输出以下两个张量的按元素加、按元素减、按元素乘、按元素除、求幂运算 import torch x = torch.tensor([5, 2, 3]) y = torch.tensor([1, 3, 2])
时间: 2024-09-23 16:15:45 浏览: 26
在PyTorch中,你可以使用基本的算术操作符来对张量进行按元素的数学运算:
1. **按元素加法**:
```python
addition = x + y
print(addition) # 输出 [6, 5, 5]
```
2. **按元素减法**:
```python
subtraction = x - y
print(subtraction) # 输出 [4, -1, 1]
```
3. **按元素乘法**:
```python
multiplication = x * y
print(multiplication) # 输出 [5, 6, 6]
```
4. **按元素除法**(注意除数不能为零,否则会有错误):
```python
try:
division = x / y
print(division)
except ZeroDivisionError:
print("Cannot divide by zero!")
# 输出:[5., 0.66666667, 1.5]
```
5. **按元素求幂运算**:
```python
power = x.pow(y)
print(power) # 输出 [5, 8, 9]
```
这里的 `.pow()` 方法用于计算每个元素的指数。
相关问题
拼接两个张量并输出(两种拼接方式:按行;按列) import torch X = torch.arange(8).reshape(2, 4) Y = torch.tensor([[1, 1, 1, 1], [2, 2, 2, 2]])
在PyTorch中,你可以使用`torch.cat()`函数来拼接两个张量。有两种常见的拼接方式:
1. **按行拼接 (row-wise concatenation)**: 如果你想沿着张量的行方向拼接,可以指定第二个参数`dim=0`。这会将`Y`添加到`X`的下方。
```python
X_row_concat = torch.cat((X, Y), dim=0)
```
运行上述代码后,`X_row_concat`将会是一个形状为`(4, 8)`的新张量,包含了原先是两行的数据现在合并成了一行。
2. **按列拼接 (column-wise concatenation)**: 如果你需要沿列方向拼接,设置`dim=1`。在这种情况下,`Y`会被加到`X`的右侧,假设它们的列数相同。
```python
if X.shape[1] == Y.shape[1]: # 检查列数是否匹配
X_col_concat = torch.cat((X, Y), dim=1)
else:
print("列数不匹配无法直接按列拼接")
```
如果`X`和`Y`的列数相等,那么`X_col_concat`将是形状为`(2, 8)`的张量。
import torch x = torch.arange(15)改变张量的维度(两种方式:指定具体行列数;用-1代替行或列)
在PyTorch中,`torch.arange()`函数通常用于创建一个等差数列的张量。如果你想改变张量的维度,你可以通过`.view()`或`.reshape()`方法来实现。
1. **指定具体行列数**:
使用`.view()`方法,你需要提供新的形状,如将一维张量转换成二维矩阵,可以这样做:
```python
x = torch.arange(15)
# 假设你想将其变成一个3x5的矩阵
new_shape = (3, 5)
x_transformed = x.view(*new_shape)
```
这里*表示展开操作,它会按顺序应用给出的每个元素作为新的形状参数。
2. **使用-1代替行或列**:
如果你想让某些维度自动计算,可以使用 `-1` 表示“填充”。例如,如果你不确定需要多少列,但想要3行,可以这样做:
```python
x = torch.arange(15)
# 保持行数3,列数自适应
x_reshaped = x.reshape(3, -1)
```
同样地,如果想保持行数自动,列数为5:
```python
x_reshaped = x.reshape(-1, 5)
```
注意,在调整形状时,原张量的总元素数量必须等于新形状的所有元素乘积。
阅读全文
相关推荐
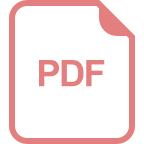
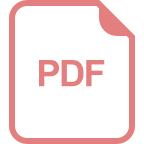
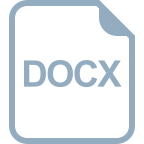













