使用bert-base-chinese训练实体识别模型的代码示例
时间: 2023-10-25 16:10:16 浏览: 182
以下是使用bert-base-chinese训练实体识别模型的代码示例:
1. 准备数据集
首先,需要准备实体识别任务的数据集。数据集应该包含标记好的实体标签,例如“B-PER”和“I-PER”表示人名实体的开始和内部标记。
2. 定义模型
定义一个使用bert-base-chinese预训练模型的实体识别模型,可以使用PyTorch和Transformers库:
```python
import torch
from transformers import BertForTokenClassification, BertTokenizer
model = BertForTokenClassification.from_pretrained('bert-base-chinese', num_labels=5)
tokenizer = BertTokenizer.from_pretrained('bert-base-chinese')
```
在这里,我们使用“num_labels”参数指定模型输出的标签数,即实体类别数。
3. 定义训练循环
接下来,我们定义训练循环来训练我们的模型:
```python
from torch.utils.data import DataLoader, RandomSampler, SequentialSampler
from transformers import AdamW, get_linear_schedule_with_warmup
device = torch.device("cuda" if torch.cuda.is_available() else "cpu")
model.to(device)
train_data = ... # 加载训练数据集
train_sampler = RandomSampler(train_data)
train_dataloader = DataLoader(train_data, sampler=train_sampler, batch_size=32)
optimizer = AdamW(model.parameters(), lr=5e-5, eps=1e-8)
total_steps = len(train_dataloader) * 3
scheduler = get_linear_schedule_with_warmup(optimizer, num_warmup_steps=0, num_training_steps=total_steps)
for epoch in range(3):
for batch in train_dataloader:
model.train()
batch = tuple(t.to(device) for t in batch)
inputs = {'input_ids': batch[0], 'attention_mask': batch[1], 'labels': batch[3]}
outputs = model(**inputs)
loss = outputs[0]
loss.backward()
torch.nn.utils.clip_grad_norm_(model.parameters(), 1.0)
optimizer.step()
scheduler.step()
optimizer.zero_grad()
```
在这里,我们使用随机采样器将训练数据集的数据随机分成小批次。我们使用AdamW优化器和带有线性学习率调度程序的预热来训练模型。在每个时期内,我们遍历每个小批次并计算损失和梯度,然后更新模型参数。
4. 评估模型
训练完成后,我们可以使用测试数据集来评估模型的性能:
```python
test_data = ... # 加载测试数据集
test_sampler = SequentialSampler(test_data)
test_dataloader = DataLoader(test_data, sampler=test_sampler, batch_size=32)
model.eval()
predictions = []
true_labels = []
for batch in test_dataloader:
batch = tuple(t.to(device) for t in batch)
inputs = {'input_ids': batch[0], 'attention_mask': batch[1], 'labels': batch[3]}
with torch.no_grad():
outputs = model(**inputs)
logits = outputs[1].detach().cpu().numpy()
label_ids = inputs['labels'].cpu().numpy()
predictions.extend([list(p) for p in np.argmax(logits, axis=2)])
true_labels.extend(label_ids)
from sklearn.metrics import f1_score
f1_score = f1_score(true_labels, predictions, average='weighted')
print("F1 score:", f1_score)
```
在这里,我们将测试数据集分成小批次,并使用模型的“eval”方法来计算预测标签。然后,我们使用f1_score度量来评估模型性能。
这就是使用bert-base-chinese训练实体识别模型的代码示例。
阅读全文
相关推荐
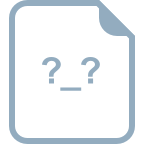
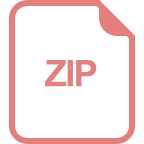
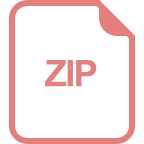

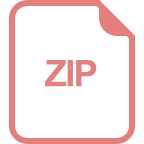
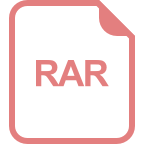


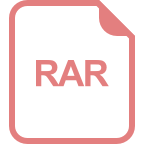
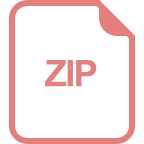
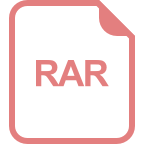
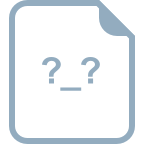
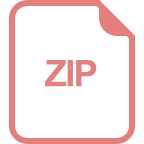
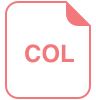
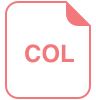
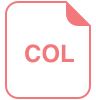
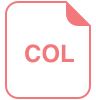
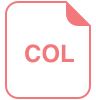
