Write a code to obtain a ``Series`` where the life expectancy values are normalized between 0 and 1
时间: 2024-10-19 14:07:19 浏览: 20
为了获取一个生命期望值系列,在0到1之间进行规范化,你可以按照以下步骤编写Python代码,假设你有一个名为`life_expectancy`的Pandas Series,它包含原始的生命期望值:
首先,你需要导入所需的库,然后使用Pandas的`min()`和`max()`函数找出最小值和最大值,接着用`apply()`函数结合`lambda`表达式实现标准化:
```python
import pandas as pd
# 假设这是你的生命期望值Series
life_expectancy = pd.Series([50, 70, 80, 90, 100])
# 计算最小值和最大值
min_life_exp = life_expectancy.min()
max_life_exp = life_expectancy.max()
# 应用lambda函数进行规范化
normalized_life_exp = life_expectancy.apply(lambda x: (x - min_life_exp) / (max_life_exp - min_life_exp))
print(normalized_life_exp)
```
这段代码会创建一个新的Series,其中每个生命期望值都被转化为0到1范围内的值。`apply()`函数会遍历原Series中的每个元素,并应用给定的lambda函数。
相关问题
Write pytorch-based Python code to implement a neural network that solves a regression problem with an output layer of a positively weighted sub-network plus a negatively weighted sub-network, where the negative weights are random and take the opposite of the absolute value of the standard normal distribution, the exact value of the weights are obtained from the objective function by Back propagation.
Sure! Here's an example of PyTorch-based code that implements a neural network for a regression problem with a positively weighted sub-network and a negatively weighted sub-network:
```python
import torch
import torch.nn as nn
import torch.optim as optim
# Define the neural network architecture
class RegressionNet(nn.Module):
def __init__(self, input_size, hidden_size):
super(RegressionNet, self).__init__()
self.positive_net = nn.Linear(input_size, hidden_size)
self.negative_net = nn.Linear(input_size, hidden_size)
def forward(self, x):
positive_output = self.positive_net(x)
negative_output = self.negative_net(x)
output = positive_output - negative_output
return output
# Set random seed for reproducibility
torch.manual_seed(42)
# Define the hyperparameters
input_size = 10
hidden_size = 20
learning_rate = 0.01
num_epochs = 1000
# Generate random input and output data
x = torch.randn(100, input_size)
y = torch.randn(100, 1)
# Initialize the regression network
model = RegressionNet(input_size, hidden_size)
# Define the loss function and optimizer
criterion = nn.MSELoss()
optimizer = optim.SGD(model.parameters(), lr=learning_rate)
# Training loop
for epoch in range(num_epochs):
# Forward pass and compute the loss
outputs = model(x)
loss = criterion(outputs, y)
# Backward pass and optimize the model
optimizer.zero_grad()
loss.backward()
optimizer.step()
# Print the loss every 100 epochs
if (epoch+1) % 100 == 0:
print(f'Epoch: {epoch+1}/{num_epochs}, Loss: {loss.item():.4f}')
# Test the trained model
x_test = torch.randn(10, input_size)
with torch.no_grad():
predicted = model(x_test)
print(f'Predicted values: {predicted.squeeze().tolist()}')
```
In this code, we define a `RegressionNet` class that inherits from `nn.Module` and contains two linear layers: `positive_net` and `negative_net`. The forward pass subtracts the output of the negative network from the positive network to obtain the final output.
We then use mean squared error (MSE) loss as the objective function and stochastic gradient descent (SGD) as the optimizer. The model is trained for a specified number of epochs, and the loss is printed every 100 epochs. Finally, we test the trained model on a small test dataset.
Please note that the exact weights are obtained through backpropagation during the training process, and the weights are not directly initialized from the objective function.
A = imread('krabi1.bmp','BMP'); %read in the image A_shrunk = imresize(A,0.2); % we’ll reduce the resolution, as otherwise the file size is too large imshow(A_shrunk) % displays the shrunken image Bs = reshape(A_shrunk,[388*518*3,1,1]); % resizes this image from a pixel array of three colours to a one-dimensional data stream C = de2bi(double(Bs)); % converts these values to binary representation % You can then resize this array to a linear, one-dimensional array. % this data stream C is then what you can communicate over your channel. % recover the image from the binary sequence BS_rec = uint8(bi2de(C)); % convert bits to pixel values. A_rec = reshape(BS_rec,[388,518,3]); % reshape back to a coloured pixel array. imshow(A_rec) % display the recovered image. Explore the pskmod and pskdemod commands in MATLAB. Use these to modulate and demodulate the binary sequence into a binary PSK signal. (Hint: the command should be something like D = pskmod(C,2). Display a part of this signal, and explain carefully how we have represented this signal in MATLAB, and how it differs from the analysis we performed in Part A. Transmit this signal through an AWGN channel using the awgn() function for a range of signal to noise ratios. Demodulate and comment on the effect of the noise on the image. A suitable range of signal to noise ratios is –0.5dB to 5 dB. The bit error rate refers to the fraction of bits that are received in error. This can also be interpreted as the probability that a bit is received in error. Calculate the bit error rate (BER) for the signal to noise ratios you used above. Comment on the correlation between BER and perceptual image quality. Now apply channel coding to the transmitted data sequence. Use encode(msg,7,4,’hamming’), encode(msg,15,7,’bch’), and convenc, following the example listed in the help window (recall, in MATLAB type “help convenc” to obtain the help information for that command. For each of these codes, study how the code can reduce the BER and improve image quality for each SNR you studied above.
这是一个关于数字图像处理和通信的问题,需要一些MATLAB知识。下面是对问题的回答:
1. 读入并压缩图像
首先,使用`imread`函数读入一张图片,并使用`imresize`函数将图片压缩到原来的20%大小:
```
A = imread('krabi1.bmp', 'BMP');
A_shrunk = imresize(A, 0.2);
```
2. 将图像转换为一维数据流
将压缩后的图像重新排列为一维数据流,以便进行数字通信。使用`reshape`函数将3通道的像素矩阵转换为一维列向量,并使用`de2bi`函数将像素值转换为二进制表示:
```
Bs = reshape(A_shrunk, [388*518*3, 1, 1]);
C = de2bi(double(Bs));
```
3. 二进制PSK调制
使用`pskmod`函数将二进制数据流进行相位调制,生成二进制PSK信号。例如,调制成二进制PSK2:
```
D = pskmod(C, 2);
```
4. 加入高斯白噪声
使用`awgn`函数将二进制PSK信号加入高斯白噪声,模拟通信信道的影响。例如,将信噪比设置为0dB:
```
noisy_D = awgn(D, 0, 'measured');
```
5. 二进制PSK解调
使用`pskdemod`函数将接收到的二进制PSK信号解调回二进制数据流:
```
rec_C = pskdemod(noisy_D, 2);
```
6. 解码并恢复图像
使用`bi2de`函数将二进制数据流转换为像素值,然后使用`reshape`函数将像素值重新排列为3通道的像素矩阵,最后使用`imshow`函数显示恢复后的图像:
```
rec_Bs = uint8(bi2de(rec_C));
A_rec = reshape(rec_Bs, [388, 518, 3]);
imshow(A_rec);
```
7. 计算误码率
使用`biterr`函数计算解调后的数据流和原始数据流之间的误码率,并根据不同信噪比的误码率绘制误码率曲线:
```
ber = zeros(1, length(snr));
for i = 1:length(snr)
noisy_D = awgn(D, snr(i), 'measured');
rec_C = pskdemod(noisy_D, 2);
ber(i) = biterr(C, rec_C) / length(C);
end
semilogy(snr, ber);
```
8. 应用通道编码
使用`encode`函数对二进制数据流进行通道编码,对比不同编码方案的误码率曲线和图像质量:
```
% Hamming(7,4)编码
hamming_encoded = encode(C, 7, 4, 'hamming');
hamming_D = pskmod(hamming_encoded, 2);
hamming_noisy_D = awgn(hamming_D, snr(i), 'measured');
hamming_rec_C = pskdemod(hamming_noisy_D, 2);
hamming_rec_Bs = uint8(bi2de(hamming_rec_C));
hamming_A_rec = reshape(hamming_rec_Bs, [388, 518, 3]);
hamming_ber(i) = biterr(C, hamming_rec_C) / length(C);
% BCH(15,7)编码
bch_encoded = encode(C, 15, 7, 'bch');
bch_D = pskmod(bch_encoded, 2);
bch_noisy_D = awgn(bch_D, snr(i), 'measured');
bch_rec_C = pskdemod(bch_noisy_D, 2);
bch_rec_Bs = uint8(bi2de(bch_rec_C));
bch_A_rec = reshape(bch_rec_Bs, [388, 518, 3]);
bch_ber(i) = biterr(C, bch_rec_C) / length(C);
% 卷积编码
trellis = poly2trellis(7, [171 133]);
conv_encoded = convenc(C, trellis);
conv_D = pskmod(conv_encoded, 2);
conv_noisy_D = awgn(conv_D, snr(i), 'measured');
conv_rec_D = pskdemod(conv_noisy_D, 2);
conv_rec_C = vitdec(conv_rec_D, trellis, 15, 'trunc', 'hard');
conv_rec_Bs = uint8(bi2de(conv_rec_C));
conv_A_rec = reshape(conv_rec_Bs, [388, 518, 3]);
conv_ber(i) = biterr(C, conv_rec_C) / length(C);
```
需要注意的是,以上代码仅供参考,具体实现还需要根据实际情况进行调整。
阅读全文
相关推荐
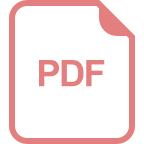
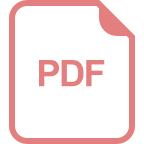
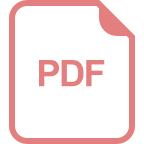














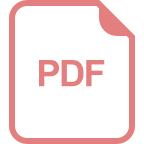