..\AAA\waveform.c(7): error: #147: declaration is incompatible with "unsigned int sin_data" (declared at line 8 of "..\AAA\waveform.h")
时间: 2023-10-07 21:14:21 浏览: 115
这个错误提示表示在 waveform.c 的第 7 行有一个声明与 waveform.h 中的 unsigned int sin_data 不兼容。具体原因可能是两个地方对同一个变量的声明不一致。
要解决这个问题,你可以按照以下步骤进行排查和修复:
1. 打开 waveform.c 文件,并定位到第 7 行,查看这个地方对 sin_data 变量的声明。
2. 打开 waveform.h 文件,并定位到第 8 行,查看对 sin_data 变量的声明。
3. 对比这两个地方对 sin_data 变量声明的方式和类型,确认是否有不一致之处。
4. 如果发现不一致,需要修正其中一个地方的声明,使其与另一个地方保持一致。可能需要修改数据类型、添加关键字或者调整声明方式等。
5. 保存修改后的文件,并重新编译你的代码,看是否解决了这个错误。
请注意,确保在 waveform.c 和 waveform.h 两个文件中对 sin_data 变量的声明完全一致是很重要的,这样才能避免编译错误。
希望这些步骤能够帮助你解决问题。如果还有其他疑问,请随时提问。
相关问题
详细解释以下Python代码:import numpy as np import adi import matplotlib.pyplot as plt sample_rate = 1e6 # Hz center_freq = 915e6 # Hz num_samps = 100000 # number of samples per call to rx() sdr = adi.Pluto("ip:192.168.2.1") sdr.sample_rate = int(sample_rate) # Config Tx sdr.tx_rf_bandwidth = int(sample_rate) # filter cutoff, just set it to the same as sample rate sdr.tx_lo = int(center_freq) sdr.tx_hardwaregain_chan0 = -50 # Increase to increase tx power, valid range is -90 to 0 dB # Config Rx sdr.rx_lo = int(center_freq) sdr.rx_rf_bandwidth = int(sample_rate) sdr.rx_buffer_size = num_samps sdr.gain_control_mode_chan0 = 'manual' sdr.rx_hardwaregain_chan0 = 0.0 # dB, increase to increase the receive gain, but be careful not to saturate the ADC # Create transmit waveform (QPSK, 16 samples per symbol) num_symbols = 1000 x_int = np.random.randint(0, 4, num_symbols) # 0 to 3 x_degrees = x_int*360/4.0 + 45 # 45, 135, 225, 315 degrees x_radians = x_degrees*np.pi/180.0 # sin() and cos() takes in radians x_symbols = np.cos(x_radians) + 1j*np.sin(x_radians) # this produces our QPSK complex symbols samples = np.repeat(x_symbols, 16) # 16 samples per symbol (rectangular pulses) samples *= 2**14 # The PlutoSDR expects samples to be between -2^14 and +2^14, not -1 and +1 like some SDRs # Start the transmitter sdr.tx_cyclic_buffer = True # Enable cyclic buffers sdr.tx(samples) # start transmitting # Clear buffer just to be safe for i in range (0, 10): raw_data = sdr.rx() # Receive samples rx_samples = sdr.rx() print(rx_samples) # Stop transmitting sdr.tx_destroy_buffer() # Calculate power spectral density (frequency domain version of signal) psd = np.abs(np.fft.fftshift(np.fft.fft(rx_samples)))**2 psd_dB = 10*np.log10(psd) f = np.linspace(sample_rate/-2, sample_rate/2, len(psd)) # Plot time domain plt.figure(0) plt.plot(np.real(rx_samples[::100])) plt.plot(np.imag(rx_samples[::100])) plt.xlabel("Time") # Plot freq domain plt.figure(1) plt.plot(f/1e6, psd_dB) plt.xlabel("Frequency [MHz]") plt.ylabel("PSD") plt.show(),并分析该代码中QPSK信号的功率谱密度图的特点
这段Python代码的作用是使用ADI Pluto SDR设备生成并传输一个QPSK信号,并将接收到的信号进行功率谱密度分析。下面是对代码的注释:
```
import numpy as np
import adi
import matplotlib.pyplot as plt
# 设置采样率、中心频率和采样点数
sample_rate = 1e6 # Hz
center_freq = 915e6 # Hz
num_samps = 100000 # number of samples per call to rx()
# 连接ADI Pluto SDR设备
sdr = adi.Pluto("ip:192.168.2.1")
sdr.sample_rate = int(sample_rate)
# 配置发送端的参数
sdr.tx_rf_bandwidth = int(sample_rate) # filter cutoff, just set it to the same as sample rate
sdr.tx_lo = int(center_freq)
sdr.tx_hardwaregain_chan0 = -50 # Increase to increase tx power, valid range is -90 to 0 dB
# 配置接收端的参数
sdr.rx_lo = int(center_freq)
sdr.rx_rf_bandwidth = int(sample_rate)
sdr.rx_buffer_size = num_samps
sdr.gain_control_mode_chan0 = 'manual'
sdr.rx_hardwaregain_chan0 = 0.0 # dB, increase to increase the receive gain, but be careful not to saturate the ADC
# 创建发送的QPSK信号
num_symbols = 1000
x_int = np.random.randint(0, 4, num_symbols) # 0 to 3
x_degrees = x_int*360/4.0 + 45 # 45, 135, 225, 315 degrees
x_radians = x_degrees*np.pi/180.0 # sin() and cos() takes in radians
x_symbols = np.cos(x_radians) + 1j*np.sin(x_radians) # this produces our QPSK complex symbols
samples = np.repeat(x_symbols, 16) # 16 samples per symbol (rectangular pulses)
samples *= 2**14 # The PlutoSDR expects samples to be between -2^14 and +2^14, not -1 and +1 like some SDRs
# 启动发送端并发送信号
sdr.tx_cyclic_buffer = True # Enable cyclic buffers
sdr.tx(samples) # start transmitting
# 接收接收端的信号
for i in range (0, 10):
raw_data = sdr.rx() # Receive samples
rx_samples = sdr.rx()
print(rx_samples)
# 停止发送端
sdr.tx_destroy_buffer()
# 计算接收到的信号的功率谱密度
psd = np.abs(np.fft.fftshift(np.fft.fft(rx_samples)))**2
psd_dB = 10*np.log10(psd)
f = np.linspace(sample_rate/-2, sample_rate/2, len(psd))
# 绘制时域图
plt.figure(0)
plt.plot(np.real(rx_samples[::100]))
plt.plot(np.imag(rx_samples[::100]))
plt.xlabel("Time")
# 绘制频域图
plt.figure(1)
plt.plot(f/1e6, psd_dB)
plt.xlabel("Frequency [MHz]")
plt.ylabel("PSD")
plt.show()
```
以上代码生成了一个随机QPSK信号,通过ADI Pluto SDR设备将其传输,并使用Pluto SDR设备接收该信号。接收到的信号进行了功率谱密度分析,并绘制了频域图。
QPSK信号的功率谱密度图的特点是,其频谱表现为四个簇,每个簇对应QPSK信号的一个符号。每个簇的带宽约为基带信号的带宽,且由于使用矩形脉冲,每个簇的带宽之间有一定的重叠。此外,功率谱密度图中还可以看到一些其他频率分量,这些分量可能是由于接收信号中存在其他干扰或噪声导致的。
D:\zhaoxiaobai\conda\envs\audio\python.exe "D:/audio_deep learning/dataset/class dataset.py" Traceback (most recent call last): File "D:/audio_deep learning/dataset/class dataset.py", line 10, in <module> waveform,sample_rate = torchaudio.load(filepath) File "D:\zhaoxiaobai\conda\envs\audio\lib\site-packages\torchaudio\backend\soundfile_backend.py", line 221, in load with soundfile.SoundFile(filepath, "r") as file_: File "D:\zhaoxiaobai\conda\envs\audio\lib\site-packages\soundfile.py", line 658, in __init__ self._file = self._open(file, mode_int, closefd) File "D:\zhaoxiaobai\conda\envs\audio\lib\site-packages\soundfile.py", line 1216, in _open raise LibsndfileError(err, prefix="Error opening {0!r}: ".format(self.name)) soundfile.LibsndfileError: Error opening 'D:\\audio_deep learning\\hole.wav': Format not recognised.
根据错误提示,您的音频文件 'D:\\audio_deep learning\\hole.wav' 的格式无法被识别。可以尝试以下方法解决问题:
1. 确认音频文件格式:使用其他工具(例如Audacity)打开音频文件,确认它的格式是否为torchaudio支持的格式(如wav、mp3、flac、ogg等)。
2. 安装依赖库:您需要安装所需的依赖库,例如libsndfile(libavformat/libavcodec)等,以支持更多的音频格式。具体方法可以参考torchaudio官方文档。
3. 尝试转换音频格式:如果您确认音频文件格式正确,但仍无法加载,请尝试使用其他工具将音频文件转换为torchaudio支持的格式,例如使用ffmpeg等。
如果以上方法仍无法解决问题,请提供更多信息和错误提示,以便更好地帮助您解决问题。
阅读全文
相关推荐
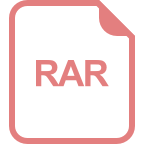
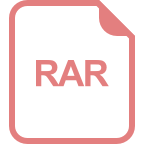
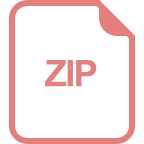
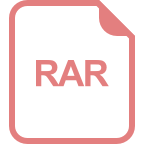
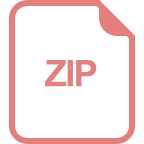
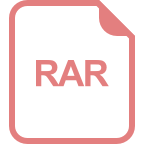
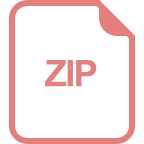
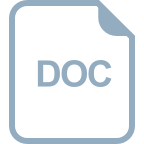







