super(myYOLO, self).__init__() self.device = device self.num_classes = num_classes self.trainable = trainable self.conf_thresh = conf_thresh self.nms_thresh = nms_thresh self.stride = 32 self.grid_cell = self.create_grid(input_size) self.input_size = input_size self.scale = np.array([[[input_size[1], input_size[0], input_size[1], input_size[0]]]]) self.scale_torch = torch.tensor(self.scale.copy(), device=device).float()解释代码
时间: 2024-04-21 20:24:53 浏览: 166
这段代码是myYOLO类的构造函数(`__init__`方法)中的一部分,用于初始化myYOLO类的实例对象。下面是对代码逐行的解释:
1. `super(myYOLO, self).__init__()`: 这行代码调用了父类(nn.Module)的构造函数,以确保myYOLO类继承了nn.Module的属性和方法。
2. `self.device = device`: 将输入的`device`参数赋值给模块的`device`属性,用于指定模型在哪个设备上运行。
3. `self.num_classes = num_classes`: 将输入的`num_classes`参数赋值给模块的`num_classes`属性,表示目标类别的数量。
4. `self.trainable = trainable`: 将输入的`trainable`参数赋值给模块的`trainable`属性,表示模型参数是否可训练。
5. `self.conf_thresh = conf_thresh`: 将输入的`conf_thresh`参数赋值给模块的`conf_thresh`属性,表示目标置信度阈值。
6. `self.nms_thresh = nms_thresh`: 将输入的`nms_thresh`参数赋值给模块的`nms_thresh`属性,表示非极大值抑制(NMS)的阈值。
7. `self.stride = 32`: 设置模型的步长(stride)为32,表示每个网格单元(cell)在输入图像上的相对尺寸。
8. `self.grid_cell = self.create_grid(input_size)`: 调用`create_grid`方法创建网格单元,该方法的作用是在输入图像上创建网格,并返回网格单元的坐标。
9. `self.input_size = input_size`: 将输入的`input_size`参数赋值给模块的`input_size`属性,表示输入图像的尺寸。
10. `self.scale = np.array([[[input_size[1], input_size[0], input_size[1], input_size[0]]]])`: 创建一个形状为(1, 1, 4)的NumPy数组,表示尺度缩放因子。这个数组用于将预测的边界框坐标转换为相对于输入图像的绝对坐标。
11. `self.scale_torch = torch.tensor(self.scale.copy(), device=device).float()`: 将`self.scale`数组复制一份并转换为PyTorch张量,赋值给模块的`scale_torch`属性。这将确保缩放因子张量与设备匹配,并将其数据类型设置为float。
这段代码的作用是在myYOLO类中初始化各种属性,并对一些需要预先计算或初始化的变量进行设置。这些属性和变量将在后续的模型计算中使用。
阅读全文
相关推荐
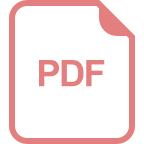
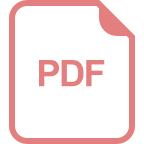
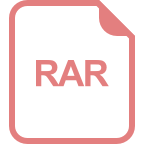















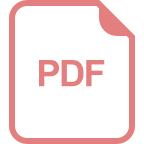