SGD with Momentum如何写在pycharm中
时间: 2024-12-23 22:24:16 浏览: 5
SGD (Stochastic Gradient Descent) 加上动量(Momentum)是一种常用的优化算法,用于更新神经网络模型的权重。在PyCharm中实现这一过程通常涉及到深度学习库如TensorFlow或PyTorch。以下是使用PyTorch的一个简短示例:
```python
import torch
import torch.nn as nn
# 定义一个有动量的SGD优化器
optimizer = torch.optim.SGD(model.parameters(), lr=learning_rate, momentum=0.9)
# 假设`loss.backward()`已经完成了反向传播
for group in optimizer.param_groups:
for param in group['params']:
if param.grad is not None:
param.grad.data.add_(group['momentum'] * param.grad.data)
# 更新参数
param.data -= group['lr'] * param.grad.data
```
在这个例子中,`model.parameters()`获取模型的所有参数,`learning_rate`是学习率,`momentum=0.9`设置了动量值。每次迭代,先计算梯度的加权平均(动量项),然后根据这个平均值和学习率进行参数更新。
相关问题
pycharm里面的SGDClassifier()没有momentum和nesterovs_momentum属性
是的,PyCharm中的SGDClassifier()确实没有momentum和nesterovs_momentum属性。这是因为SGDClassifier()使用的是随机梯度下降算法(SGD),而这些属性是用于改进SGD算法的一些变种,如带有动量的SGD和Nesterov加速梯度的SGD。如果您需要使用这些属性,可以考虑使用其他库,如TensorFlow或Keras,它们提供了更多的优化算法和选项。
在PyCharm中不使用torchvision完成cifar10的卷积神经网络代码
好的,让我来回答你的问题。
首先,我们需要导入必要的库,包括PyTorch、NumPy等:
``` python
import torch
import torch.nn as nn
import torch.optim as optim
import numpy as np
```
接着,我们需要定义一个卷积神经网络。在这个例子中,我们定义一个包含两个卷积层和两个全连接层的神经网络。代码如下:
``` python
class Net(nn.Module):
def __init__(self):
super().__init__()
self.conv1 = nn.Conv2d(3, 6, 5)
self.pool = nn.MaxPool2d(2, 2)
self.conv2 = nn.Conv2d(6, 16, 5)
self.fc1 = nn.Linear(16 * 5 * 5, 120)
self.fc2 = nn.Linear(120, 84)
self.fc3 = nn.Linear(84, 10)
def forward(self, x):
x = self.pool(torch.relu(self.conv1(x)))
x = self.pool(torch.relu(self.conv2(x)))
x = x.view(-1, 16 * 5 * 5)
x = torch.relu(self.fc1(x))
x = torch.relu(self.fc2(x))
x = self.fc3(x)
return x
```
接下来,我们需要定义一些超参数,包括学习率、损失函数、优化器等:
``` python
lr = 0.001
criterion = nn.CrossEntropyLoss()
optimizer = optim.SGD(net.parameters(), lr=lr, momentum=0.9)
```
然后,我们需要加载CIFAR-10数据集并进行预处理:
``` python
from torchvision import datasets, transforms
transform = transforms.Compose([transforms.ToTensor(),
transforms.Normalize((0.5, 0.5, 0.5), (0.5, 0.5, 0.5))])
trainset = datasets.CIFAR10(root='./data', train=True,
download=True, transform=transform)
trainloader = torch.utils.data.DataLoader(trainset, batch_size=4,
shuffle=True, num_workers=2)
testset = datasets.CIFAR10(root='./data', train=False,
download=True, transform=transform)
testloader = torch.utils.data.DataLoader(testset, batch_size=4,
shuffle=False, num_workers=2)
```
但是由于你不想使用torchvision,我们需要手动加载数据集并进行预处理:
``` python
import pickle
def load_batch(filename):
with open(filename, 'rb') as f:
data = pickle.load(f, encoding='bytes')
images = data[b'data']
labels = data[b'labels']
images = images.reshape((len(images), 3, 32, 32))
images = images.transpose((0, 2, 3, 1))
labels = np.array(labels)
return images, labels
def load_data():
train_images = []
train_labels = []
for i in range(1, 6):
filename = f'./data_batch_{i}'
images, labels = load_batch(filename)
train_images.append(images)
train_labels.append(labels)
train_images = np.concatenate(train_images, axis=0)
train_labels = np.concatenate(train_labels, axis=0)
test_images, test_labels = load_batch('./test_batch')
return train_images, train_labels, test_images, test_labels
train_images, train_labels, test_images, test_labels = load_data()
train_images = train_images / 255.0
test_images = test_images / 255.0
train_images = torch.from_numpy(train_images).float()
train_labels = torch.from_numpy(train_labels).long()
test_images = torch.from_numpy(test_images).float()
test_labels = torch.from_numpy(test_labels).long()
trainset = torch.utils.data.TensorDataset(train_images, train_labels)
trainloader = torch.utils.data.DataLoader(trainset, batch_size=4,
shuffle=True, num_workers=2)
testset = torch.utils.data.TensorDataset(test_images, test_labels)
testloader = torch.utils.data.DataLoader(testset, batch_size=4,
shuffle=False, num_workers=2)
```
最后,我们可以开始训练模型了:
``` python
for epoch in range(10):
running_loss = 0.0
for i, data in enumerate(trainloader, 0):
inputs, labels = data
optimizer.zero_grad()
outputs = net(inputs)
loss = criterion(outputs, labels)
loss.backward()
optimizer.step()
running_loss += loss.item()
if i % 2000 == 1999:
print('[%d, %5d] loss: %.3f' %
(epoch + 1, i + 1, running_loss / 2000))
running_loss = 0.0
print('Finished Training')
```
在训练完成后,我们可以对模型进行测试并输出准确率:
``` python
correct = 0
total = 0
with torch.no_grad():
for data in testloader:
images, labels = data
outputs = net(images)
_, predicted = torch.max(outputs.data, 1)
total += labels.size(0)
correct += (predicted == labels).sum().item()
print('Accuracy of the network on the 10000 test images: %d %%' % (
100 * correct / total))
```
这就是一个不使用torchvision完成cifar10的卷积神经网络的代码。希望能对你有所帮助!
阅读全文
相关推荐
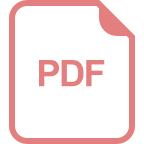
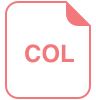
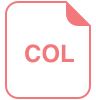
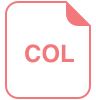
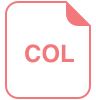










