解释代码: avg_train_loss = accumulate_train_loss / len(train) avg_test_loss = accumulate_test_loss / len(test) print("{} / {} train_loss: {:.6f}".format(epoch, epochs, avg_train_loss)) print("{} / {} test_loss : {:.6f}".format(epoch, epochs, avg_test_loss)) train_loss_list.append(avg_train_loss) test_loss_list.append(avg_test_loss) if avg_test_loss < best_loss: best_loss = avg_test_loss best_model_weights = copy.deepcopy(model.state_dict()) flag = True if flag == False and epoch > 100: # 100轮未得到best_loss连续3轮则结束训练 cnt_no_increasing += 1 if cnt_no_increasing > 3: break else: cnt_no_increasing = 0
时间: 2024-04-18 14:33:17 浏览: 14
这段代码用于计算并打印每个训练周期(epoch)的平均训练损失和平均测试损失,并将它们存储在相应的列表中。此外,它还根据测试损失的表现更新最佳模型的权重。
1. `avg_train_loss = accumulate_train_loss / len(train)`: 这行代码计算平均训练损失,通过将累积的训练损失值除以训练数据集的大小(`len(train)`)得到。
2. `avg_test_loss = accumulate_test_loss / len(test)`: 这行代码计算平均测试损失,通过将累积的测试损失值除以测试数据集的大小(`len(test)`)得到。
3. `print("{} / {} train_loss: {:.6f}".format(epoch, epochs, avg_train_loss))`: 这行代码打印当前训练周期、总周期数和平均训练损失。使用`format`方法将这些变量插入到打印字符串中,其中`{:.6f}`表示使用6位小数来显示训练损失值。
4. `print("{} / {} test_loss : {:.6f}".format(epoch, epochs, avg_test_loss))`: 这行代码打印当前训练周期、总周期数和平均测试损失。与上一行类似,使用`format`方法将变量插入到打印字符串中。
5. `train_loss_list.append(avg_train_loss)`: 将平均训练损失添加到训练损失列表`train_loss_list`中。
6. `test_loss_list.append(avg_test_loss)`: 将平均测试损失添加到测试损失列表`test_loss_list`中。
7. `if avg_test_loss < best_loss: ...`: 这个条件判断当前的平均测试损失是否比之前记录的最佳损失`best_loss`更低。如果是,则更新`best_loss`为当前平均测试损失,并使用`copy.deepcopy()`方法深度复制模型的权重`model.state_dict()`到`best_model_weights`中。
8. `if flag == False and epoch > 100: ...`: 这个条件判断是否需要终止训练。如果`flag`为False(表示在最近的一次迭代中没有更新最佳损失)且当前训练周期大于100,将计数器`cnt_no_increasing`加1。
9. `cnt_no_increasing = 0`: 将计数器`cnt_no_increasing`重置为0。
10. `break`: 如果连续3个训练周期都没有更新最佳损失,则跳出训练循环,结束训练过程。
通过以上代码,可以实时地跟踪训练和测试损失,并在达到一定条件时终止训练,以避免过拟合或提前停止。
相关推荐
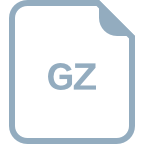
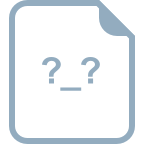














