nsga-ii多目标优化python
时间: 2024-08-06 21:01:30 浏览: 107
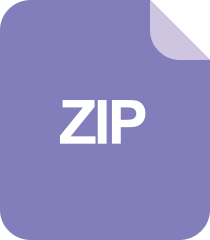
NSGA-II.zip_NSGA_NSGA-II_hunterngn_python_python nsga

NSGA-II(Non-dominated Sorting Genetic Algorithm II)是一种常用的多目标优化算法,它属于进化计算家族,用于解决同时优化多个目标函数的问题,通常出现在工程设计、机器学习等领域。在Python中,你可以使用`pygmo`库来进行NSGA-II的实现,这个库提供了丰富的多目标优化算法支持。
NSGA-II的工作原理包括以下几个步骤:
1. 初始化种群:生成一组随机解作为初始个体。
2. 非支配排序:对种群中的个体进行排序,区分那些不能通过任一目标函数被其他个体超越的个体(称为Pareto最优解)。
3. 更新种群:选择、交叉和变异操作,保留非劣解,并且保证多样性,避免陷入局部最优。
4. 重复迭代:直到达到预定的迭代次数或者满足停止条件。
使用Python的示例代码可能会像这样:
```python
from pygmo import NSGA2
def fitness_function(individual):
# 这里需要替换为你的目标函数
return [objective_1(individual), objective_2(individual)]
# 创建NSGA2实例
solver = NSGA2(gen=100) # 指定迭代次数
# 运行优化
pop = solver.solve(fitness_function)
# 取出最优解
pareto_front = pop.get("Pareto front")
```
阅读全文
相关推荐
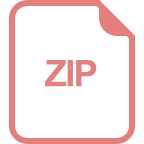


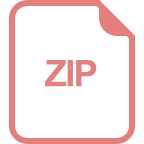
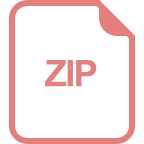
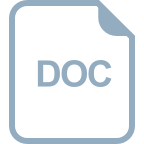
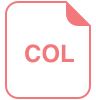
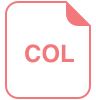
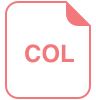
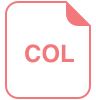
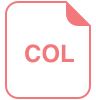
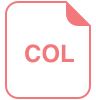
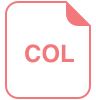
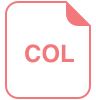
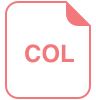
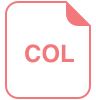