sklearn贝叶斯分类模型的可视化
时间: 2023-07-12 22:11:26 浏览: 169
sklearn中贝叶斯分类模型的可视化可以通过使用matplotlib库来实现。
以高斯朴素贝叶斯分类器(GaussianNB)为例,可以使用以下代码来可视化分类结果:
```python
import numpy as np
import matplotlib.pyplot as plt
from sklearn.naive_bayes import GaussianNB
from sklearn.datasets import make_classification
from sklearn.model_selection import train_test_split
from sklearn.metrics import accuracy_score
# 生成模拟数据集
X, y = make_classification(n_samples=1000, n_features=2, n_informative=2, n_redundant=0, random_state=42)
# 划分训练集和测试集
X_train, X_test, y_train, y_test = train_test_split(X, y, test_size=0.3, random_state=42)
# 创建高斯朴素贝叶斯分类器
gnb = GaussianNB()
# 拟合训练集
gnb.fit(X_train, y_train)
# 预测测试集
y_pred = gnb.predict(X_test)
# 计算准确率
accuracy = accuracy_score(y_test, y_pred)
print("Accuracy:", accuracy)
# 可视化分类结果
x_min, x_max = X[:, 0].min() - 1, X[:, 0].max() + 1
y_min, y_max = X[:, 1].min() - 1, X[:, 1].max() + 1
xx, yy = np.meshgrid(np.arange(x_min, x_max, 0.1), np.arange(y_min, y_max, 0.1))
Z = gnb.predict(np.c_[xx.ravel(), yy.ravel()])
Z = Z.reshape(xx.shape)
plt.contourf(xx, yy, Z, alpha=0.4)
plt.scatter(X[:, 0], X[:, 1], c=y, alpha=0.8)
plt.title("GaussianNB Classification")
plt.xlabel("Feature 1")
plt.ylabel("Feature 2")
plt.show()
```
在上述代码中,首先使用make_classification函数生成一个二维的模拟数据集,然后将其划分为训练集和测试集。接着创建高斯朴素贝叶斯分类器,并拟合训练集。使用预测函数predict对测试集进行预测,并计算准确率。最后,使用meshgrid和contourf函数可视化分类结果,使用scatter函数绘制数据点。
阅读全文
相关推荐
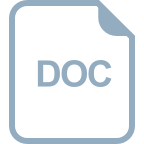
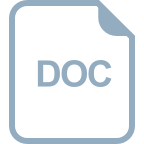
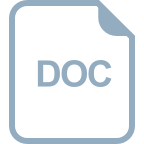

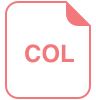






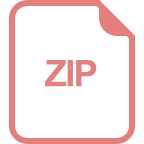
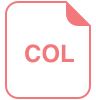





