python 计算图像分割后的miou
时间: 2023-08-05 08:05:10 浏览: 163
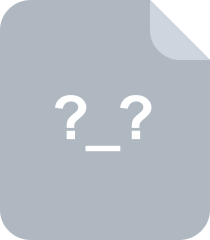
计算 语义分割结果 MIOU,miou.py操作简单,输出结果清晰
计算图像分割后的Mean Intersection over Union (mIoU) 可以使用以下步骤进行:
1. 首先,需要获取原始图像和分割结果图像。可以使用Python的图像处理库(如PIL或OpenCV)读取图像,或者使用现有的数据集加载器获取图像和标签。
2. 将原始图像和分割结果图像转换为numpy数组,以便进行后续计算。你可以使用库函数或自定义函数来完成这个步骤。
3. 计算每个类别的Intersection over Union (IoU)。对于每个类别,需要计算该类别在原始图像和分割结果图像中的交集和并集。交集是指在两个图像中都被标记为该类别的像素数量,而并集是指在原始图像和分割结果图像中被标记为该类别的像素总数量。然后,将交集除以并集得到该类别的IoU。
4. 对所有类别的IoU进行求平均,得到mIoU。可以简单地将所有类别的IoU相加,然后除以类别数量,或者根据像素数量加权求平均。
以下是一个示例代码,展示了如何计算图像分割后的mIoU:
```python
import numpy as np
def calculate_iou(segmentation, label):
intersection = np.logical_and(segmentation, label)
union = np.logical_or(segmentation, label)
iou = np.sum(intersection) / np.sum(union)
return iou
def calculate_miou(segmentation_images, label_images):
num_classes = segmentation_images.shape[3]
miou = 0
for class_idx in range(num_classes):
segmentation = segmentation_images[..., class_idx]
label = label_images[..., class_idx]
iou = calculate_iou(segmentation, label)
miou += iou
miou /= num_classes
return miou
# 示例使用
segmentation_images = np.random.randint(0, 2, size=(100, 100, 3, 5)) # 假设有5个类别,每个图像大小为100x100
label_images = np.random.randint(0, 2, size=(100, 100, 3, 5))
miou = calculate_miou(segmentation_images, label_images)
print("mIoU:", miou)
```
请注意,这只是一个简单的示例代码,实际应用中可能需要根据具体的需求进行修改和优化。
阅读全文
相关推荐


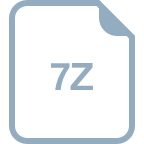
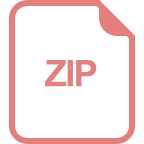
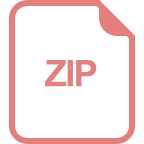
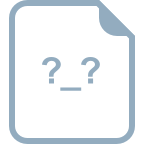
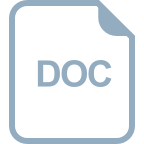
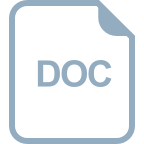
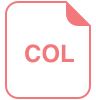
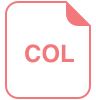
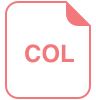
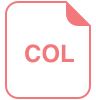



