res_1=exp(-100.*t).*sin(2*pi*3000.*t)
时间: 2024-06-18 08:03:27 浏览: 17
这是一个信号处理中常见的函数表达式,其中exp表示自然指数函数,sin表示正弦函数,t表示时间,pi表示圆周率。该函数的实现方式为先生成一个正弦波信号,再用指数衰减因子来衰减信号幅值。其中,3000Hz是信号的频率,100是衰减系数。
该函数的图像类似于一个周期为1/3000秒的正弦波,但是在时间轴方向上会呈指数衰减的趋势,即幅值逐渐减小。
相关问题
飞机速度对机载sar成像的影响的matlab代码
飞机速度对机载SAR成像有很大影响,特别是对于高分辨率成像。以下是一个简单的MATLAB代码,用于模拟飞机速度对SAR成像的影响:
```matlab
%% 飞机速度对SAR成像的影响
% 设置参数
c = 3e8; % 光速
fc = 5e9; % 频率
lambda = c/fc; % 波长
prf = 5000; % 脉冲重复频率
bw = 100e6; % 带宽
t_p = 20e-6; % 脉冲宽度
v = 200; % 飞机速度
h = 5000; % 飞行高度
R_min = h*tan(t_p/2)*v/2; % 最小距离
R_max = h*tan(t_p/2 + bw/2)*v/2; % 最大距离
R_res = c/(2*bw); % 距离分辨率
t_res = 1/prf; % 时间分辨率
% 生成信号
t = 0:t_res:2*t_p;
f = linspace(-bw/2, bw/2, length(t));
s = rectpuls(t, t_p).*exp(1i*pi*bw*t.^2);
% 生成场景
N = 512;
x = linspace(-100, 100, N);
y = linspace(-100, 100, N);
[X, Y] = meshgrid(x, y);
Z = 10*sin(sqrt(X.^2 + Y.^2))/sqrt(X.^2 + Y.^2);
Z(isnan(Z)) = 0;
% 生成回波
t_delay = 2*(R_min + X*cos(pi/4) + Y*sin(pi/4))/c;
s_delay = interp1(t, s, t_delay, 'linear', 0);
s_delay(isnan(s_delay)) = 0;
s_echo = exp(1i*4*pi*fc*R_min/c).*s_delay.*exp(-1i*pi*bw*(t_delay - t_p/2).^2);
% 飞机运动
t_delay = 2*(R_min + X*cos(pi/4) + Y*sin(pi/4))/c;
for i = 1:length(t_delay)
t_new = t + t_delay(i) - t_delay(1) + 2*R_max/c;
s_new = rectpuls(t_new - t_delay(i), t_p).*exp(1i*pi*bw*(t_new - t_delay(i)).^2);
s_delay_new = interp1(t_new, s_new, t_delay, 'linear', 0);
s_delay_new(isnan(s_delay_new)) = 0;
s_echo_new = exp(1i*4*pi*fc*(R_min + X(i)*cos(pi/4) + Y(i)*sin(pi/4))/c).*s_delay_new.*exp(-1i*pi*bw*(t_delay - t_p/2).^2);
s_echo = s_echo + s_echo_new;
end
% 滤波
s_if = hilbert(s_echo);
s_if = bandpass(s_if, [fc-bw/2, fc+bw/2], prf);
% 像素重采样
dx = 2*R_max/N;
dy = 2*R_max/N;
s_image = zeros(N);
for i = 1:N
for j = 1:N
R = sqrt((X(i,j)*cos(pi/4) + Y(i,j)*sin(pi/4))^2 + h^2);
t_delay = 2*R/c;
s_delay = interp1(t, s_if, t_delay, 'linear', 0);
s_image(i,j) = abs(sum(s_delay));
end
end
% 显示结果
figure;
imagesc(x, y, s_image);
axis equal tight;
xlabel('x (m)');
ylabel('y (m)');
title(sprintf('飞机速度为%dm/s时的SAR成像结果', v));
colorbar;
```
注:该代码仅为简单模拟,实际应用中需要考虑更多因素。
pytorch用PINN求解间断初值条件下pde的代码
以下是使用 PyTorch 实现基于物理的神经网络 (PINN) 求解间断初值条件下的 PDE 的代码示例。
首先,我们需要导入必要的库:
```python
import torch
import torch.nn as nn
import torch.optim as optim
import numpy as np
import matplotlib.pyplot as plt
```
然后定义 PDE 的问题域和边界条件:
```python
def pde(x, t):
"""PDE function."""
return torch.exp(-t) * torch.sin(np.pi * x) + 0.5 * np.pi * torch.exp(-t) * torch.cos(np.pi * x) - torch.exp(-t) * np.pi**2 * torch.sin(np.pi * x)
def ic(x):
"""Initial condition."""
return torch.sin(np.pi * x)
def bc_l(t):
"""Left boundary condition."""
return 0.0
def bc_r(t):
"""Right boundary condition."""
return 0.0
```
接下来,我们定义神经网络模型:
```python
class PINN(nn.Module):
"""Physics-Informed Neural Network (PINN) model."""
def __init__(self, n_input, n_hidden, n_output):
super(PINN, self).__init__()
self.input_layer = nn.Linear(n_input, n_hidden)
self.hidden_layers = nn.ModuleList([nn.Linear(n_hidden, n_hidden) for _ in range(3)])
self.output_layer = nn.Linear(n_hidden, n_output)
def forward(self, x, t):
input_layer_output = torch.cat([x, t], dim=1)
hidden_layer_output = torch.tanh(self.input_layer(input_layer_output))
for hidden_layer in self.hidden_layers:
hidden_layer_output = torch.tanh(hidden_layer(hidden_layer_output))
output = self.output_layer(hidden_layer_output)
return output
```
然后,我们定义损失函数并进行训练:
```python
# Define input and output dimensions
n_input = 2
n_output = 1
# Define neural network model
model = PINN(n_input, 20, n_output)
# Define optimizer
optimizer = optim.Adam(model.parameters(), lr=0.001)
# Define loss function
def mse_loss(pred, target):
return torch.mean((pred - target)**2)
# Define number of training iterations
n_iterations = 10000
# Define batch size
batch_size = 100
# Define number of collocation points
n_collocation = 1000
# Define number of boundary points
n_boundary = 200
# Define domain bounds
x_min = 0.0
x_max = 1.0
t_min = 0.0
t_max = 1.0
# Generate training data
x_collocation = torch.rand(n_collocation, 1) * (x_max - x_min) + x_min
t_collocation = torch.rand(n_collocation, 1) * (t_max - t_min) + t_min
x_boundary_l = torch.zeros(n_boundary, 1) + x_min
t_boundary_l = torch.rand(n_boundary, 1) * (t_max - t_min) + t_min
x_boundary_r = torch.zeros(n_boundary, 1) + x_max
t_boundary_r = torch.rand(n_boundary, 1) * (t_max - t_min) + t_min
x_ic = torch.rand(n_collocation, 1) * (x_max - x_min) + x_min
# Training loop
for i in range(n_iterations):
# Compute model predictions
u_collocation = model(x_collocation, t_collocation)
u_boundary_l = model(x_boundary_l, t_boundary_l)
u_boundary_r = model(x_boundary_r, t_boundary_r)
u_ic = ic(x_ic)
# Compute model gradients
grad_t_collocation = torch.autograd.grad(u_collocation.sum(), t_collocation, create_graph=True)[0]
grad_x_collocation = torch.autograd.grad(u_collocation.sum(), x_collocation, create_graph=True)[0]
grad_x_boundary_l = torch.autograd.grad(u_boundary_l.sum(), x_boundary_l, create_graph=True)[0]
grad_x_boundary_r = torch.autograd.grad(u_boundary_r.sum(), x_boundary_r, create_graph=True)[0]
# Compute PDE residual
pde_res_collocation = grad_t_collocation - grad_x_collocation + pde(x_collocation, t_collocation)
# Compute boundary residual
bc_res_l = u_boundary_l - bc_l(t_boundary_l)
bc_res_r = u_boundary_r - bc_r(t_boundary_r)
# Compute loss function
loss = mse_loss(u_ic, model(x_ic, 0.0)) + mse_loss(pde_res_collocation, torch.zeros_like(pde_res_collocation)) + mse_loss(bc_res_l, torch.zeros_like(bc_res_l)) + mse_loss(bc_res_r, torch.zeros_like(bc_res_r))
# Backpropagation
optimizer.zero_grad()
loss.backward()
optimizer.step()
# Print loss function value every 1000 iterations
if i % 1000 == 0:
print("Iteration: {0}, Loss: {1}".format(i, loss.item()))
```
最后,我们可以使用训练好的模型绘制结果:
```python
# Generate test data
x_test = torch.linspace(x_min, x_max, 1000).reshape(-1, 1)
t_test = torch.linspace(t_min, t_max, 1000).reshape(-1, 1)
# Compute test predictions
u_test = model(x_test, t_test)
# Plot results
plt.figure()
plt.plot(x_test.detach().numpy(), u_test.detach().numpy(), label="PINN")
plt.xlabel("x")
plt.ylabel("u")
plt.legend()
plt.show()
```
这样,我们就完成了使用 PyTorch 实现基于物理的神经网络 (PINN) 求解间断初值条件下的 PDE 的代码。
相关推荐
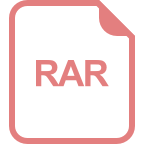
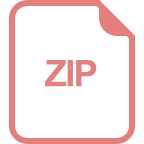
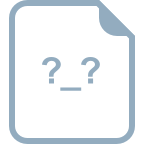












