def plot(self): self.is_running = False pwd_path = os.path.abspath(os.path.join(os.getcwd(), "..")) script_path = os.path.join(pwd_path, 'callisto-test-tool\\algo_galileo_2e235eb933c9_deg90\\An_test') folder_path = os.path.join(pwd_path, 'callisto-test-tool\\source\\Test_Source_Data') os.chdir(script_path) os.system('powershell -Command "./csv_output.sh {0}"'.format(folder_path)) 怎么检测该段程序是否运行完毕
时间: 2024-02-13 21:03:29 浏览: 25
可以在程序运行完毕后,使用一个标志变量来表示程序已经运行完毕,例如在该段程序中,可以在最后添加一行代码 `self.is_finished = True` 来表示程序已经运行完毕。然后在调用该程序的地方,可以使用一个循环来不断检测该标志变量是否为True,直到程序运行完毕才继续执行后面的代码。例如:
```
chitgpt.plot() # 调用程序
# 检测程序是否运行完毕
while not chitgpt.is_finished:
time.sleep(1) # 等待1秒钟
continue
# 程序已经运行完毕,继续执行后面的代码
print("程序已经运行完毕!")
```
这样就可以检测该段程序是否运行完毕了。需要注意的是,在使用循环等待程序运行完毕时,需要添加一些等待时间,否则可能会出现死循环的情况。
相关问题
def plot(self): self.is_running = False pwd_path = os.path.abspath(os.path.join(os.getcwd(), "..")) script_path = os.path.join(pwd_path, 'callisto-test-tool\\algo_galileo_2e235eb933c9_deg90\\An_test') folder_path = os.path.join(pwd_path, 'callisto-test-tool\\source\\Test_Source_Data') os.chdir(script_path) os.system('powershell -Command "./csv_output.sh {0}"'.format(folder_path)) 怎么检测该段程序中powershell是否运行完毕
要检测该段程序中 `powershell` 是否运行完毕,可以使用 Python 的 `subprocess` 模块来代替 `os.system` 函数来运行 `powershell`,并使用 `communicate()` 方法来等待 `powershell` 进程结束。例如:
```
import subprocess
def plot(self):
self.is_running = False
pwd_path = os.path.abspath(os.path.join(os.getcwd(), ".."))
script_path = os.path.join(pwd_path, 'callisto-test-tool\\algo_galileo_2e235eb933c9_deg90\\An_test')
folder_path = os.path.join(pwd_path, 'callisto-test-tool\\source\\Test_Source_Data')
os.chdir(script_path)
cmd = 'powershell -Command "./csv_output.sh {0}"'.format(folder_path)
process = subprocess.Popen(cmd, shell=True, stdout=subprocess.PIPE, stderr=subprocess.PIPE)
stdout, stderr = process.communicate() # 等待进程结束
if process.returncode == 0:
print('程序执行成功!')
else:
print('程序执行失败!')
```
这样就可以在程序执行完成后得到 `powershell` 进程的返回值,并根据返回值来判断程序是否执行成功。如果返回值为 0,则表示程序执行成功,否则表示程序执行失败。
def plot(self): self.is_running = False pwd_path = os.getcwd() script_path = os.path.join(pwd_path, 'algo_galileo_2e235eb933c9_deg90\\An_test') folder_path = os.path.join(pwd_path, 'source\\Test_Source_Data') os.chdir(script_path) os.system('powershell -Command "./csv_output.sh {0}"'.format(folder_path)) time.sleep(30) 这段代码中,怎么把路径pwd_path改为os.getcwd()的上级目录
可以使用os.path.abspath(os.path.join(os.getcwd(), ".."))来获取当前工作目录的上级目录,然后将其赋值给pwd_path。所以,可以将第三行代码改为:
```
pwd_path = os.path.abspath(os.path.join(os.getcwd(), ".."))
```
这样就能获取到当前工作目录的上级目录了。
相关推荐
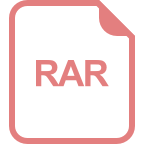













