选择一种聚类算法评价指标评价OPTICS算法结果,给出具体代码
时间: 2024-10-17 12:10:22 浏览: 32
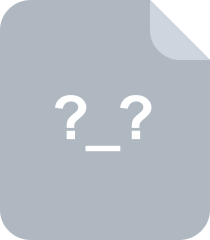
基于密度的聚类算法optics(matlab程序)。

在评估 OPTICS (Ordering Points To Identify the Clustering Structure) 算法的结果时,常用的指标之一是 DBSCAN(Density-Based Spatial Clustering of Applications with Noise)的核心半径 (Eps) 和邻域大小 (MinPts),因为它们与 OPTICS 中的 reachability distance 和 ordering 相对应。然而,由于 OPTICS本身并不直接输出核心半径和邻域大小,我们通常会结合其产生的 Reachability Plot 或者 Local Reachability Density (LRD) 来分析。
一种简单的评价方法是对发现的高密度区域(核心点和可达区域)进行比较,看是否符合预期的簇结构。你可以使用以下 Python 示例来评估:
```python
from sklearn.cluster import OPTICS
import matplotlib.pyplot as plt
# 假设你已经有了预处理的数据 points
points = ...
# 使用 OPTICS
clust = OPTICS(min_samples=5, eps=0.5)
clust.fit(points)
# 获取 reachability 和 ordering 资料
reachability = clust.reachability_
ordering = clust.ordering_
# 可视化 Reachability Plot
plt.figure(figsize=(10, 6))
plt.plot(ordering, reachability, marker='o')
plt.xlabel('Index')
plt.ylabel('Reachability Distance')
plt.title('Reachability Plot for OPTICS')
# 检查是否存在明确的聚集区域
core_distances, densities = clust.core_distances_, clust.min_cluster_size_
clusters = [i for i in range(len(densities)) if densities[i] >= min_pts]
# 对每个簇进行评价
for cluster_id in clusters:
core_points = points[ordering[cluster_id]:ordering[cluster_id + 1]]
print(f"Cluster {cluster_id+1}: Core radius = {core_distances[cluster_id]}, MinPts = {min_pts}")
# 结论部分(
阅读全文
相关推荐
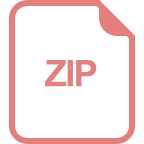
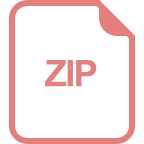
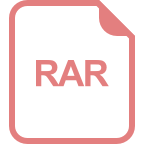
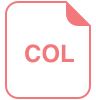




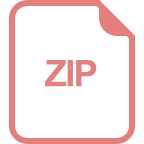
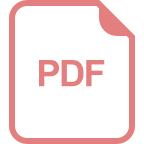
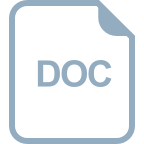






