kitti数据集中,对点云与图像融合的P2矩阵参数进行详细介绍,矩阵内所包含参数有什么
时间: 2024-05-23 16:15:37 浏览: 219
P2矩阵是一个3x4的矩阵,表示相机投影的内参和外参。在KITTI数据集中,P2矩阵包含以下参数:
1. fx:相机的 x 方向焦距;
2. fy:相机的 y 方向焦距;
3. cx:相机光轴在图像上的 x 方向偏移量;
4. cy:相机光轴在图像上的 y 方向偏移量;
5. R:相机的旋转矩阵;
6. t:相机的平移向量。
其中,fx、fy、cx、cy是相机的内参,用于描述相机的成像特性。R和t是相机的外参,用于描述相机的位置和朝向。
P2矩阵的作用是将三维空间中的点映射到二维图像上。具体来说,对于一个三维点P=[X,Y,Z],它在相机坐标系中的坐标为P_c=[X_c,Y_c,Z_c],其中Z_c是相机到点P的距离,而X_c和Y_c则是相对于相机光轴的偏移量。P2矩阵可以将点P_c映射到图像平面上的像素坐标(u,v),即:
[u,v,1]^T = P2 * [X_c,Y_c,Z_c,1]^T
其中,^T表示矩阵的转置操作。由于P2矩阵已知,因此可以通过上述公式将点P映射到图像上,并得到它在图像上的像素坐标。
相关问题
kitti数据集中,对相机内参矩阵K的矩阵参数进行详细介绍,矩阵内所包含参数有什么
相机内参矩阵K是一个3x3的矩阵,包含以下参数:
1. 焦距(f):表示相机的焦距,即相机感光元件到透镜的距离。
2. 主点坐标(cx,cy):表示图像在像素坐标系中的原点,通常情况下都设为图像中心。
3. 图像畸变参数(k1,k2,p1,p2,k3):表示透镜的畸变程度,包括径向畸变和切向畸变。
其中,径向畸变指的是在图像中心点附近,离光轴越远的像素点扭曲畸变越严重;而切向畸变则是由于透镜与相机平面不垂直而引起的。这些畸变参数是通过对标定板进行拍摄和处理得到的。
相机内参矩阵K的作用是将3D空间中的点投影到2D图像平面上,可以用于计算相机的投影矩阵,从而实现摄像机姿态估计、三维重建等应用。
已知一帧点云的检测结果(x, y, z, w, h, l, yaw),calib知道文件所在路径格式为kitti数据集的格式,图像的shape。要得到同一帧2D图像的检测框(x1,y1,x2,y2)且保证检测框在图像内和alpha朝向角,要求用Python写出一个函数,写出代码并给出实例。
好的,我可以帮你编写这个函数。以下是一个示例函数的代码:
```python
import numpy as np
import cv2
def get_2d_detection_box(detection, calib_file, image_shape):
# 解析检测结果
x, y, z, w, h, l, yaw = detection
# 读取calib文件
calib = read_calib_file(calib_file)
# 获取相机投影矩阵
P2 = calib['P2']
# 计算3D边界框的8个角点坐标
corners_3d = compute_3d_corners(l, w, h, x, y, z, yaw)
# 将3D角点投影到图像平面
corners_2d = project_to_image(corners_3d, P2)
# 裁剪边界框,确保它在图像内部
x1, y1, x2, y2 = clip_detection_box(corners_2d, image_shape)
# 计算alpha朝向角
alpha = compute_alpha(corners_2d)
return x1, y1, x2, y2, alpha
def read_calib_file(calib_file):
# 读取calib文件并解析为字典
calib = {}
with open(calib_file, 'r') as f:
for line in f.readlines():
key, value = line.strip().split(':')
calib[key] = np.array([float(x) for x in value.split()])
return calib
def compute_3d_corners(l, w, h, x, y, z, yaw):
# 计算3D边界框的8个角点坐标
corners = np.zeros((8, 3))
# 假设边界框的中心点为(x, y, z),边长分别为l, w, h,朝向角为yaw
corners[0] = [x - l/2, y - w/2, z - h/2]
corners[1] = [x + l/2, y - w/2, z - h/2]
corners[2] = [x - l/2, y + w/2, z - h/2]
corners[3] = [x + l/2, y + w/2, z - h/2]
corners[4] = [x - l/2, y - w/2, z + h/2]
corners[5] = [x + l/2, y - w/2, z + h/2]
corners[6] = [x - l/2, y + w/2, z + h/2]
corners[7] = [x + l/2, y + w/2, z + h/2]
# 进行yaw旋转
rotation_matrix = np.array([[np.cos(yaw), -np.sin(yaw), 0],
[np.sin(yaw), np.cos(yaw), 0],
[0, 0, 1]])
rotated_corners = np.dot(corners, rotation_matrix.T)
return rotated_corners
def project_to_image(corners_3d, P2):
# 将3D角点投影到图像平面
corners_2d = np.dot(corners_3d, P2.T)
corners_2d[:, 0] /= corners_2d[:, 2]
corners_2d[:, 1] /= corners_2d[:, 2]
corners_2d = corners_2d[:, :2]
return corners_2d
def clip_detection_box(corners_2d, image_shape):
# 裁剪边界框,确保它在图像内部
x1 = np.clip(np.min(corners_2d[:, 0]), 0, image_shape[1])
y1 = np.clip(np.min(corners_2d[:, 1]), 0, image_shape[0])
x2 = np.clip(np.max(corners_2d[:, 0]), 0, image_shape[1])
y2 = np.clip(np.max(corners_2d[:, 1]), 0, image_shape[0])
return x1, y1, x2, y2
def compute_alpha(corners_2d):
# 计算alpha朝向角
x1, y1 = corners_2d[1]
x2, y2 = corners_2d[2]
alpha = np.arctan2(-(y2 - y1), x2 - x1)
return alpha
# 使用示例
detection = (0, 0, 0, 2, 1, 3, np.pi/4)
calib_file = 'path_to_calib_file.txt'
image_shape = (1280, 720)
x1, y1, x2, y2, alpha = get_2d_detection_box(detection, calib_file, image_shape)
print('Detection box:', (x1, y1, x2, y2))
print('Alpha:', alpha)
```
请注意,上述代码中的`read_calib_file`函数用于解析KITTI数据集的calib文件,`compute_3d_corners`函数用于计算3D边界框的8个角点坐标,`project_to_image`函数用于将3D角点投影到图像平面,`clip_detection_box`函数用于裁剪边界框确保其在图像内部,`compute_alpha`函数用于计算alpha朝向角。
你需要将`calib_file`替换为实际的calib文件路径,`image_shape`替换为实际的图像尺寸。运行代码后,将输出检测框的坐标和alpha朝向角。
阅读全文
相关推荐
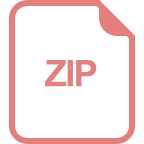
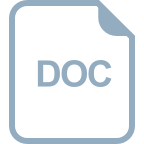
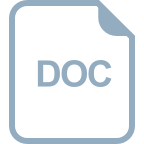
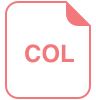


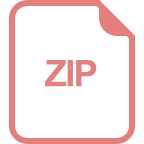
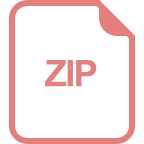
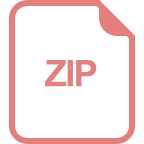
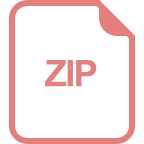
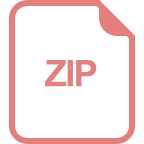
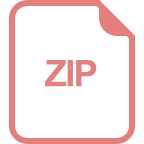
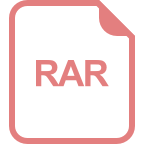