torch.repeat 和 torch.repeat_
时间: 2023-11-18 16:54:50 浏览: 37
torch.repeat和torch.repeat_都是PyTorch中的函数,用于对张量进行重复操作。它们的区别在于,torch.repeat返回一个新的张量,而torch.repeat_直接在原始张量上进行操作。
具体来说,torch.repeat(dim, repeats)函数会将张量在指定维度上重复指定次数,返回一个新的张量。其中,dim参数表示需要重复的维度,repeats参数表示需要重复的次数。例如,a.repeat(2, dim=0)表示将a在第0维上重复2次。
而torch.repeat_(dim, repeats)函数则是直接在原始张量上进行操作,将张量在指定维度上重复指定次数。其中,dim参数和repeats参数的含义与torch.repeat相同。
需要注意的是,torch.repeat和torch.repeat_都会返回一个新的张量,而不是在原始张量上进行操作。如果需要在原始张量上进行操作,需要使用torch.repeat_函数。
相关问题
torch.repeat_interleave(
torch.repeat_interleave()函数是PyTorch中的一个函数,用于将输入张量中的元素重复指定次数。函数的原型为torch.repeat_interleave(input, repeats, dim=None),其中input是输入张量,repeats是每个元素的重复次数,dim是需要重复的维度,默认情况下dim=None,表示将输入张量展平为向量,然后将每个元素重复repeats次,并返回重复后的张量。\[1\]
举例说明:
```
x = torch.tensor(\[1, 2, 3\])
x.repeat_interleave(2)
# 输出: tensor(\[1, 1, 2, 2, 3, 3\])
y = torch.tensor(\[\[1, 2\], \[3, 4\]\])
torch.repeat_interleave(y, 2)
# 输出: tensor(\[1, 1, 2, 2, 3, 3, 4, 4\])
torch.repeat_interleave(y, 3, dim=0)
# 输出: tensor(\[\[1, 2\], \[1, 2\], \[1, 2\], \[3, 4\], \[3, 4\], \[3, 4\]\])
torch.repeat_interleave(y, 3, dim=1)
# 输出: tensor(\[\[1, 1, 1, 2, 2, 2\], \[3, 3, 3, 4, 4, 4\]\])
torch.repeat_interleave(y, torch.tensor(\[1, 2\]), dim=0)
# 输出: tensor(\[\[1, 2\], \[3, 4\], \[3, 4\]\])
```
以上是一些使用torch.repeat_interleave()函数的示例,可以根据需要指定重复次数和重复的维度来实现不同的重复操作。\[2\]
注意:在传入多维张量时,函数会默认将其展平为向量进行重复操作。\[2\]
#### 引用[.reference_title]
- *1* *3* [Pytorch中torch.repeat_interleave()函数解析](https://blog.csdn.net/flyingluohaipeng/article/details/125039411)[target="_blank" data-report-click={"spm":"1018.2226.3001.9630","extra":{"utm_source":"vip_chatgpt_common_search_pc_result","utm_medium":"distribute.pc_search_result.none-task-cask-2~all~insert_cask~default-1-null.142^v91^control_2,239^v3^insert_chatgpt"}} ] [.reference_item]
- *2* [torch.repeat_interleave()函数详解](https://blog.csdn.net/weixin_43823669/article/details/126283277)[target="_blank" data-report-click={"spm":"1018.2226.3001.9630","extra":{"utm_source":"vip_chatgpt_common_search_pc_result","utm_medium":"distribute.pc_search_result.none-task-cask-2~all~insert_cask~default-1-null.142^v91^control_2,239^v3^insert_chatgpt"}} ] [.reference_item]
[ .reference_list ]
torch.repeat
引用\[1\]:torch.repeat_interleave()函数可以对张量进行重复扩充。它的功能是重复张量的元素,并返回一个新的张量。输入参数包括input(输入张量)、repeats(重复的次数)和dim(在哪个维度进行重复)。默认情况下,该函数会将输入张量展平为向量,然后将每个元素重复repeats次,并返回重复后的张量。\[1\]
引用\[2\]:torch.repeat()函数也可以对张量进行重复扩充。当参数只有两个时,表示行的重复倍数和列的重复倍数,其中1表示不重复。当参数有三个时,表示通道数的重复倍数、行的重复倍数和列的重复倍数,其中1表示不重复。\[3\]
综上所述,torch.repeat_interleave()函数和torch.repeat()函数都可以用来对张量进行重复扩充,但是它们的参数和功能略有不同。
#### 引用[.reference_title]
- *1* *2* [torch.repeat_interleave()函数详解](https://blog.csdn.net/weixin_43823669/article/details/126283277)[target="_blank" data-report-click={"spm":"1018.2226.3001.9630","extra":{"utm_source":"vip_chatgpt_common_search_pc_result","utm_medium":"distribute.pc_search_result.none-task-cask-2~all~insert_cask~default-1-null.142^v91^control_2,239^v3^insert_chatgpt"}} ] [.reference_item]
- *3* [Pytorch中torch.repeat()函数解析](https://blog.csdn.net/flyingluohaipeng/article/details/125039368)[target="_blank" data-report-click={"spm":"1018.2226.3001.9630","extra":{"utm_source":"vip_chatgpt_common_search_pc_result","utm_medium":"distribute.pc_search_result.none-task-cask-2~all~insert_cask~default-1-null.142^v91^control_2,239^v3^insert_chatgpt"}} ] [.reference_item]
[ .reference_list ]
相关推荐
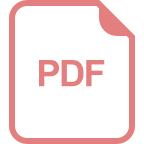
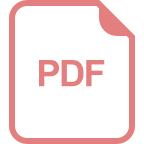













