ResNet中BN的作用
时间: 2024-04-22 20:28:08 浏览: 25
BN(Batch Normalization)在ResNet中的作用是对每个批次的输入进行归一化处理,以加速网络的训练过程并提高网络的泛化能力。
具体来说,BN的作用有以下几个方面:
1. 加速收敛:通过对每个批次的输入进行归一化,可以使得网络更容易收敛。因为归一化可以将输入数据的分布调整到一个较小的范围内,避免了梯度爆炸或梯度消失的问题,从而加速了网络的收敛速度。
2. 抑制过拟合:BN在训练过程中引入了一些随机性,有助于抑制网络的过拟合现象。这是因为在每个批次中,BN会对输入进行归一化,并且会对归一化后的数据进行缩放和平移操作,从而引入一些噪声,增加了模型的泛化能力。
3. 改善梯度传播:在深层网络中,梯度传播往往会受到较大的影响,导致训练过程变得困难。通过BN,可以使得每一层的输入都具有相似的分布,从而减小了梯度传播的问题,使得网络更易于训练。
4. 具有一定的正则化效果:通过归一化输入,BN可以减少参数之间的相互依赖性,从而起到一定的正则化效果,有助于控制网络的复杂度。
综上所述,BN在ResNet中发挥了重要的作用,既可以加速网络的训练过程,又可以提高网络的泛化能力并抑制过拟合现象。
相关问题
resnet50中的BN层的用途
BN层是ResNet50中的一种正则化方法,用于加速神经网络的训练过程,防止梯度消失和梯度爆炸问题。它通过对每个batch的数据进行归一化,使得每个特征的均值和方差都接近于和1,从而提高网络的稳定性和泛化能力。
在resnet中加入Coordinate Attention模块代码
好的,下面是在ResNet中加入Coordinate Attention模块的代码。
首先,我们需要导入必要的库:
```python
import torch
import torch.nn as nn
import torch.nn.functional as F
```
然后,我们定义Coordinate Attention模块:
```python
class CoordAtt(nn.Module):
def __init__(self, in_channels):
super(CoordAtt, self).__init__()
self.in_channels = in_channels
self.gamma = nn.Parameter(torch.zeros(1))
self.sigma = nn.Parameter(torch.zeros(1))
self.coord_conv = nn.Conv2d(in_channels, in_channels, kernel_size=1)
self.bn = nn.BatchNorm2d(in_channels)
def forward(self, x):
batch, _, height, width = x.size()
xx = torch.arange(width).repeat(height, 1).float().to(x.device) / (width - 1)
yy = torch.arange(height).repeat(width, 1).t().float().to(x.device) / (height - 1)
xx = xx.view(1, 1, height, width).repeat(batch, 1, 1, 1)
yy = yy.view(1, 1, height, width).repeat(batch, 1, 1, 1)
coord_feat = torch.cat([xx, yy], 1)
coord_feat = self.coord_conv(coord_feat)
coord_feat = self.bn(coord_feat)
coord_feat = torch.sigmoid(coord_feat)
x_pool = x.view(batch, self.in_channels, -1).mean(dim=2).view(batch, self.in_channels, 1, 1)
gamma = self.gamma.view(1, -1, 1, 1)
sigma = self.sigma.view(1, -1, 1, 1)
y = torch.matmul(x_pool, coord_feat.view(batch, self.in_channels, -1))
y = y.view(batch, self.in_channels, 1, 1)
z = torch.matmul(coord_feat.view(batch, self.in_channels, -1), coord_feat.view(batch, self.in_channels, -1).transpose(1, 2))
attn = torch.softmax(torch.div(torch.matmul(y, z), sigma), dim=-1)
y = torch.matmul(attn, x.view(batch, self.in_channels, -1))
y = y.view(batch, self.in_channels, 1, 1)
out = gamma * y + x
return out
```
最后,我们把Coordinate Attention模块加入到ResNet中:
```python
class ResNet(nn.Module):
def __init__(self, block, layers, num_classes=1000, zero_init_residual=False):
super(ResNet, self).__init__()
self.in_channels = 64
self.conv1 = nn.Conv2d(3, 64, kernel_size=7, stride=2, padding=3, bias=False)
self.bn1 = nn.BatchNorm2d(64)
self.relu = nn.ReLU(inplace=True)
self.maxpool = nn.MaxPool2d(kernel_size=3, stride=2, padding=1)
self.layer1 = self._make_layer(block, 64, layers[0])
self.coord_att1 = CoordAtt(64) # 添加Coordinate Attention模块
self.layer2 = self._make_layer(block, 128, layers[1], stride=2)
self.coord_att2 = CoordAtt(128) # 添加Coordinate Attention模块
self.layer3 = self._make_layer(block, 256, layers[2], stride=2)
self.coord_att3 = CoordAtt(256) # 添加Coordinate Attention模块
self.layer4 = self._make_layer(block, 512, layers[3], stride=2)
self.coord_att4 = CoordAtt(512) # 添加Coordinate Attention模块
self.avgpool = nn.AdaptiveAvgPool2d((1, 1))
self.fc = nn.Linear(512 * block.expansion, num_classes)
for m in self.modules():
if isinstance(m, nn.Conv2d):
nn.init.kaiming_normal_(m.weight, mode='fan_out', nonlinearity='relu')
elif isinstance(m, (nn.BatchNorm2d, nn.GroupNorm)):
nn.init.constant_(m.weight, 1)
nn.init.constant_(m.bias, 0)
if zero_init_residual:
for m in self.modules():
if isinstance(m, Bottleneck):
nn.init.constant_(m.bn3.weight, 0)
elif isinstance(m, BasicBlock):
nn.init.constant_(m.bn2.weight, 0)
def _make_layer(self, block, out_channels, blocks, stride=1):
downsample = None
if stride != 1 or self.in_channels != out_channels * block.expansion:
downsample = nn.Sequential(
nn.Conv2d(self.in_channels, out_channels * block.expansion, kernel_size=1, stride=stride, bias=False),
nn.BatchNorm2d(out_channels * block.expansion),
)
layers = []
layers.append(block(self.in_channels, out_channels, stride, downsample))
self.in_channels = out_channels * block.expansion
for _ in range(1, blocks):
layers.append(block(self.in_channels, out_channels))
return nn.Sequential(*layers)
def forward(self, x):
x = self.conv1(x)
x = self.bn1(x)
x = self.relu(x)
x = self.maxpool(x)
x = self.layer1(x)
x = self.coord_att1(x) # Coordinate Attention模块
x = self.layer2(x)
x = self.coord_att2(x) # Coordinate Attention模块
x = self.layer3(x)
x = self.coord_att3(x) # Coordinate Attention模块
x = self.layer4(x)
x = self.coord_att4(x) # Coordinate Attention模块
x = self.avgpool(x)
x = x.view(x.size(0), -1)
x = self.fc(x)
return x
```
这样,我们就在ResNet中成功加入了Coordinate Attention模块。
相关推荐
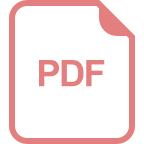
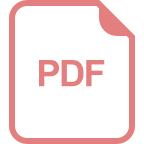
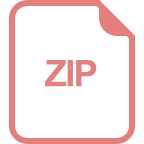












