import os def record_sample_indexes(test_folder, test_file_extension, original_test_file, index_folder): # 读取原测试集文件的内容 with open(original_test_file, 'r') as f: original_test_lines = f.readlines() # 遍历测试文件夹中的每个测试文件 for filename in os.listdir(test_folder): if filename.endswith(test_file_extension): test_file = os.path.join(test_folder, filename) index_file = os.path.join(index_folder, f"index{os.path.splitext(filename)[0]}.txt") # 读取当前测试文件的内容 with open(test_file, 'r') as f: test_lines = f.readlines() # 获取当前测试文件中每个样本在原测试集中的位置,并记录到索引文件中 with open(index_file, 'w') as f_index: for line in test_lines: line_parts = line.strip().split('\t') sample_id = int(line_parts[-1]) index = original_test_lines.index(f"{sample_id}\n") + 1 f_index.write(f"{index}\n") # 指定测试文件夹路径、测试文件扩展名、原测试集文件路径和索引文件夹路径 test_folder = 'exp1_test' test_file_extension = '.new' original_test_file = 'task1.test.new' index_folder = 'exp1_test_index' # 调用函数进行记录每个样本在原测试集中的位置 record_sample_indexes(test_folder, test_file_extension, original_test_file, index_folder)
时间: 2023-07-15 19:14:07 浏览: 71
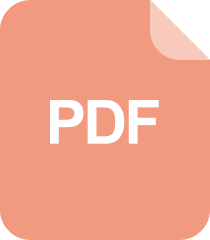
mysql创建Bitmap_Join_Indexes中的约束与索引
这段代码是一个 Python 函数,用于记录测试集中每个样本在原测试集中的位置,并将其写入索引文件中。具体实现为:
1. 首先读取原测试集文件的内容,保存在 `original_test_lines` 列表中。
2. 然后遍历测试文件夹中的每个测试文件,如果文件名以指定的测试文件扩展名结尾,则处理该文件。
3. 对于每个测试文件,读取其内容到 `test_lines` 列表中。
4. 遍历测试文件中的每一行,将其分割成若干部分,其中最后一部分是样本 ID。
5. 在原测试集中找到对应样本 ID 的行,并记录该行的位置(即行号)。
6. 将该位置写入索引文件中。
7. 最终得到的索引文件名为 `index{文件名}.txt`,文件内容是每个样本在原测试集中的位置。
代码中的参数有:
- `test_folder`: 测试文件夹路径
- `test_file_extension`: 测试文件扩展名
- `original_test_file`: 原测试集文件路径
- `index_folder`: 索引文件夹路径
调用该函数即可实现记录每个样本在原测试集中的位置。
阅读全文
相关推荐
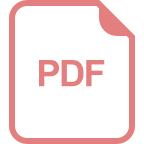
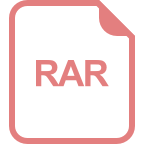
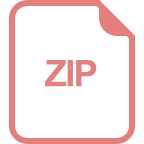















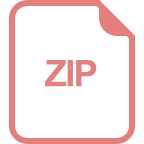