定义一个损失函数,在mse的基础上加入稀疏约束项和权重衰减项用于一维稀疏自编码器keras
时间: 2024-02-01 20:17:02 浏览: 79
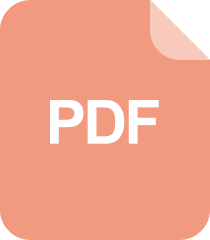
Keras之自定义损失(loss)函数用法说明
好的,下面是一个一维稀疏自编码器的Keras模型,其中包含了带有L1稀疏约束和L2权重衰减的损失函数:
```python
from keras.layers import Input, Dense
from keras.models import Model
from keras import regularizers
# 定义输入层
input_layer = Input(shape=(num_features,))
# 定义编码层
encoded = Dense(encoding_dim, activation='relu',
kernel_regularizer=regularizers.l2(0.001),
activity_regularizer=regularizers.l1(10e-5))(input_layer)
# 定义解码层
decoded = Dense(num_features, activation='sigmoid')(encoded)
# 定义自编码器模型
autoencoder = Model(input_layer, decoded)
# 定义编码器模型
encoder = Model(input_layer, encoded)
# 定义损失函数
def custom_loss(y_true, y_pred):
mse_loss = K.mean(K.square(y_true - y_pred), axis=-1)
sparse_loss = K.sum(K.abs(encoded), axis=-1)
total_loss = mse_loss + 0.01 * sparse_loss
return total_loss
# 编译自编码器模型
autoencoder.compile(optimizer='adam', loss=custom_loss)
```
在上述代码中,我们使用了`regularizers`库来定义L1稀疏约束和L2权重衰减。我们在编码层中使用这两个约束项,其中L1稀疏约束的系数为`10e-5`,L2权重衰减的系数为`0.001`。然后我们定义了一个自定义的损失函数`custom_loss`,该损失函数包含了MSE损失和L1稀疏约束项。注意在L1稀疏约束项的系数上,我们使用了一个较小的值`0.01`,以便更好地平衡MSE损失和稀疏约束项的贡献。
最后,我们使用`autoencoder.compile`方法来编译自编码器模型,并将自定义的损失函数作为参数传递给它。
阅读全文
相关推荐
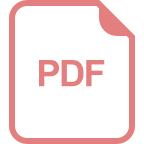


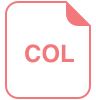
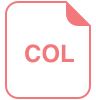
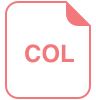
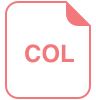
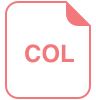
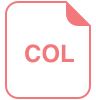
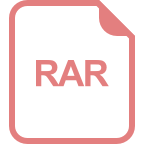
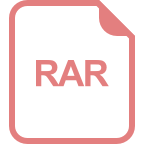
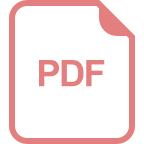