matlab计算图片的均方根误差
时间: 2023-07-13 11:04:09 浏览: 68
MATLAB中计算图片的均方根误差可以使用`immse`函数。具体步骤如下:
1. 读取原始图片和处理后的图片,可以使用`imread`函数读取图片。
```matlab
img1 = imread('original.jpg');
img2 = imread('processed.jpg');
```
2. 将图片转换成双精度类型,并将像素值归一化到[0,1]范围内。
```matlab
img1 = im2double(img1);
img2 = im2double(img2);
```
3. 使用`immse`函数计算均方根误差。该函数的第一个参数为原始图片,第二个参数为处理后的图片。
```matlab
mse = immse(img1, img2);
rmse = sqrt(mse);
```
其中`mse`为均方误差,`rmse`为均方根误差。
完整代码如下:
```matlab
img1 = imread('original.jpg');
img2 = imread('processed.jpg');
img1 = im2double(img1);
img2 = im2double(img2);
mse = immse(img1, img2);
rmse = sqrt(mse);
```
相关问题
matlab计算均方根误差
在Matlab中计算均方根误差(RMSE),可以使用以下方法之一:
方法1:直接使用Matlab中的rmse函数
```matlab
% 假设ytest和ytest_fit是一维数组,分别表示测试集的真实值和预测值
test_error = ytest - ytest_fit;
test_rmse = rmse(test_error);
```
方法2:使用均方根误差(RMSE)公式手动计算
```matlab
% 假设ytest和ytest_fit是一维数组,分别表示测试集的真实值和预测值
test_error = ytest - ytest_fit;
test_rmse = sqrt(sum(test_error.^2) / length(test_error));
```
matlab交叉验证均方根误差
在MATLAB中,可以使用交叉验证来计算均方根误差(Root Mean Square Error, RMSE)。交叉验证是一种评估模型性能的方法,它通过将数据集分成训练集和验证集,然后使用训练集来训练模型,再使用验证集来评估模型的预测性能。
在交叉验证过程中,通常会使用均方根误差作为评估指标。均方根误差是预测值与实际值之间差异的平方的平均值的平方根。它可以用来衡量模型的预测精度,值越小表示模型的预测越准确。
具体计算均方根误差的步骤如下:
1. 将数据集分成K个折叠(K-Fold Cross Validation)。
2. 对于每个折叠,将其作为验证集,其余的折叠作为训练集。
3. 使用训练集来训练模型,并使用验证集来进行预测。
4. 计算每个验证集的预测值与实际值之间的差异,并计算均方根误差。
5. 对于所有的K个折叠,计算均方根误差的平均值。
通过交叉验证计算得到的均方根误差可以用来评估模型的性能,并选择最佳的模型参数或模型结构。
引用\[2\]和引用\[3\]提供了关于交叉验证的更详细的信息和示例。
#### 引用[.reference_title]
- *1* *2* *3* [执行交叉验证和验证](https://blog.csdn.net/weixin_39641738/article/details/115951280)[target="_blank" data-report-click={"spm":"1018.2226.3001.9630","extra":{"utm_source":"vip_chatgpt_common_search_pc_result","utm_medium":"distribute.pc_search_result.none-task-cask-2~all~insert_cask~default-1-null.142^v91^control_2,239^v3^insert_chatgpt"}} ] [.reference_item]
[ .reference_list ]
相关推荐
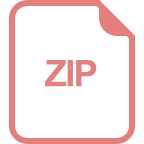
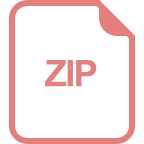












