def is_preprocess_batch(input,n): out = np.empty([n, 1, 400]) for i in range(n): y = is_preprocess(input[i,0,:]) out[i, 0] = y return out请用中文逐行分析这段代码
时间: 2024-02-14 21:29:25 浏览: 94
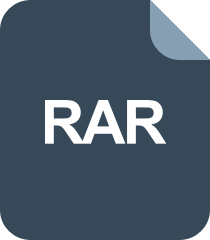
speex_preprocess.rar_Never Never
这段代码定义了一个函数 is_preprocess_batch,它接受两个参数:input 和 n。该函数的目的是对输入的数据进行批量预处理。
首先,代码创建了一个大小为 [n, 1, 400] 的空数组 out,用于存储预处理后的数据。这个数组将包含 n 个样本,每个样本都是一个大小为 1x400 的数组。
接下来,使用 for 循环遍历从 0 到 n-1 的索引 i。在每次循环中,代码取出输入数据 input 的第 i 行的第一个元素(input[i,0,:]),并调用函数 is_preprocess 对这个元素进行预处理。
预处理后的结果 y 被赋值给数组 out 的第 i 行的第一个元素(out[i, 0] = y)。
最后,函数返回数组 out,其中包含了经过预处理的所有样本数据。
阅读全文
相关推荐
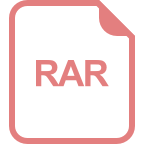
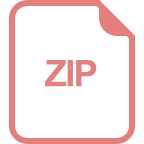
















