audio_path = 'C:\\Users\\75230\\Desktop\\audio_recording_1666952148489.wav' 代码是否存在问题
时间: 2023-08-27 22:20:15 浏览: 53
根据你提供的代码,音频文件路径的写法是正确的。使用双反斜杠"\\ "来表示文件路径中的单个反斜杠"\ "是Windows系统中的常见写法。因此,你的代码中的路径格式是正确的。
然而,为了确保代码的正确性,你仍然需要确保提供的音频文件路径是正确的,并且可以在你的计算机上找到该文件。另外,还需要确保Librosa库已经正确安装。
如果你仍然遇到问题,请提供更详细的错误信息,以便我们能够更好地帮助你。
相关问题
文件地址为:C:\\Users\\75230\\Desktop\\audio_recording_1666952148489.wav 请给出提取该文件语音特征的Python代码
要提取音频文件的语音特征,你可以使用`librosa`库来完成。下面是一个示例代码,可以提取音频文件的MFCC特征:
```python
import librosa
# 音频文件路径
audio_path = 'C:\\Users\\75230\\Desktop\\audio_recording_1666952148489.wav'
# 加载音频文件
audio, sr = librosa.load(audio_path)
# 提取MFCC特征
mfcc = librosa.feature.mfcc(audio, sr=sr)
# 打印MFCC特征矩阵的形状
print("MFCC 特征矩阵的形状:", mfcc.shape)
```
在上面的代码中,我们使用`librosa.load`函数来加载音频文件,并返回音频数据和采样率。然后,我们使用`librosa.feature.mfcc`函数来提取音频的MFCC特征。最后,我们打印出MFCC特征矩阵的形状。
请注意,此代码需要确保`librosa`库已经正确安装。如果你尚未安装该库,可以使用以下命令进行安装:
```
pip install librosa
```
这样就可以在你的Python环境中使用`librosa`库来提取音频特征了。
class HotwordDetector(object): """ Snowboy decoder to detect whether a keyword specified by `decoder_model` exists in a microphone input stream. :param decoder_model: decoder model file path, a string or a list of strings :param resource: resource file path. :param sensitivity: decoder sensitivity, a float of a list of floats. The bigger the value, the more senstive the decoder. If an empty list is provided, then the default sensitivity in the model will be used. :param audio_gain: multiply input volume by this factor. :param apply_frontend: applies the frontend processing algorithm if True. """ def __init__(self, decoder_model, resource=RESOURCE_FILE, sensitivity=[], audio_gain=1, apply_frontend=False): tm = type(decoder_model) ts = type(sensitivity) if tm is not list: decoder_model = [decoder_model] if ts is not list: sensitivity = [sensitivity] model_str = ",".join(decoder_model) self.detector = snowboydetect.SnowboyDetect( resource_filename=resource.encode(), model_str=model_str.encode()) self.detector.SetAudioGain(audio_gain) self.detector.ApplyFrontend(apply_frontend) self.num_hotwords = self.detector.NumHotwords() if len(decoder_model) > 1 and len(sensitivity) == 1: sensitivity = sensitivity * self.num_hotwords if len(sensitivity) != 0: assert self.num_hotwords == len(sensitivity), \ "number of hotwords in decoder_model (%d) and sensitivity " \ "(%d) does not match" % (self.num_hotwords, len(sensitivity)) sensitivity_str = ",".join([str(t) for t in sensitivity]) if len(sensitivity) != 0: self.detector.SetSensitivity(sensitivity_str.encode()) self.ring_buffer = RingBuffer( self.detector.NumChannels() * self.detector.SampleRate() * 5) def start(self, detected_callback=play_audio_file, interrupt_check=lambda: False, sleep_time=0.03, audio_recorder_callback=None, silent_count_threshold=15, recording_timeout=100):
这段代码看起来像是一个热词检测器类的初始化方法。参数包括 `decoder_model`,一个解码器模型文件的路径;`resource`,资源文件的路径;`sensitivity`,一个或多个检测器灵敏度的浮点数;`audio_gain`,音频增益的倍数;`apply_frontend`,是否应用前端处理算法。这个类的实例化会生成一个 SnowboyDetect 对象,用于检测语音流中是否存在指定的关键词。类还包括了一些方法,用于开始检测、回调处理、中断检查等。
相关推荐
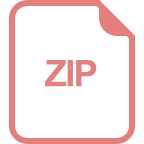
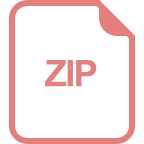













