deftSuffic = "Videos(*.avi *.mp4 *.wmv);;Films(*.mkv *.rmvb *.mpeg);;Alls(*.*)";
时间: 2023-11-14 18:54:42 浏览: 44
这是一个文件筛选器的字符串,用于在打开文件对话框中设置可选择的文件类型。其中,Videos(*.avi *.mp4 *.wmv)表示可以选择扩展名为.avi、.mp4和.wmv的视频文件,Films(*.mkv *.rmvb *.mpeg)表示可以选择扩展名为.mkv、.rmvb和.mpeg的电影文件,Alls(*.*)表示可以选择任何类型的文件。多个类型之间使用双分号“;;”隔开。
相关问题
vscode Component name "Films" should always be multi-word.eslint
根据引用和引用,在Unity 2018.2版本中,开始移除了旧版的MovieTexture功能,并引入了VideoPlayer来替代。所以,如果你的项目仍在使用MovieTexture,建议升级到使用VideoPlayer。然而,由于VideoPlayer与MovieTexture的方法不同,可能无法提供自动升级工具。关于MovieTexture的弃用可以在Unity的论坛中了解更多信息。
另外,根据引用,在面向.NET 4.x脚本运行时的项目中,现在支持C# 7.3版本。通过与开源Roslyn C#编译器的更好兼容性,引入了最新的C# 7.3语言特性,并减少了编译时间。
综上所述,根据提供的引用内容,"Films"这个组件名应该始终是多个单词,以符合eslint的要求。
def login_in(self): account = self.ui.lineEdit_L_account.text() password = self.ui.lineEdit_L_password.text() account_list = [] password_list = [] conn = pymysql.connect( user='root', password='796691xjl@', host='127.0.0.1', database='mysql', port=3306 ) cur = conn.cursor() cur.execute('SELECT * FROM films.manger') rows = cur.fetchall() for row in rows: account_list.append(row[0]) password_list.append(row[1]) print(account_list, password_list) conn.commit() conn.close() for i in range(len(account_list)): if len(account) == 0 or len(password) == 0: self.ui.stackedWidget.setCurrentIndex(1) elif account == account_list[i] and password == password_list[i]: global manger_now manger_now = account self.win = MainWindow() self.close() else: self.ui.stackedWidget.setCurrentIndex(2) print("wrong")无法执行elif语句
根据给出的代码段,我发现问题可能出在 elif 语句的缩进上。else 语句下的 print("wrong") 语句没有与前面的 elif 语句保持相同的缩进,导致该语句没有被包含在 elif 语句块中,因此无法执行 elif 语句。你可以将 print("wrong") 语句的缩进调整到与 elif 语句相同的缩进,例如:
```
if len(account) == 0 or len(password) == 0:
self.ui.stackedWidget.setCurrentIndex(1)
elif account == account_list[i] and password == password_list[i]:
global manger_now
manger_now = account
self.win = MainWindow()
self.close()
else:
self.ui.stackedWidget.setCurrentIndex(2)
print("wrong")
```
这样修改后,你再次执行代码时应该就能够执行 elif 语句了。
相关推荐
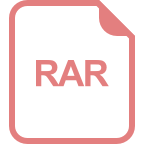
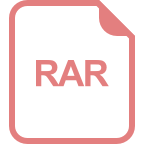
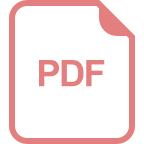





import requests import re # from bs4 import BeautifulSoup import matplotlib.pyplot as plt import numpy as np # import pandas as pd i = 1 lists = [0, 25, 50, 75, 100, 125, 150, 175, 200, 225, 250] title = [] year = [] country = [] score = [] number = [] for page in range(0, 226, 25): url = 'https://movie.douban.com/top250?start=' + str(page) + '&filter=' headers = { 'User-Agent': "Mozilla/5.0 (Windows NT 10.0; WOW64) AppleWebKit/537.36 (KHTML, like Gecko) Chrome/72.0.3626.121 Safari/537.36"} resp = requests.get(url=url, headers=headers) resp.encoding = "utf-8" pattern = re.compile( r'.*? < img width="100" alt="(?P<title>.*?)".*?class="">.*?.*?导演: (?P<director>.*?) .*?
.*?(?P<year>.*?) / (?P<country>.*?) .*?"v:average">(?P<score>.*?).*?(?P<number>.*?)人评价', re.S) pic_url = re.compile(r'< img width="100".*?src="(.*?)" class="">', re.S) pic_URl = pic_url.findall(resp.text) data2 = pattern.finditer(str(resp.text)) for url1 in pic_URl: file1 = open('films.pic\\' + str(i) + '.jpg', 'ab') Pic = requests.get(url1) file1.write(Pic.content) i = i + 1 file1.close() file2 = open('movie.text', 'a+', encoding='utf-8') for m in data2: if int(m['number']) / 100000 > 13: number.append(int(m['number']) / 100000) country.append(m['country']) year.append(m['year']) title.append(m['title']) score.append(m['score']) file2.write( '电影名:' + m['title'] + ', 导演:' + m['director'] + ', 年份:' + m['year'] + ', 国家:' + m['country'] + ', 评分:' + m[ 'score'] + ',评价人数:' + str(int(m['number']) / 100000) + ' 100k') file2.write('\n') print( '电影名:' + m['title'] + ', 导演:' + m['director'] + ', 年份:' + m['year'] + ', 国家:' + m['country'] + ', 评分:' + m[ 'score'] + ',评价人数:' + str(int(m['number']) / 100000) + ' 100k')








