paddle.concat([25, 10], axis=1)
时间: 2024-04-12 12:29:24 浏览: 15
`paddle.concat` 函数用于将多个张量沿指定的维度拼接在一起。但是根据你给出的示例代码 `paddle.concat([25, 10], axis=1)`,存在一些错误。这里是正确的示例代码:
```python
import paddle
x = paddle.to_tensor([25])
y = paddle.to_tensor([10])
result = paddle.concat([x, y], axis=1)
print(result)
```
在上述代码中,我们首先使用 `paddle.to_tensor` 函数将列表 `[25]` 和 `[10]` 转换为 PaddlePaddle 的张量对象 `x` 和 `y`。然后,我们使用 `paddle.concat` 函数将这两个张量沿着 `axis=1` 的维度进行拼接。最后,我们打印输出结果。
拼接的结果将会是一个形状为 `(1, 2)` 的张量,其中第一个维度大小为 1,第二个维度大小为 2。输出结果将是 `[[25, 10]]`。
请注意,拼接操作的维度索引从0开始,所以 `axis=1` 表示沿着第二个维度进行拼接。
相关问题
请在这个DeepCFD的网络添加attention机制,并给出示例代码:import paddle import paddle.nn as nn class Attention(nn.Layer): def __init__(self, input_size, hidden_size): super(Attention, self).__init__() self.input_size = input_size self.hidden_size = hidden_size self.attn = nn.Linear(self.input_size + self.hidden_size, 1) self.softmax = nn.Softmax(axis=1) def forward(self, input, hidden): max_len = input.shape[0] attn_energies = paddle.zeros([max_len, 1]) for i in range(max_len): attn_energies[i] = self.score(input[i], hidden) attn_weights = self.softmax(attn_energies) context = paddle.sum(attn_weights * input, axis=0) return context.unsqueeze(0) def score(self, input, hidden): energy = self.attn(paddle.concat([input, hidden], axis=1)) return energy class DeepCFD(nn.Layer): def __init__(self, input_size, hidden_size, output_size): super(DeepCFD, self).__init__() self.input_size = input_size self.hidden_size = hidden_size self.output_size = output_size self.lstm = nn.LSTM(input_size, hidden_size, num_layers=2, batch_first=True) self.attention = Attention(input_size, hidden_size) self.fc = nn.Linear(hidden_size, output_size) def forward(self, input): output, (hidden, cell) = self.lstm(input) context = self.attention(output, hidden[-1]) output = self.fc(context) return output
好的,下面是添加了Attention机制的DeepCFD网络的示例代码:
import paddle
import paddle.nn as nn
class Attention(nn.Layer):
def __init__(self, input_size, hidden_size):
super(Attention, self).__init__()
self.input_size = input_size
self.hidden_size = hidden_size
self.attn = nn.Linear(self.input_size + self.hidden_size, 1)
self.softmax = nn.Softmax(axis=1)
def forward(self, input, hidden):
max_len = input.shape[1]
attn_energies = paddle.zeros([input.shape[0], max_len, 1])
for i in range(max_len):
attn_energies[:, i] = self.score(input[:, i, :], hidden)
attn_weights = self.softmax(attn_energies)
context = paddle.sum(attn_weights * input, axis=1)
return context
def score(self, input, hidden):
energy = self.attn(paddle.concat([input, hidden], axis=1))
return energy
class DeepCFD(nn.Layer):
def __init__(self, input_size, hidden_size, output_size):
super(DeepCFD, self).__init__()
self.input_size = input_size
self.hidden_size = hidden_size
self.output_size = output_size
self.lstm = nn.LSTM(input_size, hidden_size, num_layers=2, batch_first=True)
self.attention = Attention(input_size, hidden_size)
self.fc = nn.Linear(hidden_size, output_size)
def forward(self, input):
output, (hidden, cell) = self.lstm(input)
context = self.attention(output, hidden[-1])
output = self.fc(context)
return output
在这个示例代码中,我们将Attention机制应用到了LSTM的输出上。在Attention中,我们计算了每个时间步的注意力能量,然后使用softmax函数计算注意力权重。然后,我们将这些权重与LSTM输出相乘并求和,得到上下文向量作为Attention机制的输出。
在DeepCFD中,我们使用了两层LSTM,然后将LSTM输出和最后一个时刻的隐藏状态作为Attention机制的输入。最后,我们将Attention机制的输出传递到一个全连接层中,得到最终的输出。
解释每一句 def forward(self, x): if self.conv_down is not None: x = self.conv_down(x) y1 = self.conv1(x) y2 = self.blocks(self.conv2(x)) y = paddle.concat([y1, y2], axis=1) if self.attn is not None: y = self.attn(y) y = self.conv3(y) return y
这是一个类的前向传播函数,用于执行输入数据的正向传递过程。具体的解释如下:
```
def forward(self, x):
```
定义了一个前向传播函数,输入为x。
```
if self.conv_down is not None:
x = self.conv_down(x)
```
如果self.conv_down不为空,则将输入x执行一次卷积操作self.conv_down。
```
y1 = self.conv1(x)
```
执行第一步卷积操作,将输入x执行一次卷积操作self.conv1,输出为y1。
```
y2 = self.blocks(self.conv2(x))
```
执行第二步卷积操作,将输入x执行一次卷积操作self.conv2,并将输出作为参数传递给self.blocks函数,输出为y2。
```
y = paddle.concat([y1, y2], axis=1)
```
将y1和y2按照轴axis=1进行拼接,输出为y。
```
if self.attn is not None:
y = self.attn(y)
```
如果self.attn不为空,则将y执行一次注意力机制操作self.attn。
```
y = self.conv3(y)
```
执行最后一步卷积操作,将y执行一次卷积操作self.conv3,输出为y。
```
return y
```
返回最终输出的y。
相关推荐
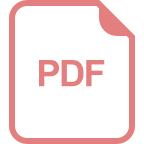
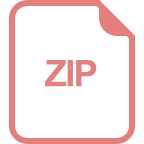
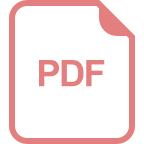












