cv2.imwrite内存泄露
时间: 2023-10-26 22:25:38 浏览: 320
对于OpenCV的imwrite函数,在某些情况下可能会导致内存泄漏。内存泄漏是指在程序执行过程中,分配的内存空间没有被正确释放,导致内存占用增加,最终可能导致程序崩溃或性能下降。
要解决这个问题,你可以尝试以下几种方法:
1. 确保在每次调用imwrite函数后,释放相关的资源。可以使用cv2.destroyAllWindows()函数来关闭所有打开的窗口,或使用cv2.destroyWindow(window_name)函数来关闭特定名称的窗口。这样可以确保在每次写入图像后释放内存。
2. 如果你在循环中使用imwrite函数,确保在每次迭代结束后释放资源。可以使用cv2.waitKey(delay)函数来等待键盘输入,并确保在每次迭代结束后调用它。这样可以防止内存泄漏。
3. 如果你需要处理大量图像,可以考虑使用cv2.imencode()函数将图像编码为内存缓冲区,然后使用numpy数组来处理和保存图像。这样可以避免直接使用imwrite函数可能引起的内存泄漏问题。
总之,要解决cv2.imwrite函数可能导致的内存泄漏问题,你需要确保在每次写入图像后及时释放资源,或使用其他方法来处理和保存图像。如果问题仍然存在,请提供更多的代码和上下文信息,以便我们能够更好地帮助你解决问题。
相关问题
cv2.imwrite有哪些paras
cv2.imwrite是OpenCV库中用于保存图像的一个函数,它提供了几个参数,用于控制如何将图像保存到文件系统中。下面是cv2.imwrite函数的一些主要参数:
1. filename:必需的参数,表示要保存的图像文件的路径和文件名。支持的文件格式通常包括:JPEG, JPG, PNG, TIFF,等等。
2. img:必需的参数,表示要保存的图像数据,通常是通过cv2.imread读取或者通过其他图像处理函数生成的。
3. params:可选参数,用于指定保存图像的特定选项。例如:
- cv2.IMWRITE_JPEG_QUALITY:指定JPEG图像保存时的质量,范围为0到100,默认为95。
- cv2.IMWRITE_JPEG_PROGRESSIVE:用于指定是否保存为JPEG的渐进式格式。
- cv2.IMWRITE_PNG_COMPRESSION:指定PNG图像保存时的压缩级别,范围为0到9,默认值为3。
- cv2.IMWRITE_PNG_STRATEGY:指定PNG压缩策略,默认值为默认策略,用于平衡压缩速度和压缩效率。
使用cv2.imwrite函数时,可以通过一个列表将这些参数传递给函数,例如保存一个质量为95的JPEG图像可以写为:cv2.imwrite('image.jpg', img, [cv2.IMWRITE_JPEG_QUALITY, 95])
cv2.imwrite
`cv2.imwrite` 是 OpenCV 中用来保存图片的函数。它接受两个参数:第一个参数是保存图片的文件名(包括文件路径),第二个参数是要保存的图片数据。可以使用 `cv2.imread` 读取一张图片,然后使用 `cv2.imwrite` 保存这张图片。
示例:
```
import cv2
# Read an image
img = cv2.imread("example.jpg")
# Save the image
cv2.imwrite("example_output.jpg", img)
```
这里有一些可用的可选参数,比如可以使用第三个参数来指定图片压缩质量。默认质量为95。
```
cv2.imwrite("example_output.jpg", img, [cv2.IMWRITE_JPEG_QUALITY, 90])
```
还有可以用来指定要保存的图片的格式,比如PNG或者BMP格式。
```
cv2.imwrite("example_output.png", img)
```
阅读全文
相关推荐
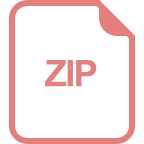
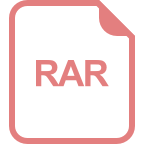
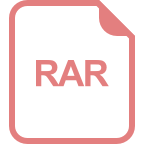





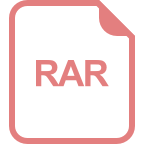
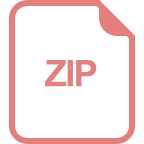
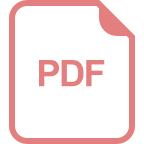
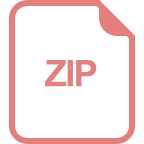
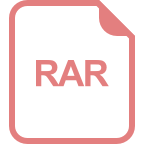
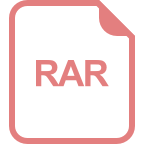
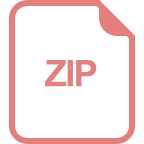