def forward(self, inp): x = inp x = self.norm1(x) x = self.conv1(x) x = self.conv2(x) x = self.gelu(x) x = x * self.se(x) x = self.conv3(x) x = self.dropout1(x) y = inp + x * self.beta x = self.conv4(self.norm2(y)) x = self.gelu(x) x = self.conv5(x) x = self.dropout2(x) return y + x * self.gamma代码中文含义
时间: 2023-12-04 10:03:46 浏览: 187
这段代码是一个神经网络的前向传播函数。在这个函数中,输入(inp)首先通过一个归一化层(norm1)进行归一化,然后通过两个卷积层(conv1和conv2)进行特征提取。接着,通过一个GELU激活函数(gelu)进行非线性变换,并通过一个SE模块(se)对特征进行加权。然后再通过一个卷积层(conv3)进行进一步的特征提取,并通过一个Dropout层(dropout1)进行正则化。此外,还定义了两个参数beta和gamma,分别用于调整残差连接(y = inp + x * beta)和特征缩放(return y + x * gamma)。最后,再通过两个卷积层(conv4和conv5)和一个Dropout层(dropout2)进行最终的特征提取和正则化,并将其与残差连接相加作为输出。
相关问题
out = self.inp_prelu(self.inp_snorm(self.inp_conv(x)))
This code represents a neural network layer where an input tensor x is passed through a series of operations:
1. The first operation is inp_conv, which performs a convolution operation on the input tensor with some learnable filters.
2. The output of the convolution operation is then passed through inp_snorm, which performs a spatial normalization operation to normalize the output tensor across channels and spatial dimensions.
3. The normalized output is then passed through inp_prelu, which applies a parametric rectified linear unit (PReLU) activation function to introduce non-linearity.
4. Finally, the output of the PReLU activation function is returned as the output of the layer.
Overall, this layer can be used as a building block for a deeper neural network architecture to learn more complex representations of input data.
class CoordAtt(nn.Module): def __init__(self, inp, oup, reduction=32): super(CoordAtt, self).__init__() self.pool_h = nn.AdaptiveAvgPool2d((None, 1)) self.pool_w = nn.AdaptiveAvgPool2d((1, None)) mip = max(8, inp // reduction) self.conv1 = nn.Conv2d(inp, mip, kernel_size=1, stride=1, padding=0) self.bn1 = nn.BatchNorm2d(mip) self.act = h_swish() self.conv_h = nn.Conv2d(mip, oup, kernel_size=1, stride=1, padding=0) self.conv_w = nn.Conv2d(mip, oup, kernel_size=1, stride=1, padding=0) def forward(self, x): identity = x n, c, h, w = x.size() x_h = self.pool_h(x) x_w = self.pool_w(x).permute(0, 1, 3, 2) y = torch.cat([x_h, x_w], dim=2) y = self.conv1(y) y = self.bn1(y) y = self.act(y) x_h, x_w = torch.split(y, [h, w], dim=2) x_w = x_w.permute(0, 1, 3, 2) a_h = self.conv_h(x_h).sigmoid() a_w = self.conv_w(x_w).sigmoid() out = identity * a_w * a_h return out将里面的平均池化变为平均池化和最大池化并联的形式
你可以将CoordAtt的__init__函数中的self.pool_h和self.pool_w修改为同时包含平均池化和最大池化的模块,例如:
```
self.pool_h = nn.Sequential(
nn.AdaptiveAvgPool2d((None, 1)),
nn.AdaptiveMaxPool2d((None, 1))
)
self.pool_w = nn.Sequential(
nn.AdaptiveAvgPool2d((1, None)),
nn.AdaptiveMaxPool2d((1, None))
)
```
这样修改后,CoordAtt的前向传播函数不需要做任何修改,就可以同时使用平均池化和最大池化。
阅读全文
相关推荐
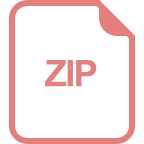













