ModuleNotFoundError: No module named 'global_state'
时间: 2023-08-18 16:14:52 浏览: 332
这个错误通常出现在你的代码中尝试导入一个名为 'global_state' 的模块,但是该模块在你的环境中并不存在。请确保你已经安装了该模块,并且使用正确的名称进行导入。
如果你使用的是 Python,你可以尝试使用以下命令安装该模块:
```
pip install global_state
```
如果你使用的是其他语言或开发环境,请参考相应的文档来安装所需的模块。
另外,如果你是在某个项目中遇到了这个错误,并且该项目依赖于其他模块,请确保你已经安装了所有依赖项,并且它们的名称正确。
相关问题
填写以下代码,并给出详细解释Hypothesis = namedtuple('Hypothesis', ['value', 'score']) class NMT(nn.Module): """ Simple Neural Machine Translation Model: - Bidrectional LSTM Encoder - Unidirection LSTM Decoder - Global Attention Model (Luon
The code you provided defines a named tuple `Hypothesis` with two fields, `value` and `score`. This is a convenient way to store and manipulate hypotheses in the context of sequence-to-sequence models.
The `NMT` class is a PyTorch module that implements a simple neural machine translation model. It consists of a bidirectional LSTM encoder, a unidirectional LSTM decoder, and a global attention mechanism based on Luong et al. (2015).
Here's a breakdown of the code:
```python
from collections import namedtuple
import torch
import torch.nn as nn
import torch.nn.functional as F
Hypothesis = namedtuple('Hypothesis', ['value', 'score'])
class NMT(nn.Module):
def __init__(self, src_vocab_size, tgt_vocab_size, emb_size, hidden_size):
super(NMT, self).__init__()
self.src_embed = nn.Embedding(src_vocab_size, emb_size)
self.tgt_embed = nn.Embedding(tgt_vocab_size, emb_size)
self.encoder = nn.LSTM(emb_size, hidden_size, bidirectional=True)
self.decoder = nn.LSTMCell(emb_size + hidden_size, hidden_size)
self.attention = nn.Linear(hidden_size * 2, hidden_size)
self.out = nn.Linear(hidden_size, tgt_vocab_size)
self.hidden_size = hidden_size
def forward(self, src, tgt):
batch_size = src.size(0)
src_len = src.size(1)
tgt_len = tgt.size(1)
# Encode the source sentence
src_embedded = self.src_embed(src)
encoder_outputs, (last_hidden, last_cell) = self.encoder(src_embedded)
# Initialize the decoder states
decoder_hidden = last_hidden.view(batch_size, self.hidden_size)
decoder_cell = last_cell.view(batch_size, self.hidden_size)
# Initialize the attention context vector
context = torch.zeros(batch_size, self.hidden_size, device=src.device)
# Initialize the output scores
outputs = torch.zeros(batch_size, tgt_len, self.hidden_size, device=src.device)
# Decode the target sentence
for t in range(tgt_len):
tgt_embedded = self.tgt_embed(tgt[:, t])
decoder_input = torch.cat([tgt_embedded, context], dim=1)
decoder_hidden, decoder_cell = self.decoder(decoder_input, (decoder_hidden, decoder_cell))
attention_scores = self.attention(encoder_outputs)
attention_weights = F.softmax(torch.bmm(attention_scores, decoder_hidden.unsqueeze(2)).squeeze(2), dim=1)
context = torch.bmm(attention_weights.unsqueeze(1), encoder_outputs).squeeze(1)
output = self.out(decoder_hidden)
outputs[:, t] = output
return outputs
```
The `__init__` method initializes the model parameters and layers. It takes four arguments:
- `src_vocab_size`: the size of the source vocabulary
- `tgt_vocab_size`: the size of the target vocabulary
- `emb_size`: the size of the word embeddings
- `hidden_size`: the size of the encoder and decoder hidden states
The model has four main components:
- `src_embed`: an embedding layer for the source sentence
- `tgt_embed`: an embedding layer for the target sentence
- `encoder`: a bidirectional LSTM encoder that encodes the source sentence
- `decoder`: a unidirectional LSTM decoder that generates the target sentence
The attention mechanism is implemented in the `forward` method. It takes two arguments:
- `src`: the source sentence tensor of shape `(batch_size, src_len)`
- `tgt`: the target sentence tensor of shape `(batch_size, tgt_len)`
The method first encodes the source sentence using the bidirectional LSTM encoder. The encoder outputs and final hidden and cell states are stored in `encoder_outputs`, `last_hidden`, and `last_cell`, respectively.
The decoder is initialized with the final hidden and cell states of the encoder. At each time step, the decoder takes as input the embedded target word and the context vector, which is a weighted sum of the encoder outputs based on the attention scores. The decoder output and hidden and cell states are updated using the LSTMCell module.
The attention scores are calculated by applying a linear transform to the concatenated decoder hidden state and encoder outputs, followed by a softmax activation. The attention weights are used to compute the context vector as a weighted sum of the encoder outputs.
Finally, the decoder hidden state is passed through a linear layer to produce the output scores for each target word in the sequence. The output scores are stored in the `outputs` tensor and returned by the method.
编写pytorch代码,定义适应度函数,要求:利用训练集的预测误差当作每个粒子的适应度值,根据粒子的适应度值,选择最优的粒子作为当前的全局最优解,以及每个粒子自身的局部最优解,根据全局最优解和局部最优解,更新每个粒子的速度和位置,使用更新后的参数来更新LSTM模型的权重和偏置,计算更新后的LSTM模型在训练集上的误差,并更新全局最优解和局部最优解,根据收敛条件是否满足,决定是否继续迭代,如果迭代次数达到预设值或误差已经足够小,则停止迭代,并返回最优的LSTM模型参数
以下是一个简单的示例代码,实现了一个适应度函数,使用训练集的预测误差作为每个粒子的适应度值,并根据粒子的适应度值选择最优的粒子作为当前的全局最优解,以及每个粒子自身的局部最优解,更新每个粒子的速度和位置,使用更新后的参数来更新LSTM模型的权重和偏置,计算更新后的LSTM模型在训练集上的误差,并更新全局最优解和局部最优解,根据收敛条件是否满足,决定是否继续迭代,如果迭代次数达到预设值或误差已经足够小,则停止迭代,并返回最优的LSTM模型参数。
```python
import torch
import torch.nn as nn
import numpy as np
# 定义LSTMAttention模型
class LSTMAttention(nn.Module):
def __init__(self, input_dim, hidden_dim, output_dim):
super(LSTMAttention, self).__init__()
self.lstm = nn.LSTM(input_dim, hidden_dim, batch_first=True)
self.attention = nn.Linear(hidden_dim, 1)
self.fc = nn.Linear(hidden_dim, output_dim)
def forward(self, x):
output, (h_n, c_n) = self.lstm(x)
attention_weights = torch.softmax(self.attention(output), dim=1)
context_vector = torch.sum(attention_weights * output, dim=1)
output = self.fc(context_vector)
return output
# 定义适应度函数
def fitness_function(model, train_loader):
criterion = nn.MSELoss()
model.eval()
with torch.no_grad():
total_loss = 0.0
for inputs, targets in train_loader:
outputs = model(inputs)
loss = criterion(outputs, targets)
total_loss += loss.item()
avg_loss = total_loss / len(train_loader)
return avg_loss
# 定义PSO算法
def pso(model, train_loader, num_particles, inertia_weight, cognitive_weight, social_weight, max_iterations, convergence_tolerance):
# 初始化粒子群
particle_positions = []
particle_velocities = []
particle_best_positions = []
particle_best_fitnesses = []
global_best_position = None
global_best_fitness = float('inf')
for i in range(num_particles):
particle_position = model.state_dict()
particle_positions.append(particle_position)
particle_velocity = {}
for name, param in model.named_parameters():
velocity = torch.randn(param.shape)
particle_velocity[name] = velocity
particle_velocities.append(particle_velocity)
particle_best_positions.append(particle_position)
particle_best_fitnesses.append(float('inf'))
# 迭代优化
for iter in range(max_iterations):
for i in range(num_particles):
# 更新粒子速度和位置
for name, velocity in particle_velocities[i].items():
rp = np.random.rand(*velocity.shape)
rg = np.random.rand(*velocity.shape)
particle_velocity[name] = (inertia_weight * velocity
+ cognitive_weight * rp * (particle_best_positions[i][name] - particle_positions[i][name])
+ social_weight * rg * (global_best_position[name] - particle_positions[i][name]))
particle_positions[i][name] += particle_velocity[name]
# 更新模型参数
model.load_state_dict(particle_positions[i])
# 计算适应度值
fitness = fitness_function(model, train_loader)
# 更新局部最优解
if fitness < particle_best_fitnesses[i]:
particle_best_positions[i] = particle_positions[i]
particle_best_fitnesses[i] = fitness
# 更新全局最优解
if fitness < global_best_fitness:
global_best_position = particle_positions[i]
global_best_fitness = fitness
# 判断是否收敛
if global_best_fitness < convergence_tolerance:
break
# 返回最优的LSTM模型参数
return global_best_position
```
需要注意的是,以上代码仅为示例代码,实际使用时需要根据具体问题进行一些调整和改进。
阅读全文
相关推荐
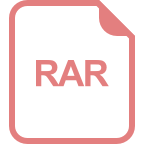
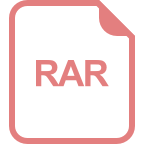
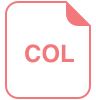
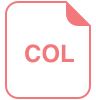
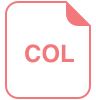
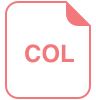
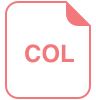
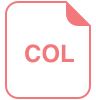
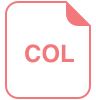
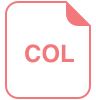
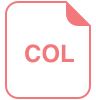
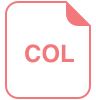
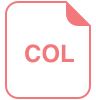
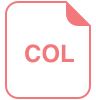
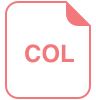
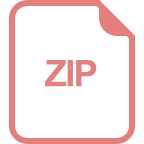
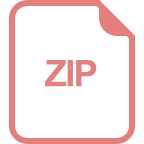