季节性ARIMA模型公式
时间: 2023-11-13 19:03:36 浏览: 109
季节性ARIMA模型是一种用于时间序列数据分析和预测的模型。它综合了自回归(AR)、差分(I)和移动平均(MA)这三种模型的特点,并考虑了季节性因素。
ARIMA模型的一般形式是ARIMA(p, d, q)(P, D, Q)s,其中:
- p表示自回归阶数,代表过去p个时间点的观测值对当前值的影响;
- d表示差分阶数,代表进行了d次差分以使时间序列平稳;
- q表示移动平均阶数,代表过去q个误差对当前值的影响;- P表示季节性自回归阶数,代表过去P个季节性时间点的观测值对当前值的影响;
- D表示季节性差分阶数,代表进行了D次季节性差分以使时间序列平稳;
- Q表示季节性移动平均阶数,代表过去Q个季节性误差对当前值的影响;
- s表示季节性周期长度。
具体地,季节性ARIMA模型的公式可以表示为:
(1 - φ₁B - φ₂B² - ... - φₚBᵖ)(1 - Φ₁Bˢ - Φ₂B²ˢ - ... - ΦₚBᵖˢ)(1 - B)ᵈ(1 - Bˢ)ᴰyt = (1 + θ₁B + θ₂B² + ... + θₚBᵖ)(1 + Θ₁Bˢ + Θ₂B²ˢ + ... + ΘₚBᵖˢ)εt
其中,B是滞后算子,yt表示观测值,εt表示误差项。φ₁, φ₂, ..., φₚ, Φ₁, Φ₂, ..., Φₚ, θ₁, θ₂, ..., θₚ, Θ₁, Θ₂, ..., Θₚ是模型的参数,需要通过拟合数据来估计。
需要注意的是,季节性ARIMA模型的参数估计和模型选择是一个复杂的过程,需要结合实际数据和统计方法进行。
相关问题
季节性ARIMA模型求解
季节性ARIMA模型求解是基于时间序列数据的一种模型,用于预测具有季节性影响的数据。ARIMA模型是由AR(自回归)、I(差分)和MA(移动平均)三个部分组成的。对于季节性ARIMA模型,还需要考虑季节性的影响。
首先,我们需要通过观察数据来确定模型的阶数。对于季节性ARIMA模型,我们需要确定AR、I、MA和季节性AR、季节性I、季节性MA的阶数。
接下来,我们需要对数据进行季节性差分。这意味着我们要对数据进行一个周期为季节长度的差分。例如,对于月度数据,我们可以对数据进行12阶差分。
然后,我们可以使用拟合数据的方法来估计模型的参数。这可以通过最小化模型的残差平方和来实现,常用的方法是最小二乘法。
一旦我们得到了模型的参数,我们就可以使用该模型来进行预测。预测可以通过迭代使用模型的公式来实现。
最后,我们可以评估模型的准确性。常用的评估方法包括均方根误差(RMSE)和平均绝对百分比误差(MAPE)等。
总结起来,季节性ARIMA模型的求解包括确定模型的阶数、进行季节性差分、估计参数、进行预测和评估模型的准确性。这个过程可以帮助我们理解和预测具有季节性影响的时间序列数据。
季节性ARIMA时间序列数学公式
季节性ARIMA(Seasonal ARIMA)时间序列模型是一种用来分析具有季节性变化的时间序列数据的方法。它是建立在ARIMA(Autoregressive Integrated Moving Average)模型的基础上,通过引入季节性差分和季节性自回归和移动平均项来对季节性数据进行建模。
季节性ARIMA模型的数学公式可以表示为:
ARIMA(p, d, q)(P, D, Q)m
其中,p、d、q是非季节性部分的阶数,P、D、Q是季节性部分的阶数,m是季节性周期。
ARIMA模型的非季节性部分表示为:
(1 - φ₁B - φ₂B² - ... - φₚBᵖ)(1 - B)ᵈXₜ = (1 + θ₁B + θ₂B² + ... + θₚBᵖ)aₜ
其中,φ₁、φ₂、...、φₚ是非季节性自回归系数,θ₁、θ₂、...、θₚ是非季节性移动平均系数,B是滞后算子,d是差分阶数,Xₜ是观测值,aₜ是白噪声。
ARIMA模型的季节性部分表示为:
(1 - Φ₁Bᵐ - Φ₂B²ᵐ - ... - ΦₚBᵐP)(1 - Bᵐ)ᴰ(1 - B)ᵈXₜ = (1 + Θ₁Bᵐ + Θ₂B²ᵐ + ... + ΘₚBᵐP)aₜ
其中,Φ₁、Φ₂、...、Φₚ是季节性自回归系数,Θ₁、Θ₂、...、Θₚ是季节性移动平均系数,P是季节性周期,D是季节性差分阶数。
通过对ARIMA模型的参数估计和模型检验,可以得到对季节性时间序列数据进行预测和分析的结果。
相关推荐
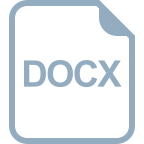
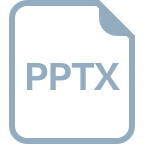
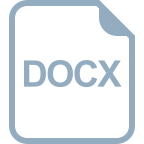











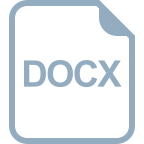
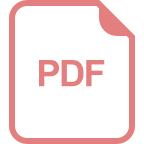