使用sklearn和遗传算法的特征选择python
时间: 2023-08-09 07:09:33 浏览: 92
可以使用遗传算法和sklearn库来进行特征选择。下面是一个使用遗传算法和sklearn库的特征选择示例:
首先,你需要导入相关的库:
```python
import numpy as np
from sklearn.datasets import load_breast_cancer
from sklearn.model_selection import train_test_split
from sklearn.preprocessing import StandardScaler
from sklearn.svm import SVC
from sklearn.metrics import accuracy_score
import matplotlib.pyplot as plt
```
然后,你需要加载数据集并将其划分为训练集和测试集:
```python
# 加载数据集
data = load_breast_cancer()
# 将数据集划分为训练集和测试集
X_train, X_test, y_train, y_test = train_test_split(data.data, data.target, test_size=0.3, random_state=42)
```
接下来,你需要对数据进行缩放,以便更好地使用SVM分类器:
```python
# 对数据进行缩放
scaler = StandardScaler()
X_train_scaled = scaler.fit_transform(X_train)
X_test_scaled = scaler.transform(X_test)
```
然后,你需要定义一个函数来计算分类器的准确性:
```python
# 计算分类器的准确性
def get_accuracy(X_train, X_test, y_train, y_test, selected_features):
clf = SVC(kernel='linear')
clf.fit(X_train[:, selected_features], y_train)
y_pred = clf.predict(X_test[:, selected_features])
return accuracy_score(y_test, y_pred)
```
接下来,你需要定义一个遗传算法来进行特征选择。在这个例子中,我们将使用遗传算法来选择前10个最佳特征:
```python
# 定义遗传算法来进行特征选择
def genetic_algorithm():
# 定义遗传算法的参数
population_size = 100
num_generations = 50
mutation_rate = 0.1
num_features = X_train_scaled.shape[1]
num_selected_features = 10
# 初始化种群
population = np.random.randint(2, size=(population_size, num_features))
# 定义每代的最佳个体和最佳适应度值
best_individual = None
best_fitness = -1
# 进化种群
for generation in range(num_generations):
# 计算每个个体的适应度值
fitness = np.zeros(population_size)
for i in range(population_size):
fitness[i] = get_accuracy(X_train_scaled, X_test_scaled, y_train, y_test, np.where(population[i] == 1)[0])
if fitness[i] > best_fitness:
best_fitness = fitness[i]
best_individual = population[i]
# 选择父代
parent1 = population[np.random.choice(range(population_size), size=population_size, replace=True), :]
parent2 = population[np.random.choice(range(population_size), size=population_size, replace=True), :]
# 交叉操作
crossover_point = np.random.randint(num_features, size=population_size)
offspring1 = np.zeros((population_size, num_features))
offspring2 = np.zeros((population_size, num_features))
for i in range(population_size):
offspring1[i, :crossover_point[i]] = parent1[i, :crossover_point[i]]
offspring1[i, crossover_point[i]:] = parent2[i, crossover_point[i]:]
offspring2[i, :crossover_point[i]] = parent2[i, :crossover_point[i]]
offspring2[i, crossover_point[i]:] = parent1[i, crossover_point[i]:]
# 突变操作
mutation_mask = np.random.uniform(0, 1, (population_size, num_features)) < mutation_rate
offspring1[mutation_mask] = 1 - offspring1[mutation_mask]
offspring2[mutation_mask] = 1 - offspring2[mutation_mask]
# 合并父代和子代
population = np.concatenate((parent1, parent2, offspring1, offspring2))
# 选择最佳个体
fitness = np.zeros(population.shape[0])
for i in range(population.shape[0]):
fitness[i] = get_accuracy(X_train_scaled, X_test_scaled, y_train, y_test, np.where(population[i] == 1)[0])
if fitness[i] > best_fitness:
best_fitness = fitness[i]
best_individual = population[i]
# 选择最佳个体作为下一代的种群
population = population[np.argsort(fitness)[::-1][:population_size], :]
return best_individual
```
最后,你可以使用matplotlib库来可视化选定的特征:
```python
# 运行遗传算法并获取最佳个体
best_individual = genetic_algorithm()
# 获取选定的特征
selected_features = np.where(best_individual == 1)[0]
# 绘制选定的特征
plt.bar(range(len(selected_features)), data.data[:, selected_features].mean(axis=0))
plt.xticks(range(len(selected_features)), data.feature_names[selected_features], rotation=90)
plt.xlabel('Feature')
plt.ylabel('Mean')
plt.show()
```
这个示例使用SVM分类器和遗传算法来进行特征选择。你可以根据需要更改分类器或算法。
相关推荐
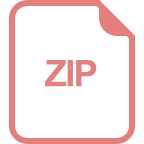
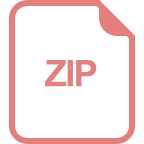
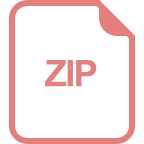














