解释代码: def detect_cma(self): # pass model = self.model output_size = self.output_size # source = self.img2predict # file/dir/URL/glob, 0 for webcam imgsz = [640, 640] # inference size (pixels) conf_thres = 0.25 # confidence threshold iou_thres = 0.45 # NMS IOU threshold max_det = 1000 # maximum detections per image # device = self.device # cuda device, i.e. 0 or 0,1,2,3 or cpu view_img = False # show results save_txt = False # save results to *.txt save_conf = False # save confidences in --save-txt labels save_crop = False # save cropped prediction boxes nosave = False # do not save images/videos classes = None # filter by class: --class 0, or --class 0 2 3 agnostic_nms = False # class-agnostic NMS augment = False # ugmented inference visualize = False # visualize features line_thickness = 3 # bounding box thickness (pixels) hide_labels = False # hide labels hide_conf = False # hide confidences half = False # use FP16 half-precision inference dnn = False # use OpenCV DNN for ONNX inference source = str(self.vid_source) device = select_device(self.device) stride, names, pt, jit, onnx = model.stride, model.names, model.pt, model.jit, model.onnx imgsz = check_img_size(imgsz, s=stride) # check image size save_img = not nosave and not source.endswith('.txt') # save inference images
时间: 2023-06-15 17:07:44 浏览: 429
这段代码用于使用 YOLOv5 模型对图像或视频进行目标检测。它首先从类的属性中获取模型、输出大小、视频源等信息。然后设置了一些参数,如推理尺寸、置信度阈值、NMS IOU 阈值等。接着根据设备类型选择使用 CPU 还是 GPU 进行推理。最后,它检查图像的大小是否符合模型要求,设置是否保存推理结果图片,然后返回检测结果。
相关问题
import cv2 import numpy as np from PyQt5 import QtWidgets from paddleocr import PaddleOCR from yolov8 import YOLOv8 # 假设你有YOLOv8的实现 # 定义车牌定位类 class PlateLocator: def __init__(self, model_path): self.model = YOLOv8(model_path) def locate_and_crop(self, image): boxes = self.model.detect(image) plates = [] for box in boxes: x, y, w, h = box plate = image[y:y+h, x:x+w] plates.append(plate) return plates # 定义车牌识别类 class PlateRecognizer: def __init__(self): self.model = PaddleOCR() def recognize(self, plate): result = self.model.ocr(plate, use_gpu=False) return result # 定义GUI类 class App(QtWidgets.QMainWindow): def __init__(self, locator, recognizer): super().__init__() self.locator = locator self.recognizer = recognizer self.initUI() def initUI(self): # 创建识别按钮 btn = QtWidgets.QPushButton('识别', self) btn.clicked.connect(self.detect_plate) # 其他UI代码... def detect_plate(self): # 从视频流中捕获一帧图像 ret, frame = self.cap.read() # 定位和裁剪车牌 plates = self.locator.locate_and_crop(frame) for plate in plates: # 对车牌进行识别 result = self.recognizer.recognize(plate) print(result) # 展示或保存结果... if __name__ == "__main__": locator = PlateLocator('yolov8_model_path.pth') recognizer = PlateRecognizer() app = QtWidgets.QApplication([]) ex = App(locator, recognizer) ex.show() app.exec_()帮我改进一下代码
好的,我来帮你改进一下代码。
1. 首先,建议将代码进行模块化,分别定义车牌定位和车牌识别两个类,这样可以使代码更加清晰易懂。
2. 在GUI类中,建议将视频流采集和识别操作分离开来,这样可以将GUI类和定位、识别类解耦,使代码更加灵活。
3. 对于定位和识别的结果,可以考虑以图像形式展示或保存,而不是只打印出来。
下面是修改后的代码示例:
```
import cv2
import numpy as np
from PyQt5 import QtWidgets
from paddleocr import PaddleOCR
from yolov8 import YOLOv8
# 定义车牌定位类
class PlateLocator:
def __init__(self, model_path):
self.model = YOLOv8(model_path)
def locate_and_crop(self, image):
boxes = self.model.detect(image)
plates = []
for box in boxes:
x, y, w, h = box
plate = image[y:y+h, x:x+w]
plates.append(plate)
return plates
# 定义车牌识别类
class PlateRecognizer:
def __init__(self):
self.model = PaddleOCR()
def recognize(self, plate):
result = self.model.ocr(plate, use_gpu=False)
return result
# 定义GUI类
class App(QtWidgets.QMainWindow):
def __init__(self):
super().__init__()
self.initUI()
def initUI(self):
# 创建采集按钮和识别按钮
self.btn_collect = QtWidgets.QPushButton('采集', self)
self.btn_collect.clicked.connect(self.collect_video)
self.btn_recognize = QtWidgets.QPushButton('识别', self)
self.btn_recognize.clicked.connect(self.recognize_plate)
# 其他UI代码...
def collect_video(self):
# 在此处添加视频流采集代码
pass
def recognize_plate(self):
# 在此处添加车牌定位和识别代码
pass
def show_image(self, image):
# 在此处添加展示或保存图像的代码
pass
if __name__ == "__main__":
app = QtWidgets.QApplication([])
ex = App()
ex.show()
app.exec_()
```
注意:在上述代码中,我将定位和识别类的初始化放到了GUI类外部进行,这样可以避免每次点击按钮都要重新初始化模型的问题。如果你的模型比较大,初始化时间比较长,可以考虑使用多线程或异步编程方式来加速模型初始化。
class TracedModel(nn.Module): def __init__(self, model=None, device=None, img_size=(640,640)): super(TracedModel, self).__init__() print(" Convert model to Traced-model... ") self.stride = model.stride self.names = model.names self.model = model self.model = revert_sync_batchnorm(self.model) self.model.to('cpu') self.model.eval() self.detect_layer = self.model.model[-1] self.model.traced = True rand_example = torch.rand(1, 3, img_size, img_size) traced_script_module = torch.jit.trace(self.model, rand_example, strict=False) #traced_script_module = torch.jit.script(self.model) traced_script_module.save("traced_model.pt") print(" traced_script_module saved! ") self.model = traced_script_module self.model.to(device) self.detect_layer.to(device) print(" model is traced! \n") def forward(self, x, augment=False, profile=False): out = self.model(x) out = self.detect_layer(out) return out是什么意思
这是一个 PyTorch 模型类,名为 TracedModel。它的构造函数中接受一个模型对象和一些参数,将该模型转换为 TorchScript 格式的模型,并保存为文件 "traced_model.pt"。该模型类还定义了一个前向传播函数 forward(),接受输入数据 x,通过模型和检测层进行预测,最终输出预测结果 out。如果参数 augment 为 True,则会进行数据增强;如果参数 profile 为 True,则会记录函数的计算时间和内存使用情况。
阅读全文
相关推荐
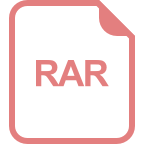
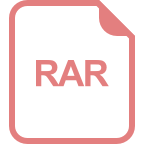
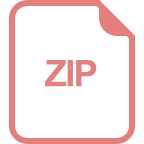









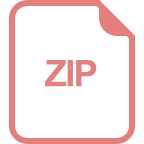
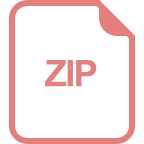
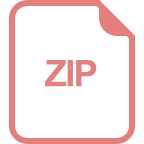
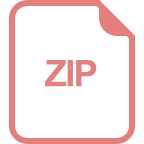
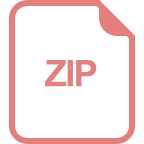