X_train, X_test, y_train, y_test = train_test_split( inputs, mises_stress, test_size=0.2, random_state=42
时间: 2024-04-04 14:27:15 浏览: 16
train_test_split是一个用于将数据集划分为训练集和测试集的函数。它的参数包括inputs(输入数据),mises_stress(目标变量),test_size(测试集占总数据集的比例),random_state(随机种子)。
函数的返回值是四个数组:X_train、X_test、y_train和y_test。其中,X_train包含了训练集的输入数据,X_test包含了测试集的输入数据,y_train包含了训练集的目标变量,y_test包含了测试集的目标变量。
这个函数的作用是将输入数据和目标变量按照指定的比例划分为训练集和测试集,以便进行机器学习模型的训练和评估。
相关问题
X_train, X_test, y_train, y_test = train_test_split(X, y, test_size=0.2, random_state=42)
This code snippet is splitting the dataset into training and testing sets using the train_test_split function from the scikit-learn library.
The inputs are X (the features) and y (the target variable), which are the two arrays that contain the data points. The test_size parameter specifies the proportion of the dataset that should be used for testing, and random_state ensures that the same split is obtained each time the code is run.
The outputs are four arrays: X_train and y_train are the training sets (used to train the machine learning model), while X_test and y_test are the testing sets (used to evaluate the performance of the model).
rain_test_split
```python
from sklearn.model_selection import train_test_split
# 输出train_test_split函数的文档字符串
help(train_test_split)
```
输出结果如下:
```
Help on function train_test_split in module sklearn.model_selection._split:
train_test_split(*arrays, **options)
Split arrays or matrices into random train and test subsets
Quick utility that wraps input validation and next(ShuffleSplit().split(X, y)).
Parameters
----------
*arrays : sequence of indexables with same length / shape[0]
Allowed inputs are lists, numpy arrays, scipy-sparse
matrices or pandas dataframes.
test_size : float or int, default=None
If float, should be between 0.0 and 1.0 and represent the proportion
of the dataset to include in the test split. If int, represents the
absolute number of test samples. If None, the value is set to the
complement of the train size. If ``train_size`` is also None, it will
be set to 0.25.
train_size : float or int, default=None
If float, should be between 0.0 and 1.0 and represent the
proportion of the dataset to include in the train split. If
int, represents the absolute number of train samples. If None,
the value is automatically set to the complement of the test size.
random_state : int or RandomState instance, default=None
Controls the shuffling applied to the data before applying the split.
Pass an int for reproducible output across multiple function calls.
See :term:`Glossary <random_state>`.
shuffle : bool, default=True
Whether or not to shuffle the data before splitting. If shuffle=False
then stratify must be None.
stratify : array-like, default=None
If not None, data is split in a stratified fashion, using this as
the class labels.
Returns
-------
splitting : list, length=2 * len(arrays)
List containing train-test split of inputs.
.. versionadded:: 0.16
Added option to specify a list of arrays to be split
Examples
--------
>>> import numpy as np
>>> from sklearn.model_selection import train_test_split
>>> X, y = np.arange(10).reshape((5, 2)), range(5)
>>> X
array([[0, 1],
[2, 3],
[4, 5],
[6, 7],
[8, 9]])
>>> list(y)
[0, 1, 2, 3, 4]
>>> X_train, X_test, y_train, y_test = train_test_split(
... X, y, test_size=0.33, random_state=42)
...
>>> X_train
array([[4, 5],
[0, 1],
[6, 7]])
>>> y_train
[2, 0, 3]
>>> X_test
array([[2, 3],
[8, 9]])
>>> y_test
[1, 4]
```
相关推荐
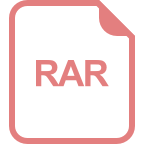
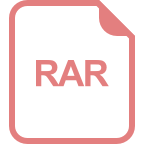
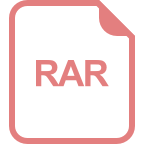













