数log_s和平移参数t。
最后,根据RealNVP论文中的公式,通过对mx进行逆变换操作,得到u。同时计算对数绝对行列式的Jacobian矩阵(log_abs_det_jacobian)。
最终,函数返回u和log_abs_det_jacobian。
import torch class Network(torch.nn.Module): def __init__(self, n_fft=1024, n_hop=160, n_hidden=1024): super().__init__() self.linear1 = torch.nn.LSTM(n_fft//2+1, n_hidden//2, num_layers=2, batch_first=True, bidirectional=True) self.linear2 = torch.nn.Linear(n_hidden, n_fft//2+1) self.n_fft = n_fft self.n_hop = n_hop # self.window = self.register_buffer('window', torch.hann_window(n_fft)) def forward(self, noisy): # 傅里叶变换 noisy_spec = torch.stft(noisy, self.n_fft, self.n_hop, window=self.window, return_complex=True) # [B,C,T] noisy_amplitude = torch.abs(noisy_spec).transpose(2,1) # [B,T,C=513] # 神经网络计算 hidden = self.linear1(noisy_amplitude)[0] # [B,T,n_hidden=1024] mask = self.linear2(hidden).sigmoid() # [B,T,C=513] (0,1) # 降噪结果 denoise_spec = noisy_spec * mask.transpose(2,1) denoise_wav = torch.istft(denoise_spec, self.n_fft, self.n_hop, window=self.window) # 返回降噪后波形和降噪后谱图 return denoise_wav, denoise_spec if __name__ == "__main__": net = Network() clean = torch.randn(4, 16000) noise = torch.randn(4, 16000) denoise_wav, denoise_spec = net(clean + noise) print(denoise_spec.shape, denoise_wav.shape)
这段代码实现了一个基于LSTM的音频降噪网络,其核心思路是使用LSTM对音频信号的幅度谱进行处理,从而得到一个掩膜(mask),该掩膜可以在频域上降噪信号。具体来说,该网络的输入是含有噪声的干净音频信号,经过傅里叶变换得到幅度谱,之后通过LSTM计算得到一个掩膜,最后将该掩膜应用于幅度谱上,得到降噪后的幅度谱,再通过逆傅里叶变换得到降噪后的音频信号。
具体来说,该网络包括以下几个部分:
- `__init__`函数:定义了一个两层的LSTM网络和一个线性层(用于将LSTM输出的隐藏状态转换为掩膜),并初始化了窗函数等参数。
- `forward`函数:实现了整个网络的前向传播过程。首先对输入信号进行傅里叶变换,得到幅度谱,之后将幅度谱通过LSTM处理得到一个掩膜,再将该掩膜应用于幅度谱上,得到降噪后的幅度谱,最后通过逆傅里叶变换得到降噪后的音频信号。
- `if __name__ == "__main__"`:对网络的功能进行测试,输入为一个随机产生的4秒钟的干净音频信号和一个随机产生的4秒钟的噪声信号,输出为降噪后的幅度谱和音频信号。
需要注意的是,由于该网络使用了LSTM,其计算成本较高,需要在计算资源允许的情况下使用。此外,该网络的性能也取决于数据集的质量和训练过程的参数设置等因素。
相关推荐
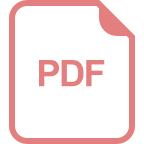












