def __init__(self, machines): self.machines = machines
时间: 2024-05-23 20:14:42 浏览: 133
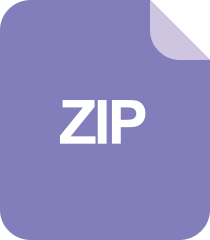
def_init
As an AI language model, I do not have access to the context in which this code is being used. However, based on the code provided, it appears to be defining an __init__ method for a class. The method takes in a parameter called "machines" and assigns it to the "self.machines" attribute of the class. The purpose of this method is likely to initialize instances of the class with a list of machines.
阅读全文
相关推荐
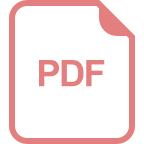
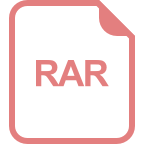


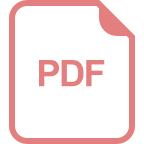
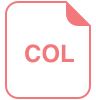
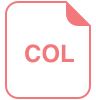
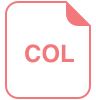
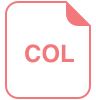
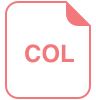
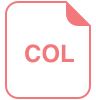
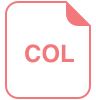
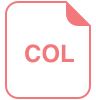
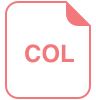
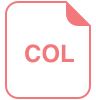
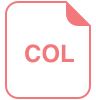
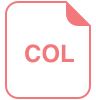