使用深度学习完成车牌检测代码
时间: 2024-02-03 17:03:19 浏览: 70
车牌检测是计算机视觉中的一个经典问题,使用深度学习可以极大地提高检测的准确性和效率。下面是一个简单的车牌检测代码,使用了OpenCV和TensorFlow进行车牌检测。
```python
import cv2
import numpy as np
import tensorflow as tf
# 读取模型文件
model = tf.keras.models.load_model('model.h5')
# 车牌检测函数
def detect_plate(img):
# 转换为灰度图像
gray = cv2.cvtColor(img, cv2.COLOR_BGR2GRAY)
# 高斯滤波
blur = cv2.GaussianBlur(gray, (5, 5), 0)
# Canny边缘检测
edges = cv2.Canny(blur, 100, 200)
# 膨胀操作,使边缘更加清晰
kernel = np.ones((3, 3), np.uint8)
dilate = cv2.dilate(edges, kernel, iterations=1)
# 查找轮廓
contours, _ = cv2.findContours(dilate, cv2.RETR_TREE, cv2.CHAIN_APPROX_SIMPLE)
# 遍历轮廓,找到最可能的车牌
max_area = 0
max_box = None
for cnt in contours:
area = cv2.contourArea(cnt)
if area > max_area:
x, y, w, h = cv2.boundingRect(cnt)
if w > h * 2 and w < h * 4:
max_area = area
max_box = (x, y, w, h)
if max_box is None:
return None
# 提取车牌图像
x, y, w, h = max_box
plate = img[y:y+h, x:x+w]
# 调整图像大小
plate = cv2.resize(plate, (224, 224))
# 归一化图像
plate = plate / 255.0
# 增加一个维度,变成4维张量
plate = np.expand_dims(plate, axis=0)
# 使用模型进行预测
pred = model.predict(plate)
# 返回车牌坐标和预测结果
return max_box, pred
# 加载测试图像
img = cv2.imread('test.jpg')
# 进行车牌检测
result = detect_plate(img)
if result is not None:
box, pred = result
x, y, w, h = box
# 绘制车牌边框
cv2.rectangle(img, (x, y), (x+w, y+h), (0, 255, 0), 2)
# 显示预测结果
pred_label = np.argmax(pred)
cv2.putText(img, str(pred_label), (x, y-10), cv2.FONT_HERSHEY_SIMPLEX, 1, (0, 0, 255), 2)
# 显示结果图像
cv2.imshow('result', img)
cv2.waitKey(0)
cv2.destroyAllWindows()
```
需要注意的是,这里的模型是针对特定的车牌数据集训练的,如果要应用到其他数据集上需要重新训练模型。此外,车牌检测还有很多细节需要处理,如车牌旋转、光照变化等问题,这里只是一个简单的示例。
阅读全文
相关推荐
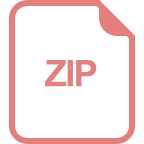


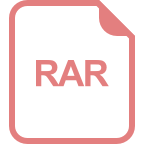
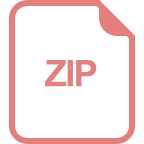
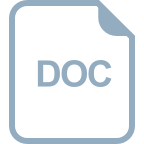







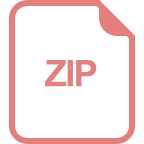
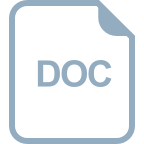
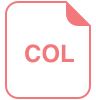
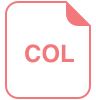