模糊识别算法Pyhton
时间: 2023-05-12 07:03:36 浏览: 145
可以使用模糊匹配算法FuzzyWuzzy库来实现模糊识别,该库可以计算两个字符串之间的相似度,并返回一个匹配分数。在Python中,可以使用以下代码导入FuzzyWuzzy库:
from fuzzywuzzy import fuzz
然后,可以使用fuzz.ratio()函数来计算两个字符串之间的相似度,例如:
score = fuzz.ratio('hello world', 'hello')
这将返回一个分数,表示“hello world”和“hello”之间的相似度。
相关问题
遗传算法pyhton
遗传算法(Genetic Algorithm, GA)是一种模拟自然选择过程的优化搜索算法[^1]。在Python中,我们可以用它来解决旅行商问题(Traveling Salesman Problem, TSP)。以下是一个基本的遗传算法解决TSP问题的示例:
1. **初始化**:创建一个初始种群,每个个体代表一个可能的解决方案(路线),通常通过随机生成。
```python
import random
def generate_route(n_cities):
route = [i for i in range(1, n_cities + 1)]
random.shuffle(route)
return route
initial_population = [generate_route(n_cities) for _ in range(population_size)]
```
2. **适应度评估**:计算每个个体(路线)的总距离,作为其适应度。
```python
def fitness(route, distance_matrix):
total_distance = sum(distance_matrix[i][route[i % len(route)]] for i in range(len(route)))
return 1 / total_distance
fitness_values = [fitness(route, distance_matrix) for route in initial_population]
```
3. **选择**:基于适应度选择部分个体进入下一代。常用的选择策略有轮盘赌选择(roulette wheel selection)或锦标赛选择(tournament selection)。
4. **交叉**:通过交叉操作结合父母的基因,产生新的个体。常用的交叉方式有单点交叉(one-point crossover)。
```python
def single_point_crossover(parent1, parent2, crossover_point):
child1 = parent1[:crossover_point] + parent2[crossover_point:]
child2 = parent2[:crossover_point] + parent1[crossover_point:]
return child1, child2
```
5. **变异**:对新产生的个体进行变异,增加多样性。如交换两个位置的操作(uniform crossover 或 bit-flip mutation)。
6. **重复**:直到达到停止条件(如迭代次数或适应度阈值),或者新一代的最优解优于上一代。
```python
while not stopping_condition:
next_generation = []
... (selection, crossover, and mutation steps are performed here ...)
# 检查是否有新的最优解
if any(fitness(new_gen, distance_matrix) > best_fitness for new_gen in next_generation):
best_solution = max(next_generation, key=fitness)
```
这个框架展示了遗传算法的基本概念和实施步骤,但实际应用时需要细化并调整参数以获得更好的性能。
遗传算法PYHTON
遗传算法是一种模拟生物进化过程的优化算法,常用于解决搜索和优化问题。在 Python 中,可以使用遗传算法库来实现遗传算法的相关功能。
一个常用的遗传算法库是 DEAP(Distributed Evolutionary Algorithms in Python),它提供了丰富的遗传算法工具和操作符。你可以通过以下步骤来安装 DEAP:
1. 安装 DEAP:在命令行中运行 `pip install deap`。
安装完成后,你可以使用 DEAP 库构建遗传算法模型。一般来说,遗传算法包括以下步骤:
1. 定义问题的适应度函数。
2. 定义问题的变量编码方式。
3. 初始化种群。
4. 选择优秀个体。
5. 进行交叉和变异操作。
6. 重复步骤4和5,直到达到停止条件。
7. 输出最优解。
具体实现遗传算法的代码示例如下:
```python
import random
from deap import base, creator, tools
# 定义问题的适应度函数
def evaluate(individual):
# 自定义适应度函数逻辑
return fitness
# 定义问题的变量编码方式
creator.create("FitnessMax", base.Fitness, weights=(1.0,))
creator.create("Individual", list, fitness=creator.FitnessMax)
toolbox = base.Toolbox()
# 注册变量和适应度函数到 toolbox
toolbox.register("attribute", random.random)
toolbox.register("individual", tools.initRepeat, creator.Individual, toolbox.attribute, n=10)
toolbox.register("population", tools.initRepeat, list, toolbox.individual)
toolbox.register("evaluate", evaluate)
toolbox.register("mate", tools.cxTwoPoint)
toolbox.register("mutate", tools.mutGaussian, mu=0, sigma=1, indpb=0.1)
toolbox.register("select", tools.selTournament, tournsize=3)
# 初始化种群
population = toolbox.population(n=100)
# 进行遗传算法迭代
for generation in range(100):
# 选择优秀个体
offspring = toolbox.select(population, k=len(population))
# 进行交叉和变异操作
offspring = [toolbox.mate(ind1, ind2) for ind1, ind2 in zip(offspring[::2], offspring[1::2])]
offspring = [toolbox.mutate(ind) for ind in offspring]
# 评估适应度函数
fits = toolbox.map(toolbox.evaluate, offspring)
for fit, ind in zip(fits, offspring):
ind.fitness.values = (fit,)
# 更新种群
population[:] = offspring
# 输出最优解
best_individual = tools.selBest(population, k=1)[0]
print("Best individual:", best_individual)
```
注意:以上代码只是一个简单的示例,你需要根据具体问题的要求来定义适应度函数和变量编码方式,并调整其他参数以满足实际需求。希望对你有所帮助!如需更多信息,请查阅 DEAP 库的官方文档。
阅读全文
相关推荐
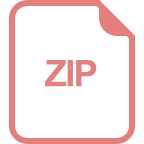
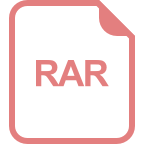













