python代码用遗传算法求解车辆路径规划问题
时间: 2024-01-16 12:04:22 浏览: 21
车辆路径规划问题是一个经典的优化问题,遗传算法是一种常用的优化算法之一。下面是一个使用遗传算法求解车辆路径规划问题的 Python 代码示例:
``` python
import random
# 路径规划问题的目标函数,输入为一个路径(一个列表),输出为路径的总长度
def fitness(path):
# TODO: 根据具体问题实现目标函数
return total_distance
# 遗传算法的参数
POPULATION_SIZE = 100 # 种群大小
GENERATIONS = 1000 # 迭代次数
MUTATION_RATE = 0.01 # 变异率
# 生成初始种群
population = []
for i in range(POPULATION_SIZE):
path = [0] + random.sample(range(1, num_cities), num_cities-1) + [0] # 随机生成一条路径,起点和终点是 0
fitness_value = fitness(path) # 计算路径的总长度
population.append((path, fitness_value))
# 迭代搜索
for g in range(GENERATIONS):
# 选择操作,使用轮盘赌算法
fitness_values = [x[1] for x in population]
total_fitness = sum(fitness_values)
selection_probabilities = [x / total_fitness for x in fitness_values]
selected_population = random.choices(population, weights=selection_probabilities, k=POPULATION_SIZE)
# 交叉操作,使用顺序交叉算子
offspring_population = []
for i in range(0, POPULATION_SIZE, 2):
parent1, parent2 = selected_population[i], selected_population[i+1]
crossover_point = random.randint(1, num_cities-2)
child1 = parent1[0][:crossover_point] + [x for x in parent2[0] if x not in parent1[0][:crossover_point]] + parent1[0][crossover_point:]
child2 = parent2[0][:crossover_point] + [x for x in parent1[0] if x not in parent2[0][:crossover_point]] + parent2[0][crossover_point:]
offspring_population.append((child1, fitness(child1)))
offspring_population.append((child2, fitness(child2)))
# 变异操作,使用交换变异算子
for i in range(POPULATION_SIZE):
if random.random() < MUTATION_RATE:
path = offspring_population[i][0]
mutation_point1, mutation_point2 = random.sample(range(1, num_cities-1), 2)
path[mutation_point1], path[mutation_point2] = path[mutation_point2], path[mutation_point1]
offspring_population[i] = (path, fitness(path))
# 新一代种群由父代和子代组成,选择优秀的个体
population = sorted(selected_population + offspring_population, key=lambda x: x[1])[:POPULATION_SIZE]
```
在这个代码中,`fitness` 函数是路径规划问题的目标函数,用于计算给定路径的总长度。`POPULATION_SIZE`、`GENERATIONS` 和 `MUTATION_RATE` 是遗传算法的参数,可以根据具体问题进行调整。在代码中,使用顺序交叉算子和交换变异算子对个体进行交叉和变异操作。在每次迭代中,使用轮盘赌算法选择优秀的个体进行交叉和变异操作,然后将父代和子代合并,并选择优秀的个体作为新一代种群。
相关推荐
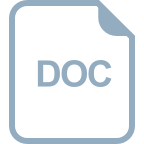
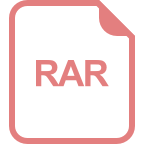
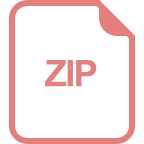
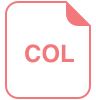
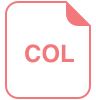
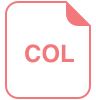
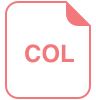
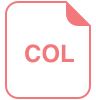









