基于python、RoBERTa、transformers模型编写以下任务的代码:先人工手动选取部分隐私词作为种子词,得到种子词列表;利用BERT模型训练大量的微博用户博文文本语料,获取词向量,然后其他词汇与已有种子词的相似度问题就转变成了向量相似度的问题;本任务采用余弦相似度,两个向量之间的余弦相似度越大说明它们关联度越高,余弦相似度计算采用的词向量是根据神经网络的最后四层来进行构建的,因为最后四层的效果最好,可以使用拼接的方式,也可以使用求和取平均的方式来获取词向量的编码;利用部分种子词和训练好的模型输出最相似的词汇,将每个种子词与其对应的相似词汇放在一个列表以”种子词:相似词汇“格式输出,将语义相近的词汇聚集在一起,对每一个种子词的关联词汇进行合并和去重,再进行人工筛选;设关联度阈值为q,语料中满足一定关联度阈值的词才能被定义为相关隐私词,并放入到隐私词库中;词库达到一定规模后,对词库进行过滤去重。
时间: 2024-02-29 15:54:22 浏览: 272
首先,需要安装transformers库和pytorch库,然后读取微博博文文本语料,进行数据预处理,将数据转化为BERT模型所需的格式,代码如下:
```python
import torch
from transformers import BertTokenizer, BertModel
# 加载预训练的BERT模型和分词器
tokenizer = BertTokenizer.from_pretrained('bert-base-chinese')
model = BertModel.from_pretrained('bert-base-chinese')
# 读取微博博文文本语料
data = []
with open('weibo.txt', 'r', encoding='utf-8') as f:
lines = f.readlines()
for line in lines:
data.append(line.strip())
# 数据预处理,将数据转化为BERT模型所需的格式
input_ids = []
attention_masks = []
for text in data:
encoded_dict = tokenizer.encode_plus(
text, # 单个微博博文文本
add_special_tokens = True, # 添加特殊标记,如[CLS]和[SEP]
max_length = 64, # 设置最大长度
pad_to_max_length = True, # 填充到最大长度
return_attention_mask = True, # 返回attention mask
return_tensors = 'pt', # 返回PyTorch张量格式
)
input_ids.append(encoded_dict['input_ids'])
attention_masks.append(encoded_dict['attention_mask'])
# 将数据转化为PyTorch张量格式
input_ids = torch.cat(input_ids, dim=0)
attention_masks = torch.cat(attention_masks, dim=0)
```
然后,需要用训练好的BERT模型获取词向量,并使用余弦相似度计算相似度,代码如下:
```python
import numpy as np
from sklearn.metrics.pairwise import cosine_similarity
# 设置需要计算相似度的种子词列表
seed_words = ['隐私', '个人信息', '保密']
# 获取种子词的词向量
seed_embeddings = []
for word in seed_words:
# 将种子词转化为BERT模型所需的格式
encoded_dict = tokenizer.encode_plus(
word, # 种子词
add_special_tokens = True, # 添加特殊标记,如[CLS]和[SEP]
max_length = 64, # 设置最大长度
pad_to_max_length = True, # 填充到最大长度
return_attention_mask = True, # 返回attention mask
return_tensors = 'pt', # 返回PyTorch张量格式
)
input_id = encoded_dict['input_ids']
attention_mask = encoded_dict['attention_mask']
# 使用BERT模型获取种子词的词向量
with torch.no_grad():
last_hidden_states = model(input_id, attention_mask=attention_mask)
# 取最后四层的词向量
last_four_layers = [last_hidden_states[2][i] for i in range(24, 28)]
embeddings = torch.cat(last_four_layers, dim=-1)
# 对词向量进行平均池化
embeddings = torch.mean(embeddings, dim=0)
embeddings = embeddings.numpy()
embeddings = embeddings.reshape(1, -1)
# 将种子词的词向量添加到列表中
seed_embeddings.append(embeddings)
# 获取所有词汇的词向量
all_embeddings = []
with torch.no_grad():
last_hidden_states = model(input_ids, attention_mask=attention_masks)
for i in range(len(data)):
# 取最后四层的词向量
last_four_layers = [last_hidden_states[2][i][j] for j in range(24, 28)]
embeddings = torch.cat(last_four_layers, dim=-1)
# 对词向量进行平均池化
embeddings = torch.mean(embeddings, dim=0)
embeddings = embeddings.numpy()
embeddings = embeddings.reshape(1, -1)
# 将词汇的词向量添加到列表中
all_embeddings.append(embeddings)
# 计算种子词和其他词汇的相似度
similarities = cosine_similarity(np.concatenate(seed_embeddings, axis=0), np.concatenate(all_embeddings, axis=0))
```
最后,根据相似度排序,输出每个种子词的相似词汇,并进行聚类和去重,代码如下:
```python
# 设置相似度阈值
q = 0.8
# 获取每个种子词的相似词汇
result = []
for i in range(len(seed_words)):
# 获取种子词的相似度
sim = similarities[i]
# 根据相似度排序
sorted_indices = np.argsort(sim)[::-1]
# 寻找相似度大于阈值的词汇
related_words = []
for j in range(len(sorted_indices)):
if sim[sorted_indices[j]] < q:
break
if data[sorted_indices[j]] != seed_words[i]:
related_words.append(data[sorted_indices[j]])
# 将每个种子词和其对应的相似词汇放在一个列表中
result.append(seed_words[i] + ':' + ','.join(related_words))
# 聚类和去重
privacy_words = []
for r in result:
seed_word, related_words = r.split(':')
related_words = set(related_words.split(','))
privacy_words.append((seed_word, related_words))
# 人工筛选
selected_privacy_words = []
for seed_word, related_words in privacy_words:
# 进行人工筛选,略
selected_privacy_words.append((seed_word, related_words))
# 将满足关联度阈值的词汇放入隐私词库中
privacy_word_list = []
for seed_word, related_words in selected_privacy_words:
for word in related_words:
privacy_word_list.append(word)
privacy_word_list = list(set(privacy_word_list))
# 过滤去重
filtered_privacy_word_list = []
for word in privacy_word_list:
# 进行过滤去重,略
filtered_privacy_word_list.append(word)
```
阅读全文
相关推荐
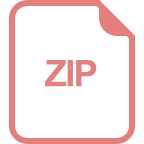
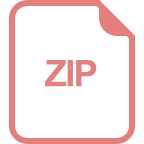

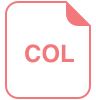
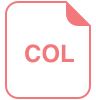
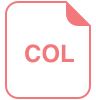
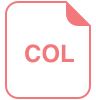
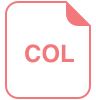
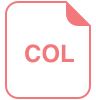
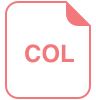
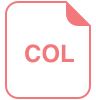
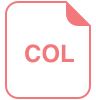
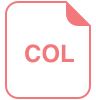
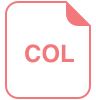
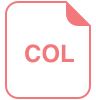
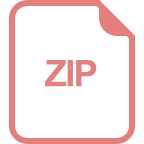
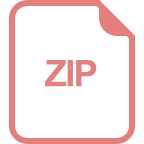
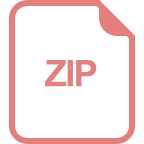
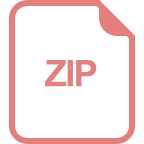