torch.softmax函数和relu
时间: 2024-06-12 21:10:54 浏览: 182
torch.softmax函数和relu是深度学习中常用的激活函数。它们的作用不同,softmax函数通常用于多分类问题中,将输出转化为概率分布;而relu函数则常用于神经网络中,可以增强模型的非线性拟合能力。
具体来说,softmax函数将一个向量转化为概率分布,公式为:
$$
\text{softmax}(x_i) = \frac{e^{x_i}}{\sum_{j=1}^n e^{x_j}}
$$
其中 $x_i$ 表示向量中的第 $i$ 个元素,$n$ 表示向量的长度。softmax函数的输出是一个概率分布,所有元素的和为1。
而relu函数则是一个非线性函数,公式为:
$$
\text{ReLU}(x) = \max(0, x)
$$
当输入 $x$ 大于0时,输出为 $x$;当输入 $x$ 小于等于0时,输出为0。relu函数的作用是增强模型的非线性拟合能力,可以有效地解决梯度消失问题。
相关问题
class Attention(torch.nn.Module): def __init__(self, in_features, out_features): super(Attention, self).__init__() self.lin1 = torch.nn.Linear(in_features, out_features) self.lin2 = torch.nn.Linear(out_features, 1) def forward(self, x): x = F.relu(self.lin1(x)) x = self.lin2(x) return torch.softmax(x, dim=0).view(-1, 1)这段代码是什么样的注意力机制的代码
这段代码实现的是一种简单的注意力机制,又称为全局注意力。它的输入是一个张量x,经过两层全连接层后输出一个标量,再经过softmax函数将其转换为注意力分布。这个注意力分布可以应用于输入张量的每个元素,以加权其重要性。具体来说,对于输入张量x,注意力分布的大小与x的每个元素相关,大的元素对应的注意力分布大,小的元素对应的注意力分布小。最终,输出是加权平均值,即x的每个元素乘以对应的注意力分布,再求和。
为以下代码写注释:class TransformerClassifier(torch.nn.Module): def __init__(self, num_labels): super().__init__() self.bert = BertForSequenceClassification.from_pretrained('bert-base-chinese', num_labels=num_labels) # print(self.bert.config.hidden_size) #768 self.dropout = torch.nn.Dropout(0.1) self.classifier1 = torch.nn.Linear(640, 256) self.classifier2 = torch.nn.Linear(256, num_labels) self.regress1 = torch.nn.Linear(640, 256) self.regress2 = torch.nn.Linear(256, 2) self.regress3 = torch.nn.Linear(640, 256) self.regress4 = torch.nn.Linear(256, 2) # self.regress3 = torch.nn.Linear(64, 1) # self.regress3 = torch.nn.Linear(640, 256) # self.regress4 = torch.nn.Linear(256, 1) # self.soft1 = torch.nn.Softmax(dim=1) def forward(self, input_ids, attention_mask, token_type_ids): # outputs = self.bert(input_ids, attention_mask=attention_mask, token_type_ids=token_type_ids) # pooled_output = outputs.logits # # pooled_output = self.dropout(pooled_output) # # logits = self.classifier(pooled_output) outputs = self.bert(input_ids, attention_mask=attention_mask, token_type_ids=token_type_ids) logits = outputs.logits clas = F.relu(self.classifier1(logits)) clas = self.classifier2(clas) death = F.relu(self.regress1(logits)) # xingqi = F.relu(self.regress2(xingqi)) death = self.regress2(death) life = F.relu(self.regress3(logits)) # xingqi = F.relu(self.regress2(xingqi)) life = self.regress4(life) # fakuan = F.relu(self.regress3(logits)) # fakuan = self.regress4(fakuan) # print(logits.shape) # logits = self.soft1(logits) # print(logits) # print(logits.shape) return clas,death,life
注释如下:
```
class TransformerClassifier(torch.nn.Module): # 定义一个名为TransformerClassifier的继承自nn.Module的类
def __init__(self, num_labels): # 初始化函数,num_labels为标签数
super().__init__() # 调用父类的构造函数
self.bert = BertForSequenceClassification.from_pretrained('bert-base-chinese', num_labels=num_labels) # 用预训练的中文Bert模型初始化self.bert, 输出维度为num_labels
# print(self.bert.config.hidden_size) #768
self.dropout = torch.nn.Dropout(0.1) # 定义dropout层,丢弃概率为0.1
self.classifier1 = torch.nn.Linear(640, 256) # 定义一个全连接层,输入维度为640,输出维度为256
self.classifier2 = torch.nn.Linear(256, num_labels) # 定义一个全连接层,输入维度为256,输出维度为num_labels
self.regress1 = torch.nn.Linear(640, 256) # 定义一个全连接层,输入维度为640,输出维度为256
self.regress2 = torch.nn.Linear(256, 2) # 定义一个全连接层,输入维度为256,输出维度为2
self.regress3 = torch.nn.Linear(640, 256) # 定义一个全连接层,输入维度为640,输出维度为256
self.regress4 = torch.nn.Linear(256, 2) # 定义一个全连接层,输入维度为256,输出维度为2
def forward(self, input_ids, attention_mask, token_type_ids): # 前向传播函数,输入参数分别为input_ids、attention_mask、token_type_ids
outputs = self.bert(input_ids, attention_mask=attention_mask, token_type_ids=token_type_ids) # 将输入传入self.bert中,得到输出
logits = outputs.logits # 从输出中得到logits
clas = F.relu(self.classifier1(logits)) # 将logits输入到self.classifier1中,经过relu函数后得到clas
clas = self.classifier2(clas) # 将clas输入到self.classifier2中,得到分类结果
death = F.relu(self.regress1(logits)) # 将logits输入到self.regress1中,经过relu函数后得到death
death = self.regress2(death) # 将death输入到self.regress2中,得到死亡概率
life = F.relu(self.regress3(logits)) # 将logits输入到self.regress3中,经过relu函数后得到life
life = self.regress4(life) # 将life输入到self.regress4中,得到生存概率
return clas, death, life # 返回分类结果、死亡概率、生存概率
阅读全文
相关推荐
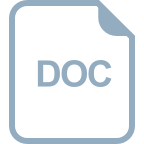
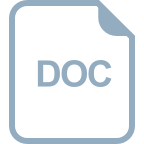
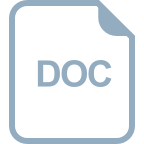














