acf,pacf判断是否平稳实例
时间: 2024-04-13 13:30:08 浏览: 95
下面是一个示例代码,用于使用自相关函数(ACF)和偏自相关函数(PACF)来判断时间序列数据是否平稳:
```python
import numpy as np
import pandas as pd
import matplotlib.pyplot as plt
from statsmodels.graphics.tsaplots import plot_acf, plot_pacf
# 生成一个非平稳时间序列数据
np.random.seed(0)
data = np.cumsum(np.random.normal(loc=0, scale=1, size=100))
# 绘制时间序列图
plt.figure(figsize=(10, 4))
plt.plot(data)
plt.xlabel('Time')
plt.ylabel('Value')
plt.title('Non-stationary Time Series')
plt.show()
# 计算ACF和PACF
acf_plot = plot_acf(data, lags=20)
pacf_plot = plot_pacf(data, lags=20)
# 判断平稳性
acf_values = acf_plot[0]
pacf_values = pacf_plot[0]
# 平稳性判断条件:ACF和PACF都需在置信区间内
is_stationary = all(acf_values < 1.96/np.sqrt(len(data))) and all(pacf_values < 1.96/np.sqrt(len(data)))
if is_stationary:
print("The time series is stationary.")
else:
print("The time series is non-stationary.")
```
在这个示例中,我们首先生成一个非平稳的时间序列数据。然后,使用Matplotlib库绘制时间序列图。接下来,我们使用statsmodels库中的plot_acf和plot_pacf函数来计算并绘制ACF和PACF。最后,我们判断时间序列数据是否平稳,根据ACF和PACF的值是否都在置信区间内。如果所有的ACF和PACF值都在置信区间内,则认为时间序列是平稳的。
阅读全文
相关推荐
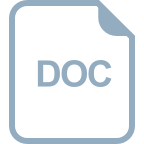
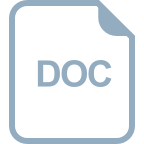
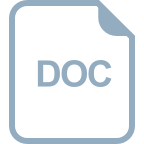
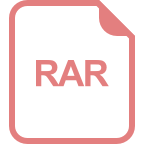
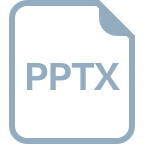
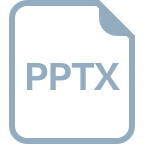
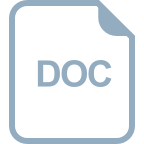
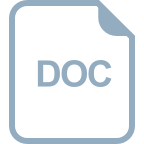
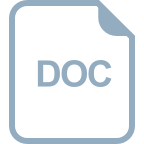
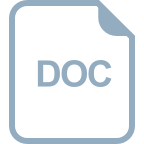
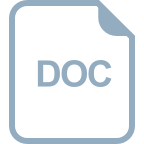
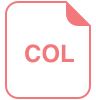






