指出代码的错误:from sklearn.feature_extraction.text import CountVectorizer vect = CountVectorizer() vect_train=vect.fit_transform(x_train) from sklearn.feature_extraction.text import TfidfVectorizer tfidf=TfidfVectorizer() tfidf_train=tfidf.fit_transform(vect_train)
时间: 2023-03-09 15:53:03 浏览: 208
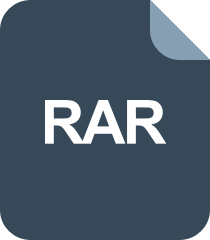
feat_extr.rar_.ana_extr_extraction_feature extraction_feature_ex
看起来你把CountVectorizer和TfidfVectorizer混淆了,应该把tfidf_train=tfidf.fit_transform(x_train)而不是tfidf_train=tfidf.fit_transform(vect_train)。
阅读全文