用python翻译List<Result_Line> vLines = ResultLineList.Where(line => line.VLineOrder != null).ToList();
时间: 2024-03-17 20:41:11 浏览: 20
这段代码的意思是:在ResultLineList中筛选出VLineOrder不为null的Result_Line对象,并将其转换为List类型,存储在vLines变量中。
对应的Python代码如下:
```
vLines = [line for line in ResultLineList if line.VLineOrder is not None]
```
其中,通过列表推导式实现了对ResultLineList的筛选和转换操作,判断VLineOrder是否为None的语法为`is not None`。
相关问题
把c#代码转为python c#代码private void CalculatePartial_AvgVelocity_Width() { int index = -1; List<Result_Line> vLines = ResultLineList.Where(line => line.VLineOrder != null).ToList(); for (int i = 0; i < vLines.Count; i++) { index = ResultLineList.IndexOf(vLines[i]); if (vLines[i].VLineOrder == -9999) { continue; } else if (vLines[i - 1].VLineOrder == -9999) { //float leftAlpha = GetInshoreCoefficient(-9999, vLines[i].WaterLvl); float leftAlpha = NumValid.PrecisionConvert_Coefficient(GetInshoreCoefficient(-9999, vLines[i - 1].InitPointDistance)); vLines[i].PartialAvgVelocity = NumValid.PrecisionConvert_Velocity((float)(leftAlpha * vLines[i].AvgVelocity)); } else if (vLines[i].VLineOrder == 9999) { //float rightAlpha = GetInshoreCoefficient(9999, vLines[i].WaterLvl); float rightAlpha = NumValid.PrecisionConvert_Coefficient(GetInshoreCoefficient(9999, vLines[i].InitPointDistance)); vLines[i].PartialAvgVelocity = NumValid.PrecisionConvert_Velocity((float)(rightAlpha * vLines[i - 1].AvgVelocity)); } else { var temp = (vLines[i - 1].AvgVelocity + vLines[i].AvgVelocity) / 2f; vLines[i].PartialAvgVelocity = NumValid.PrecisionConvert_Velocity((float)temp); } ResultLineList[index] = vLines[i]; ResultLineList[index].WidthBetweenVLine = NumValid.PrecisionConvert_Width(vLines[i].InitPointDistance - vLines[i - 1].InitPointDistance); } }
将C#代码转换为Python代码如下:
def CalculatePartial_AvgVelocity_Width():
index = -1
vLines = list(filter(lambda line: line.VLineOrder != None, ResultLineList))
for i in range(len(vLines)):
index = ResultLineList.index(vLines[i])
if vLines[i].VLineOrder == -9999:
continue
elif vLines[i - 1].VLineOrder == -9999:
leftAlpha = NumValid.PrecisionConvert_Coefficient(GetInshoreCoefficient(-9999, vLines[i - 1].InitPointDistance))
vLines[i].PartialAvgVelocity = NumValid.PrecisionConvert_Velocity(float(leftAlpha * vLines[i].AvgVelocity))
elif vLines[i].VLineOrder == 9999:
rightAlpha = NumValid.PrecisionConvert_Coefficient(GetInshoreCoefficient(9999, vLines[i].InitPointDistance))
vLines[i].PartialAvgVelocity = NumValid.PrecisionConvert_Velocity(float(rightAlpha * vLines[i - 1].AvgVelocity))
else:
temp = (vLines[i - 1].AvgVelocity + vLines[i].AvgVelocity) / 2.0
vLines[i].PartialAvgVelocity = NumValid.PrecisionConvert_Velocity(float(temp))
ResultLineList[index] = vLines[i]
ResultLineList[index].WidthBetweenVLine = NumValid.PrecisionConvert_Width(vLines[i].InitPointDistance - vLines[i - 1].InitPointDistance)
if continue_flag == 1: q_online = torch.load('Result_discount=0.9_lr=0.0005_net') q_target = torch.load('Result_discount=0.9_lr=0.0005_net') buffer_save = np.load('Result_discount=0.9_lr=0.0005_buffer.npy', allow_pickle=True) memory.buffer = collections.deque(buffer_save.tolist(), maxlen=buffer_limit) reward_ave_list = np.load('Result_discount=0.9_lr=0.0005_reward.npy').tolist() loss_ave_list = np.load('Result_discount=0.9_lr=0.0005_loss.npy').tolist()
这段代码中,首先检查 `continue_flag` 是否等于 1。如果是,则加载模型和数据,以便继续训练或使用之前保存的结果。
- `q_online = torch.load('Result_discount=0.9_lr=0.0005_net')` 会加载之前训练好的神经网络模型并将其赋值给 `q_online` 变量。
- `q_target = torch.load('Result_discount=0.9_lr=0.0005_net')` 同样是加载神经网络模型,并赋值给 `q_target` 变量。在一些深度强化学习算法中,存在两个网络,一个用于估计当前状态的值(`q_online`),另一个用于估计目标状态的值(`q_target`)。
- `buffer_save = np.load('Result_discount=0.9_lr=0.0005_buffer.npy', allow_pickle=True)` 加载之前保存的经验缓存数据,并将其转换为Python的`deque`对象,并赋值给 `memory.buffer`。这样做是为了继续使用之前的经验数据。
- `reward_ave_list = np.load('Result_discount=0.9_lr=0.0005_reward.npy').tolist()` 加载之前保存的平均奖励列表,并将其转换为Python的列表形式。
- `loss_ave_list = np.load('Result_discount=0.9_lr=0.0005_loss.npy').tolist()` 加载之前保存的平均损失列表,并将其转换为Python的列表形式。
通过这些加载操作,您可以在之前训练的基础上进行继续训练或使用之前保存的结果进行其他操作。请确保在加载模型和数据之前,已经将相关文件保存在正确的路径下,并且文件格式与加载函数相匹配。
相关推荐
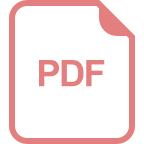














